A Semantic Detection Method for Network Flows With Global and Generalized Nature
IEEE Transactions on Network and Service Management(2024)
摘要
Network threat detection and identification are essential tasks in the defense of cyberspace. However, current network threat detection methods have limitations such as narrow feature extraction, targeted feature effects, and limited generalization performance. Therefore, there is a need for a more comprehensive understanding and description of network behavior. As a result, we propose a global and generalized method for semantic detection of network flow to enhance the definition of flow data and representation of network behavior. To improve the problem of narrow feature range in existing methods, this paper designs three feature embedding methods that represent global, temporal, and local semantic correlations from both temporal and spatial dimensions: global embedding, position embedding, and learning embedding. In order to overcome the problem of existing methods only targeting specific behaviours, this article focuses on constructing global correlation features to replace the detection mode of building an inherent feature set. By utilizing text analysis features, we extract global embedding features containing network flow relationship information by constructing a topology heterogeneous graph between flows and bytes. This is combined with position embedding and learning embedding to complete data detection and behavior classification through input into the transformer encoder. We validated the effectiveness of our method in three scenarios: the Internet, the Internet of Things, and encryption. The final experimental results demonstrated that our proposed method outperformed existing advanced models. Furthermore, after incorporating global embedding representing international correlation relationships, the model’s classification accuracy was further improved.
更多查看译文
关键词
threat detection,Semantic analysis,topological relationship heterogeneous graph,graph convolutional network,transformer encoder
AI 理解论文
溯源树
样例
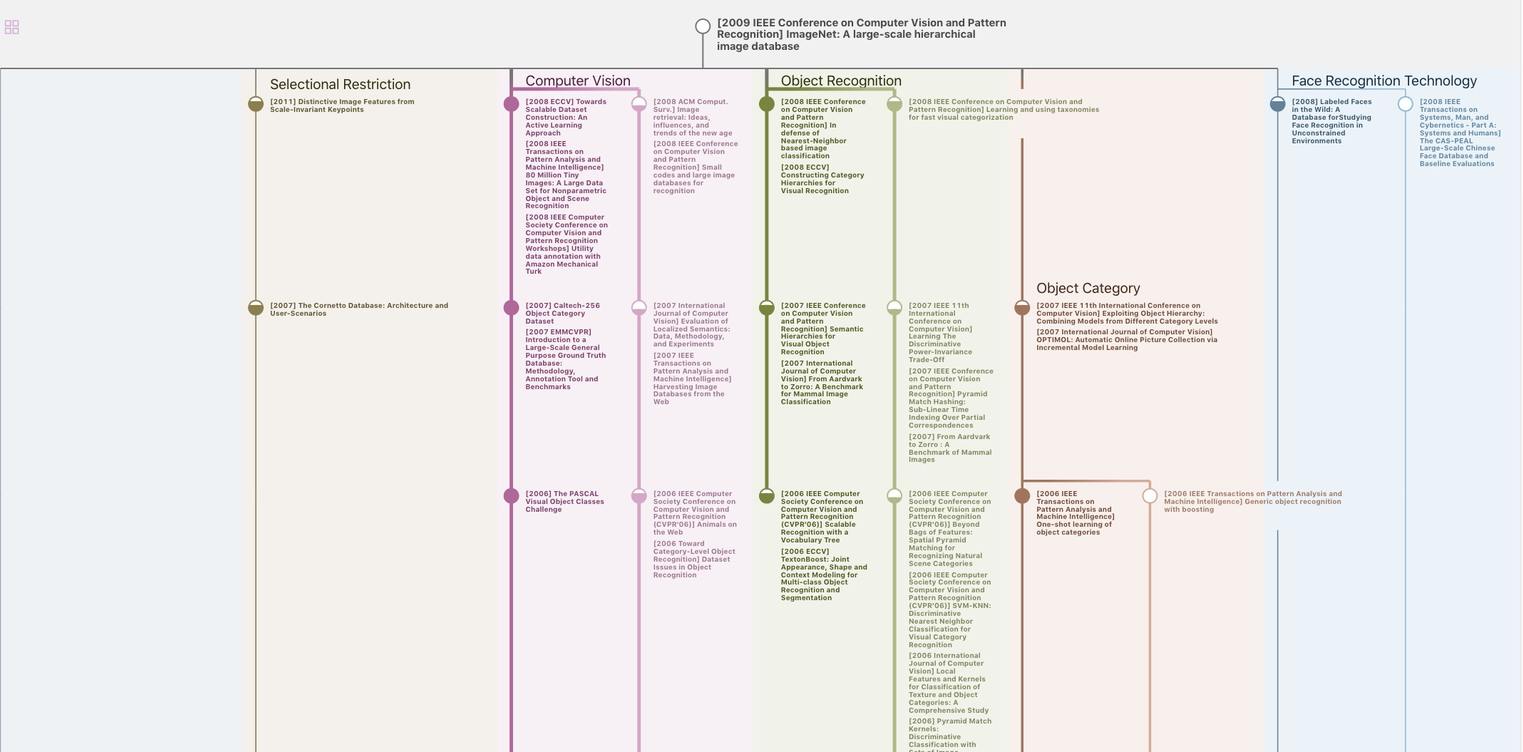
生成溯源树,研究论文发展脉络
Chat Paper
正在生成论文摘要