CA-PDBPR: category-aware privacy preserving POI recommendation using decentralized Bayesian personalized ranking
Applied Intelligence(2024)
摘要
Point-of-interest (POI) recommendation has gained significant traction recently due to the rising trend of location-based networks. Traditional approaches rely on a centralized collection of user data. Concerning privacy protection, decentralized federated learning employs model training on each user’s device with nearby collaborative training techniques. However, existing decentralized federated recommendations suffer from two major problems: (1) Privacy risks: existing approaches expose geographical location or co-rated items information when constructing user neighborhoods. (2) Performance limitations: existing approaches adopt a simple model without incorporating auxiliary information. To solve these, we propose CA-PDBPR (category-aware privacy preserving POI recommendation using decentralized Bayesian personalized ranking) to address the above challenges. Specifically, we introduce a novel privacy-enhanced neighborhood creation method utilizing POI category preferences to calculate decentralized user similarity through secret sharing technology, ensuring a higher level of privacy. Moreover, we integrate POI category information with a refined Bayesian personalized ranking (BPR) loss function to enhance recommendation performance. Experimental evaluations conducted on real-world datasets validate the effectiveness of the CA-PDBPR model, demonstrating enhanced recommendation quality while minimizing data exposure compared with state-of-the-art alternatives.
更多查看译文
关键词
Decentralized federated learning,POI recommendation,BPR,POI category
AI 理解论文
溯源树
样例
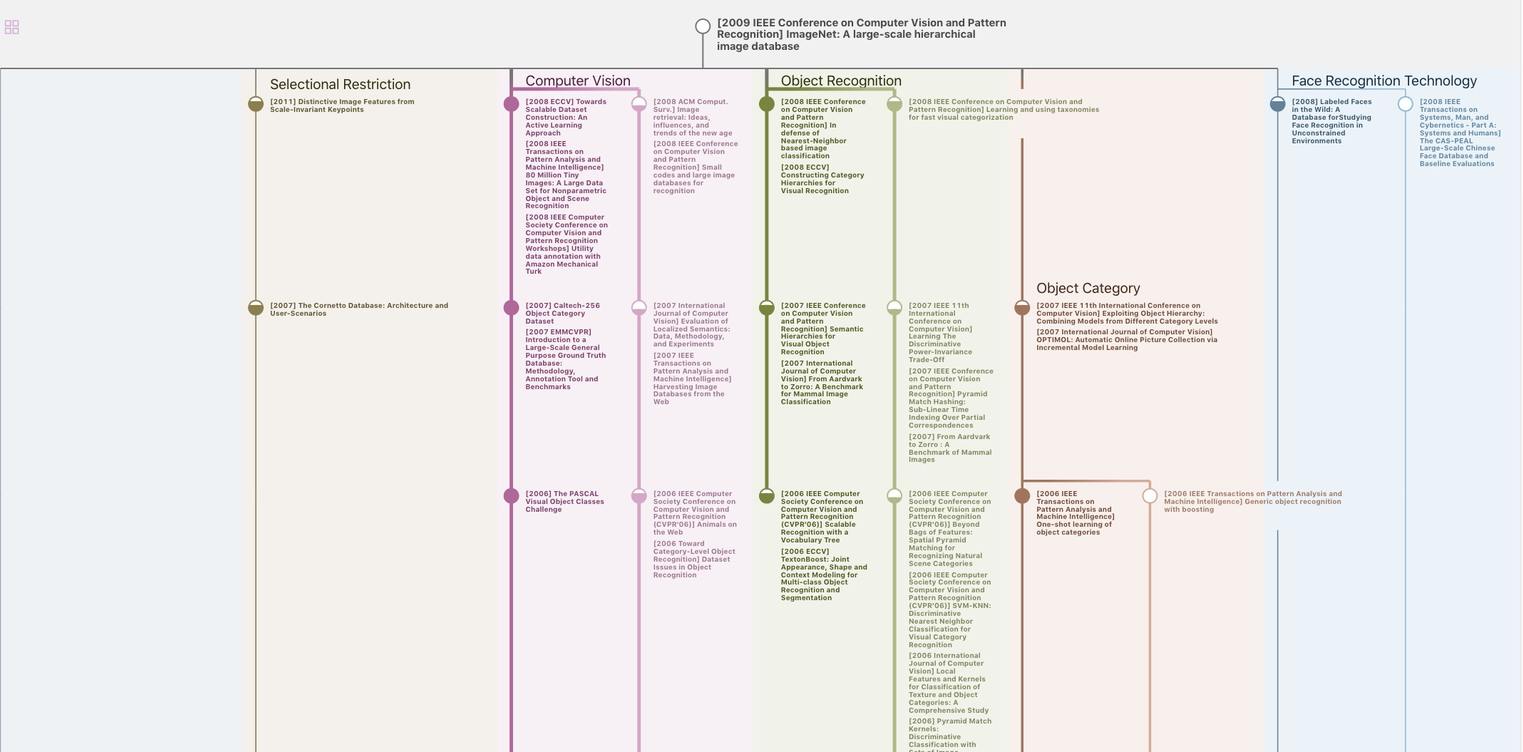
生成溯源树,研究论文发展脉络
Chat Paper
正在生成论文摘要