Intelligent fault diagnosis under imbalanced multivariate working conditions leveraging dynamic unsupervised domain adaptation with sample and margin regularization
MEASUREMENT SCIENCE AND TECHNOLOGY(2024)
摘要
Utilizing unsupervised domain adaptation for intelligent fault diagnosis (IFD) has demonstrated significant potential for ensuring the security of machinery systems. Nonetheless, the inherent imbalance attribute of collected data affects the performance of diagnostic model. Especially, for machines working under varied conditions, the acquired unlabeled data frequently exhibits diverse degrees of distributional deviations, thus further undermining the transferable model's generalization capability. To address this challenge, we introduce a method termed Dynamic Unsupervised Imbalanced Domain Adaptation (DUIDA) for IFD. Employment of class rebalancing and label-dependent margin regularization strategies optimizes the selection of decision boundaries which counteract the distributional deviations introduced by the imbalance. In addition, by integrating a dynamic weighting mechanism, encompassing both adversarial-based and MMD-based domain adaptation, our model becomes versatile across varied UIDA tasks, assigning higher weights to fundamental faulty features. Finally, our empirical analyses on two faulty bearing datasets substantiate the efficacy and superior performance of the proposed framework across diverse operational scenarios.
更多查看译文
关键词
fault diagnosis,class imbalance,domain adaptation,transfer learning,rolling bearing
AI 理解论文
溯源树
样例
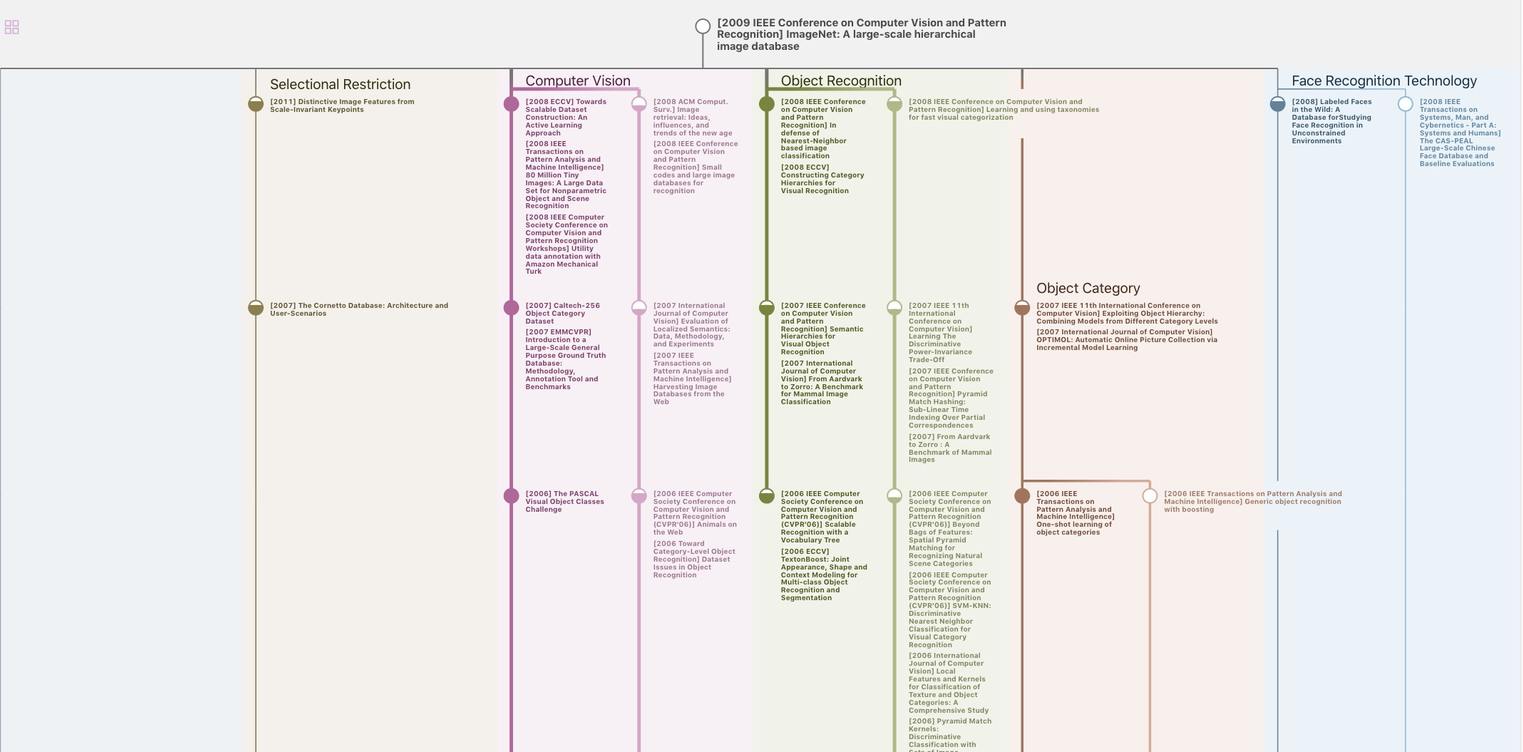
生成溯源树,研究论文发展脉络
Chat Paper
正在生成论文摘要