Bayesian Optimization for Identification of Optimal Biological Dose Combinations in Personalized Dose-Finding Trials
arxiv(2024)
摘要
Early phase, personalized dose-finding trials for combination therapies seek
to identify patient-specific optimal biological dose (OBD) combinations, which
are defined as safe dose combinations which maximize therapeutic benefit for a
specific covariate pattern. Given the small sample sizes which are typical of
these trials, it is challenging for traditional parametric approaches to
identify OBD combinations across multiple dosing agents and covariate patterns.
To address these challenges, we propose a Bayesian optimization approach to
dose-finding which formally incorporates toxicity information into both the
initial data collection process and the sequential search strategy. Independent
Gaussian processes are used to model the efficacy and toxicity surfaces, and an
acquisition function is utilized to define the dose-finding strategy and an
early stopping rule. This work is motivated by a personalized dose-finding
trial which considers a dual-agent therapy for obstructive sleep apnea, where
OBD combinations are tailored to obstructive sleep apnea severity. To compare
the performance of the personalized approach to a standard approach where
covariate information is ignored, a simulation study is performed. We conclude
that personalized dose-finding is essential in the presence of heterogeneity.
更多查看译文
AI 理解论文
溯源树
样例
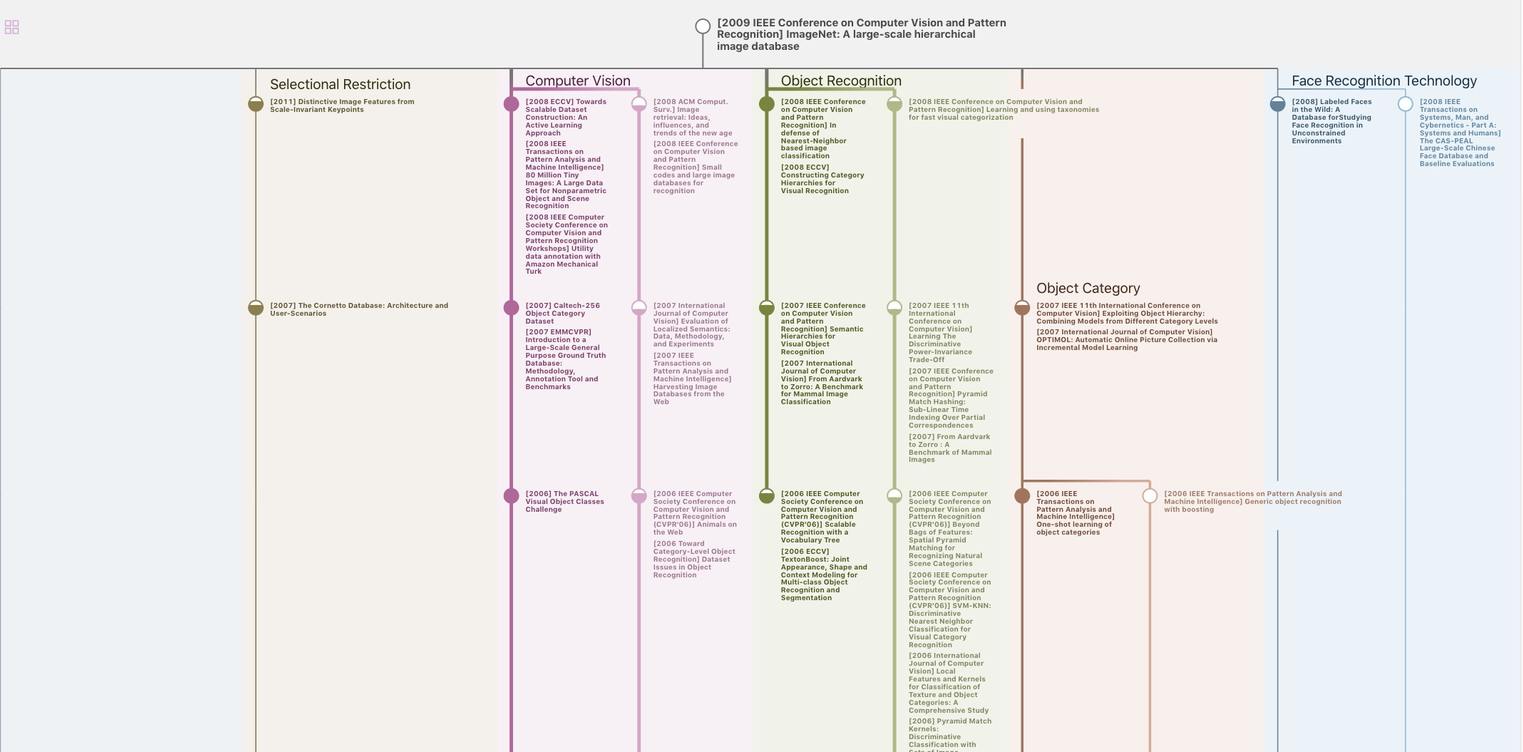
生成溯源树,研究论文发展脉络
Chat Paper
正在生成论文摘要