Hierarchical fuzzy regression functions for mixed predictors and an application to real estate price prediction
Neural Computing and Applications(2024)
摘要
Categorical features appear in datasets from almost every practice area, including real estate datasets. One of the most critical handicaps of machine learning algorithms is that they are not designed to capture the qualitative nature of the categorical features, leading to sub-optimal predictions for the datasets with categorical observations. This study focuses on a new fuzzy regression functions framework, namely hierarchical fuzzy regression functions, that can handle categorical features properly for the regression task. The proposed framework is benchmarked with linear regression, support vector machines, deep neural networks, and adaptive neuro-fuzzy inference systems with real estate data having categorical features from six markets. It is observed that the proposed method produces better prediction performance for real estate price prediction than the benchmark methods in a wide variety of real estate markets. Since we provide all the required software codes to implement the proposed hierarchical fuzzy regression functions framework, our approach offers practitioners a readily applicable, high-performing tool for real estate price prediction and other regression problems involving categorical independent features.
更多查看译文
关键词
Categorical features,Deep learning,Market segmentation,Neural networks,Real estate valuation,Support vector machines
AI 理解论文
溯源树
样例
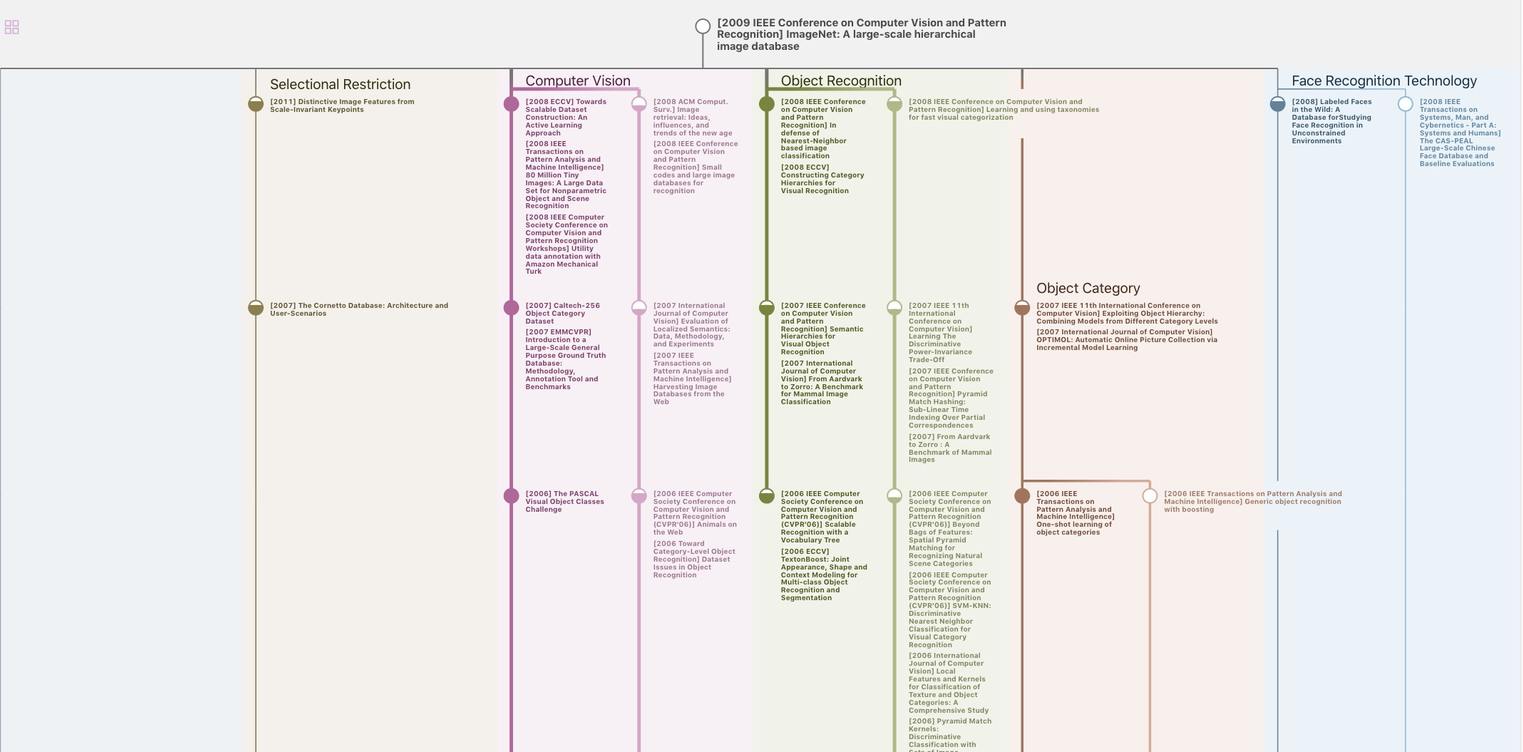
生成溯源树,研究论文发展脉络
Chat Paper
正在生成论文摘要