A Dynamic-Inner Manifold Broad Learning System With Coupled Time-Frequency Domain for Wind Speed Prediction
IEEE TRANSACTIONS ON INDUSTRIAL INFORMATICS(2024)
摘要
Wind speed prediction plays an important role in operating renewable energy power systems that incorporate wind power. However, wind speed prediction remains a significant challenge due to the non linearity, chaos, and nonstationarity of wind speed data. In this article, we propose a novel wind speed prediction model based on the dynamic-inner manifold broad learning system with coupled time-frequency domain (DiM-BLS-CTFD). We first propose the autovariational-mode decomposition (AVMD) algorithm to automatically transform nonstationary wind speed series data into a relatively more stationary series. Then, the dynamic-inner manifold learning (DiML) algorithm has been developed to extract the dynamic manifold structure with regression properties from the decomposed results by AVMD. Finally, building upon AVMD and DiML, DiM-BLS-CTFD is designed for wind speed prediction. DiM-BLS-CTFD is a unified framework that combines signal processing, feature extraction, and prediction. It effectively couples the multimodal time-frequency features of wind speed data, enhancing the accuracy of wind speed prediction. Compared with the original BLS model, DiM-BLS-CTFD showed an average reduction of 50.63% in root-mean-square error, 50.49% in mean absolute error, and 51.90% in symmetric mean absolute percentage error.
更多查看译文
关键词
Broad learning system (BLS),chaotic time series,dynamic feature extraction,dynamic latent modeling,manifold learning (ML),nonuniform embedding,variational-mode decomposition (VMD),wind speed prediction
AI 理解论文
溯源树
样例
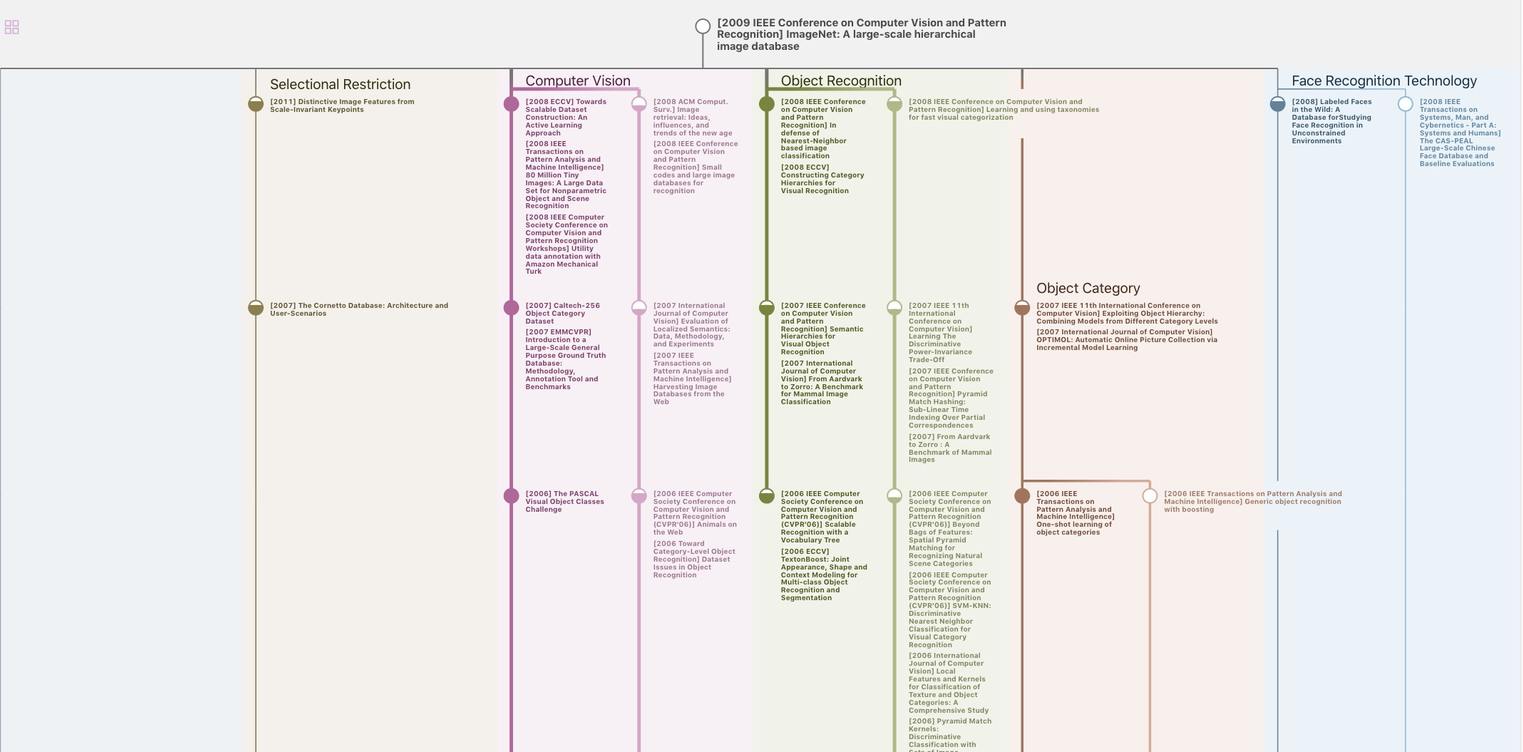
生成溯源树,研究论文发展脉络
Chat Paper
正在生成论文摘要