Graph Convolutional Networks Based on Neighborhood Expansion
EITCE '23: Proceedings of the 2023 7th International Conference on Electronic Information Technology and Computer Engineering(2024)
摘要
Graph neural networks (GNNs) are an efficient framework for learning graph-structured data and achieving state-of-the-art performance on many tasks, including node classification, link prediction, and graph classi-fication. Over-smoothing problem is troublesome in GNNs, that is, increasing the depth of the network leads to significant degradation in model performance. A large number of methods address it through regu-larization or residual concatenation, but these methods only mitigate the over-smoothing in some extent, which persists as the network depth increases. In this paper, we propose the Graph Convolutional Network Based on Neighborhood Expansion (NEGCN), which enables the shallow network aggregate multi-hop neighbor features information for node embedding. specifically, NEGCN uses Depth-First-Search to ex-pand 1-hop neighbors with similarity, so as to avoid the problem of over-smoothing. The robustness of NEGCN is further improved by combining with attention mechanism and graph sparsification methods. Extensive experiments demonstrate that our model has a superior performance on real-world graph learning benchmarks.
更多查看译文
AI 理解论文
溯源树
样例
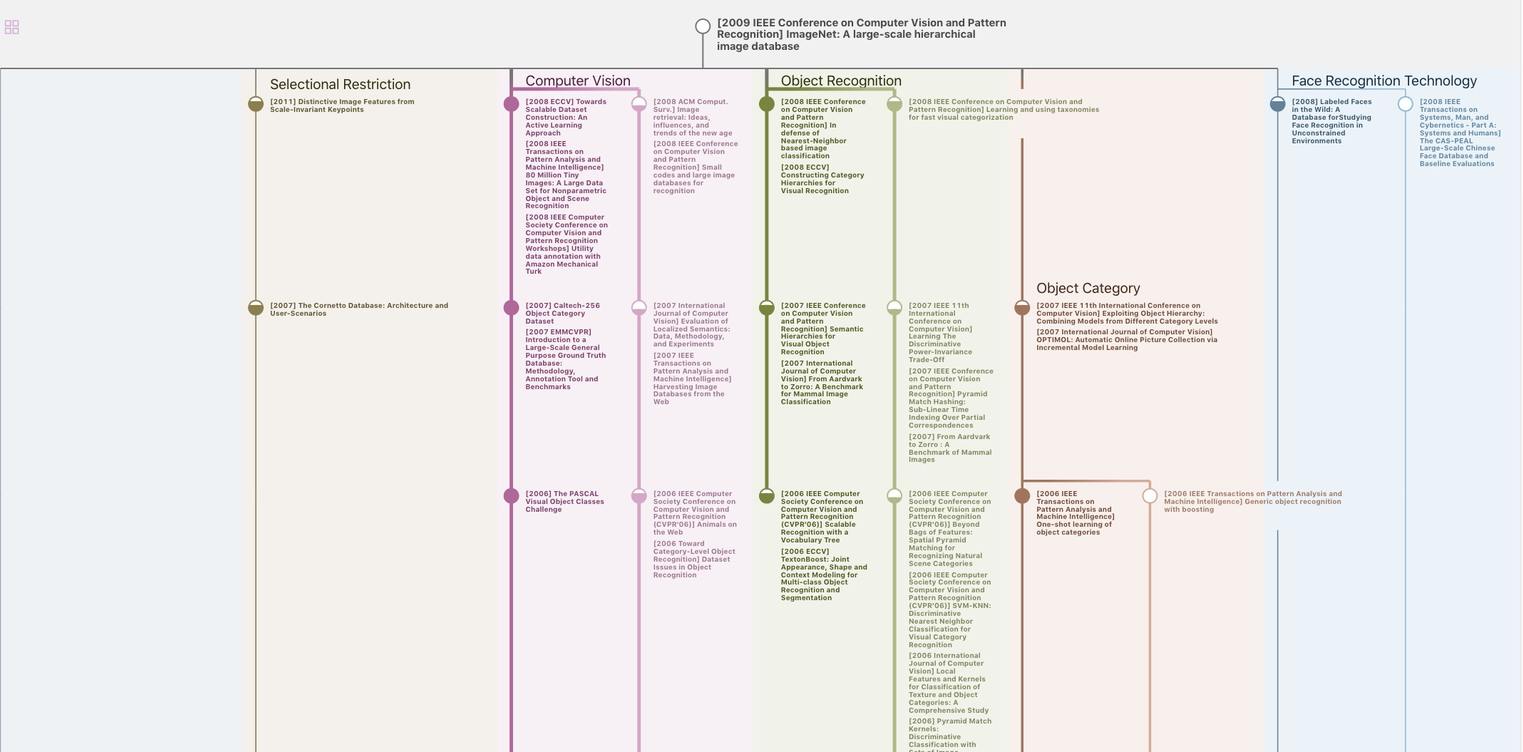
生成溯源树,研究论文发展脉络
Chat Paper
正在生成论文摘要