Validated LSTM Neural Network for Triggered Goals of Care Discussions in Seriously Ill Hospitalized Patients. (GP152)
Journal of Pain and Symptom Management(2024)
摘要
Outcomes
1. Utilize predictive models to enhance hospice and palliative care services.2. Understand the interpretations and implications of predictive models' performance parameters.
Key Message
Several predictive models for mortality were developed during the COVID-19 pandemic. However, models become obsolete with clinical advancements. Hence, new predictive models for mortality that consider recent clinical advancements are needed for effective and efficient early-life-saving interventions, hospital resource triaging, and palliative care services.
Importance
This study develops a model to identify high-risk patients for mortality, enabling effective and efficient early-life-saving interventions, optimizing triaging of hospital resources, and enhancing palliative care services.
Objective(s)
Our study aims to develop a new model that accounts for recent clinical advancements, such as the introduction of COVID-19 vaccination, to predict in-hospital mortality, mortality within 30 days after discharge from hospitalization, or discharge from hospitalization into hospice care.
Scientific Methods Utilized
We conducted a retrospective cohort study by using data from BJC Integrated Health Systems between March 2021 and October 2022 to develop a Long Short-Term Memory (LSTM) neural network model among hospitalized patients. The model predicts a composite outcome of in-hospital mortality, mortality within 30 days after discharge, or discharge into hospice care.
Results
The total sample size used for model development was 94,017, with 75,213 for training data, 9,402 for validation, and 9,402 for test data. Our model achieved an area under the curve (AUC) of 90% and demonstrated high specificity at a threshold of 0.5 (specificity of 99% and sensitivity of 30%). Furthermore, the model exhibited good sensitivity at a threshold of 0.1 (specificity of 86% and sensitivity of 78%). Across different population strata such as race (White and Non-white), COVID-19 status (positive and negative), and Intensive Care Unit (ICU) admissions (positive and negative), the model consistently performed well, with AUC values at or above 85% and sensitivity and specificity mostly exceeding 80% at a threshold of 0.1.
Conclusion(s)
Our developed model effectively identified patients at a high risk of in-hospital mortality, mortality within 30 days after discharge, or discharge into hospice care. Therefore, it can be used to support the identification of patients requiring hospice as well as enhancing palliative care services in the hospital.
Impact
Further study is needed in this area to determine how long it takes for predictive models for mortality to become obsolete, allowing for the timely development of new models to support hospice and palliative care services.
更多查看译文
AI 理解论文
溯源树
样例
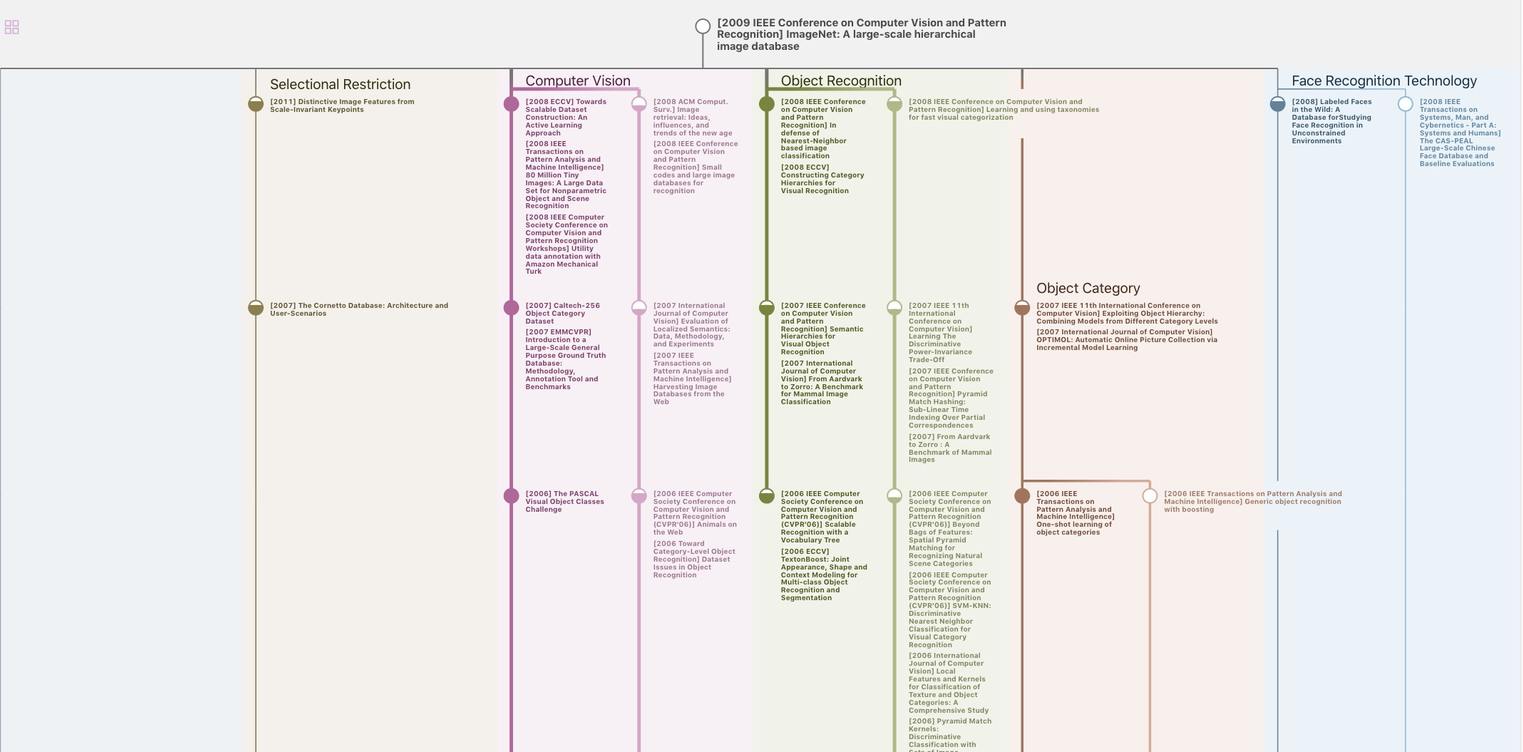
生成溯源树,研究论文发展脉络
Chat Paper
正在生成论文摘要