DeepSeq2Drug: An Expandable Ensemble End-to-end Anti-viral Drug Repurposing Benchmark Framework by Multi-modal Embeddings and Transfer Learning
Computers in Biology and Medicine(2024)
摘要
Drug repurposing is promising in multiple scenarios, such as emerging viral outbreak controls and cost reductions of drug discovery. Traditional graph-based drug repurposing methods are limited to fast, large-scale virtual screens, as they constrain the counts for drugs and targets and fail to predict novel viruses or drugs. Moreover, though deep learning has been proposed for drug repurposing, only a few methods have been used, including a group of pre-trained deep learning models for embedding generation and transfer learning. Hence, we propose DeepSeq2Drug to tackle the shortcomings of previous methods. We leverage multi-modal embeddings and an ensemble strategy to complement the numbers of drugs and viruses and to guarantee the novel prediction. This framework (including the expanded version) involves four modal types: six NLP models, four CV models, four graph models, and two sequence models. In detail, we first make a pipeline and calculate the predictive performance of each pair of viral and drug embeddings. Then, we select the best embedding pairs and apply an ensemble strategy to conduct anti-viral drug repurposing. To validate the effect of the proposed ensemble model, a monkeypox virus (MPV) case study is conducted to reflect the potential predictive capability. This framework could be a benchmark method for further pre-trained deep learning optimization and anti-viral drug repurposing tasks. We also build software further to make the proposed model easier to reuse. The code and software are freely available at http://deepseq2drug.cs.cityu.edu.hk.
更多查看译文
关键词
Anti-viral Drug,Drug Repurposing,Transfer Learning,Ensemble Learning
AI 理解论文
溯源树
样例
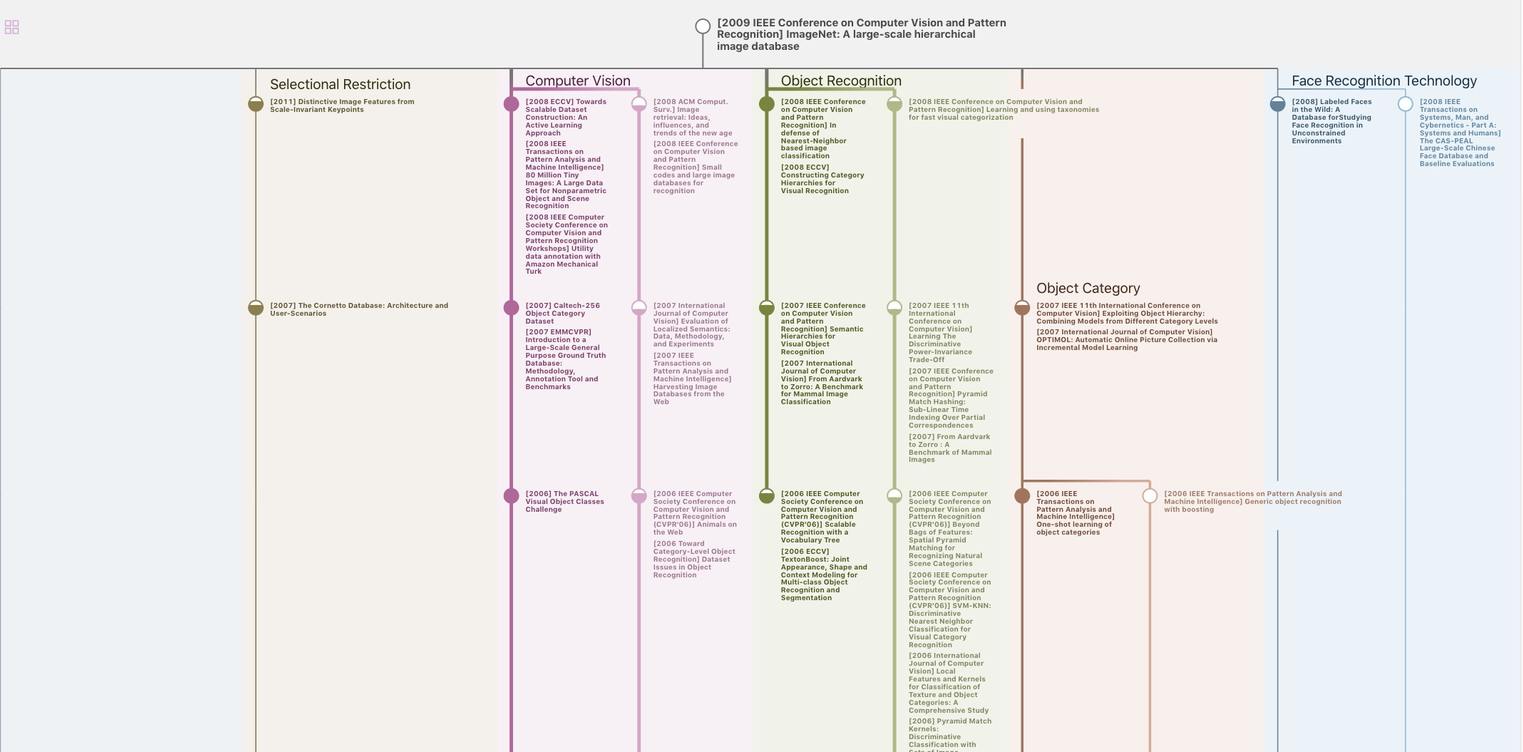
生成溯源树,研究论文发展脉络
Chat Paper
正在生成论文摘要