A ranking-based problem transformation method for weakly supervised multi-label learning
Pattern Recognition(2024)
摘要
Problem transformation is a simple yet effective framework for multi-label learning, where the original multi-label problem can be transformed into a series of single-label subproblems. However, the existing problem transformation methods have difficulties in handling label defect issues in real applications, e.g. multi-label learning with missing labels, partial multi-label learning and noisy multi-label learning. To deal with these issues, we propose a novel problem transformation method named EPR (i.e., Ensemble of Pairwise Ranking learners) applicable to various multi-label tasks. In EPR, the weakly supervised multi-label problem is converted into an ensemble of supervised single-label patterns due to pairwise label ranking, which successfully enhances label correlation exploration and improves the utilization of instances with defect labels. Moreover, an ensemble pruning mechanism is presented to heuristically balance the model performance and efficiency. Extensive experiments demonstrate the effectiveness of EPR against state-of-the-art algorithms in diverse multi-label learning scenarios.
更多查看译文
关键词
Multi-label learning,Problem transformation,Pairwise label correlation,Ensemble learning,Multi-label learning with missing labels,Partial multi-label learning
AI 理解论文
溯源树
样例
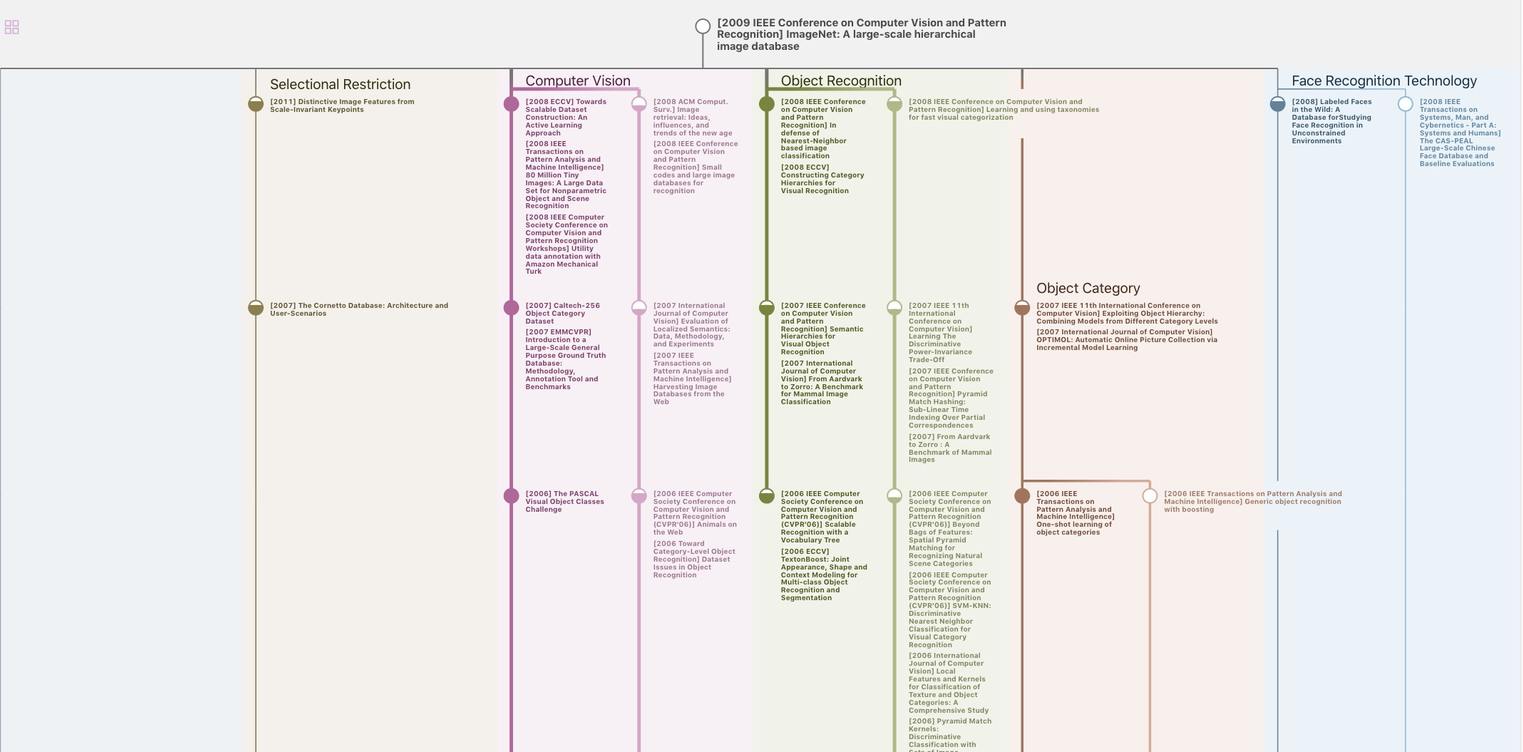
生成溯源树,研究论文发展脉络
Chat Paper
正在生成论文摘要