Real-Time Trajectory Synthesis with Local Differential Privacy
CoRR(2024)
摘要
Trajectory streams are being generated from location-aware devices, such as
smartphones and in-vehicle navigation systems. Due to the sensitive nature of
the location data, directly sharing user trajectories suffers from privacy
leakage issues. Local differential privacy (LDP), which perturbs sensitive data
on the user side before it is shared or analyzed, emerges as a promising
solution for private trajectory stream collection and analysis. Unfortunately,
existing stream release approaches often neglect the rich spatial-temporal
context information within trajectory streams, resulting in suboptimal utility
and limited types of downstream applications. To this end, we propose RetraSyn,
a novel real-time trajectory synthesis framework, which is able to perform
on-the-fly trajectory synthesis based on the mobility patterns privately
extracted from users' trajectory streams. Thus, the downstream trajectory
analysis can be performed on the high-utility synthesized data with privacy
protection. We also take the genuine behaviors of real-world mobile travelers
into consideration, ensuring authenticity and practicality. The key components
of RetraSyn include the global mobility model, dynamic mobility update
mechanism, real-time synthesis, and adaptive allocation strategy. We conduct
extensive experiments on multiple real-world and synthetic trajectory datasets
under various location-based utility metrics, encompassing both streaming and
historical scenarios. The empirical results demonstrate the superiority and
versatility of our proposed framework.
更多查看译文
关键词
Local differential privacy,trajectory streams
AI 理解论文
溯源树
样例
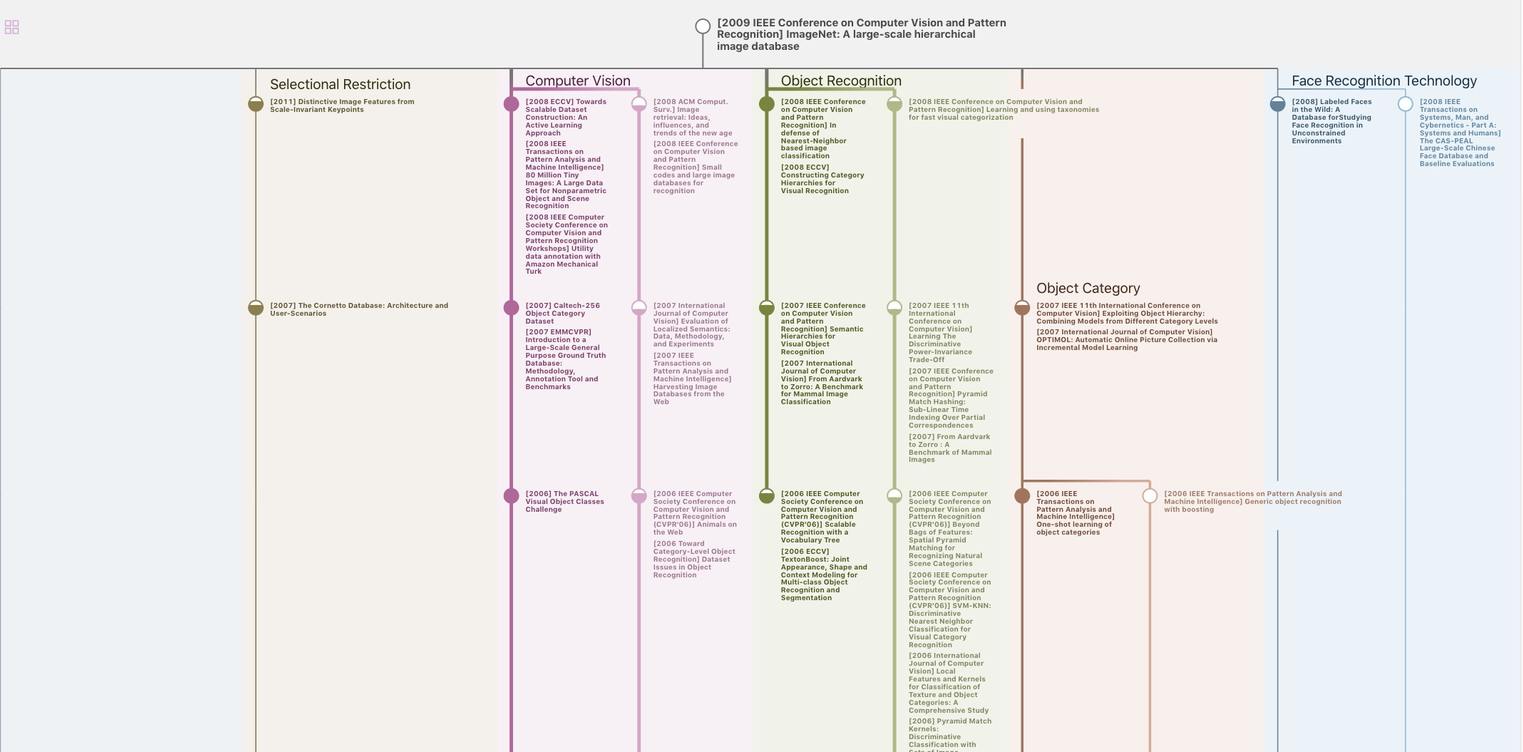
生成溯源树,研究论文发展脉络
Chat Paper
正在生成论文摘要