Lithium-ion battery remaining useful life prediction: a federated learning-based approach
Energy, Ecology and Environment(2024)
摘要
In line with Industry 5.0 principles, energy systems form a vital part of sustainable smart manufacturing systems. As an integral component of energy systems, the importance of Lithium-Ion (Li-ion) batteries cannot be overstated. Accurately predicting the remaining useful life (RUL) of these batteries is a paramount undertaking, as it impacts the overall reliability and sustainably of the smart manufacturing systems. Despite various existing methods have achieved good results, their applicability is limited due to the data isolation and data silos. To address the aforementioned challenges, this paper presents a novel federated learning (FL)-based approach that predicts the RUL of Li-ion batteries, thereby contributing to the sustainability of smart manufacturing. Firstly, a denoising recursive autoencoder-based transformer (DRAT) model is devised, focusing on extracting robust and latent features for RUL prediction under various conditions. Secondly, we propose an adaptive DRAT-based federated RUL framework (Fed-DRAT) for the collaborative modeling of Li-ion batteries RUL prediction for different energy systems. Specifically, an innovative adaptive model aggregation strategy is developed to equalize the contribution weights of different participating systems and improve model performance. Our extensive experiments with Li-ion batteries datasets indicate that our proposed DRAT significantly outperforms existing methods and demonstrates superior performance in different scenarios.
更多查看译文
关键词
Industry 5.0,Lithium-ion battery,Remaining useful life prediction,Transformer,Federated learning
AI 理解论文
溯源树
样例
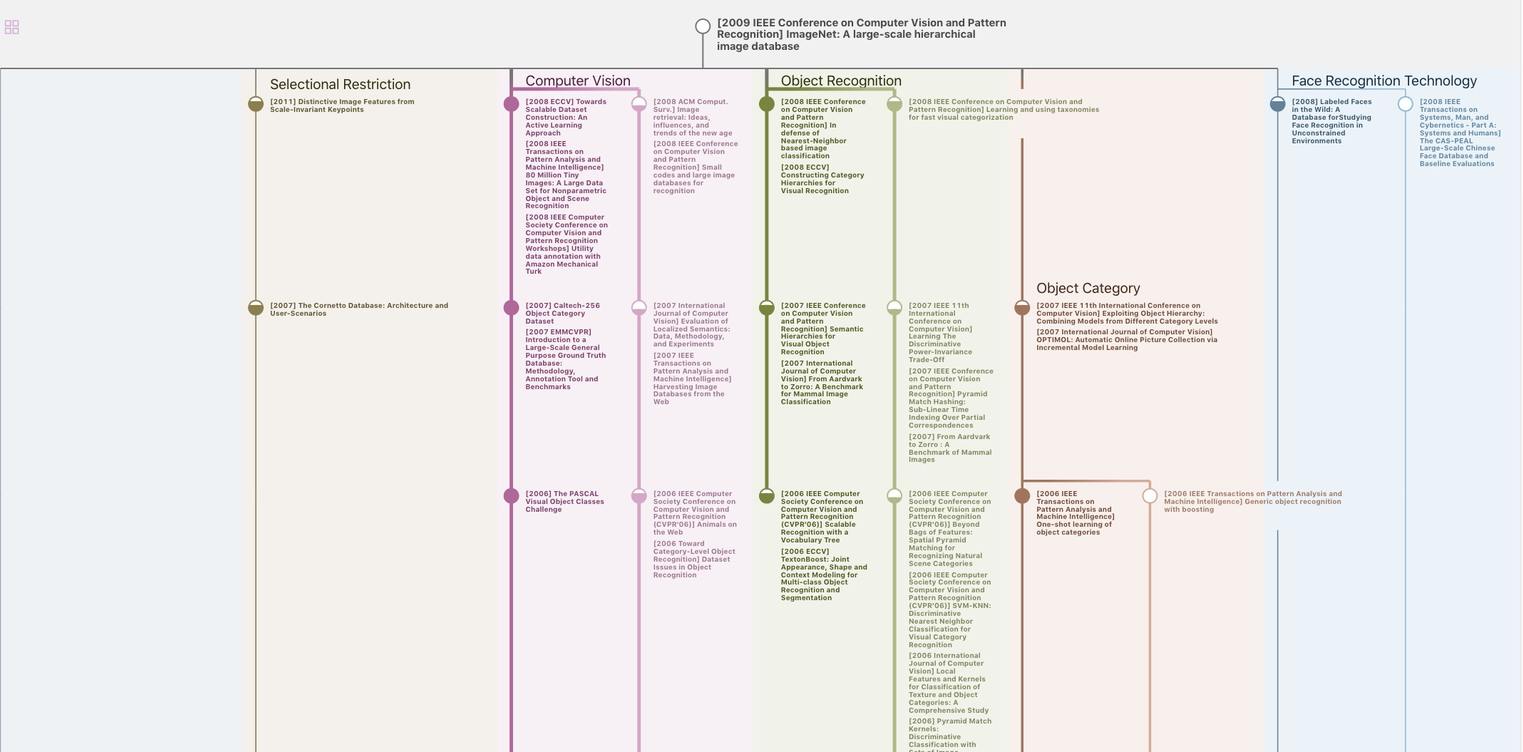
生成溯源树,研究论文发展脉络
Chat Paper
正在生成论文摘要