Residual Connections Harm Self-Supervised Abstract Feature Learning
arxiv(2024)
摘要
We demonstrate that adding a weighting factor to decay the strength of
identity shortcuts within residual networks substantially improves semantic
feature learning in the state-of-the-art self-supervised masked autoencoding
(MAE) paradigm. Our modification to the identity shortcuts within a VIT-B/16
backbone of an MAE boosts linear probing accuracy on ImageNet from 67.3
72.3
serves an essential role in facilitating gradient propagation, it may have a
harmful side effect of reducing capacity for abstract learning by virtue of
injecting an echo of shallower representations into deeper layers. We
ameliorate this downside via a fixed formula for monotonically decreasing the
contribution of identity connections as layer depth increases. Our design
promotes the gradual development of feature abstractions, without impacting
network trainability. Analyzing the representations learned by our modified
residual networks, we find correlation between low effective feature rank and
downstream task performance.
更多查看译文
AI 理解论文
溯源树
样例
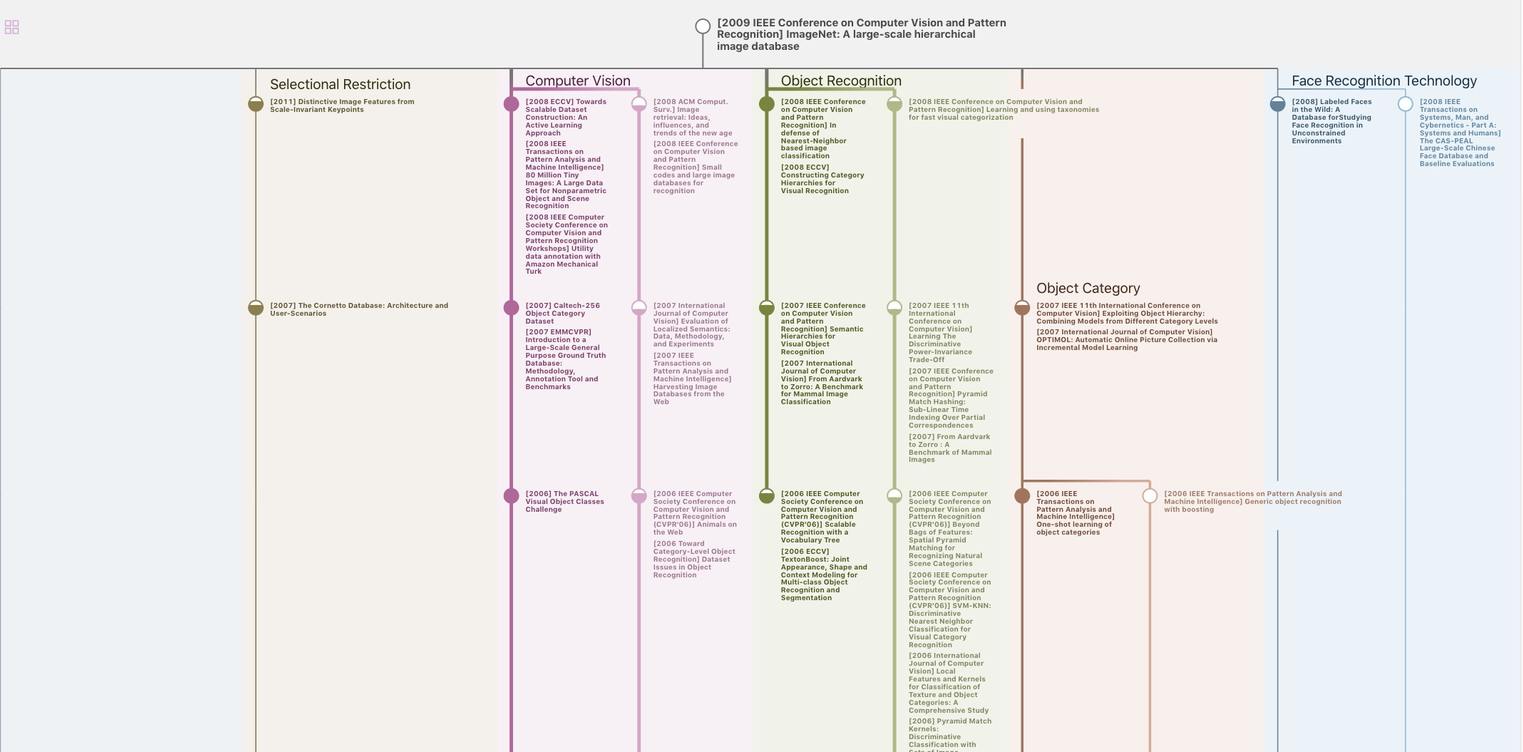
生成溯源树,研究论文发展脉络
Chat Paper
正在生成论文摘要