Larger Receptive Field and Context Information for Pose Estimation: Larger Gaussian Kernel
2023 International Conference on High Performance Big Data and Intelligent Systems (HDIS)(2023)
Abstract
The field of pose estimation has a wide range of application prospects in various industries in the current era. With the continuous development of deep learning techniques, the effects in the field of human pose estimation are constantly being optimized. However, due to the influence of factors such as occlusion and human posture variability, it is easy to lead to inaccurate identification of keypoints and even keypoints' missing and matching errors, there is still a lot of optimization space for the effect of pose estimation. In this paper, we propose an improvement to generate heatmaps in the training process: increase the radius of the Gaussian kernel and adjust the smoothness of Gaussian distribution to get a larger receptive field, encourage the model to use more context information in prediction, and control other parameters unchanged on the premise of using heatmap regression. Improve keypoint detection performance by optimizing this item. In the experiment, for the COCO-Keypoint data set, we selected different methods published in recent years, and after our improvement, we obtained better accuracy and performance than the original method, which proves the validity of our method.
MoreTranslated text
Key words
heatmap,Gaussian kernel radius,receptive field,Context information
AI Read Science
Must-Reading Tree
Example
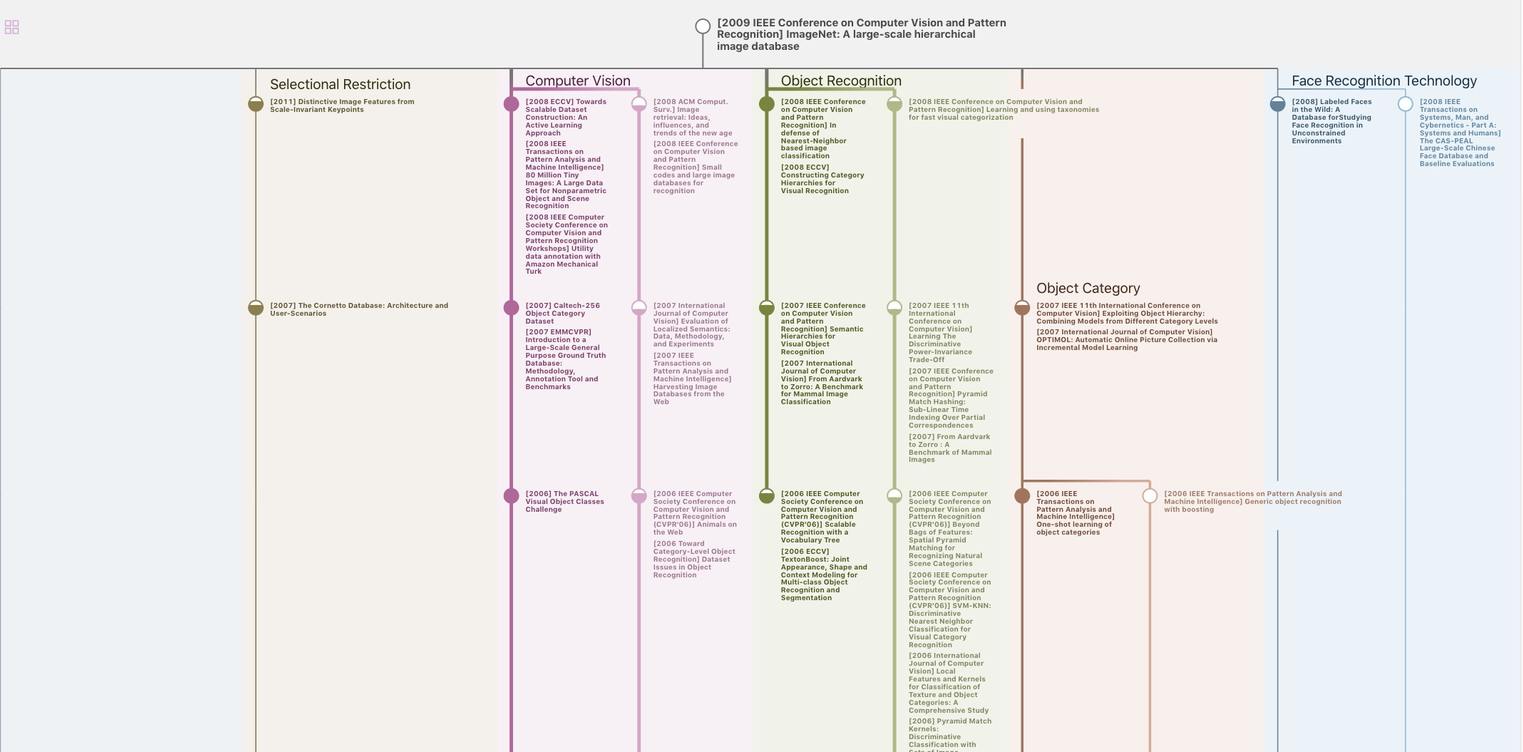
Generate MRT to find the research sequence of this paper
Chat Paper
Summary is being generated by the instructions you defined