Explainable artificial intelligence framework for urban global digital elevation model correction based on the SHapley additive explanation-random forest algorithm considering spatial heterogeneity and factor optimization
International Journal of Applied Earth Observation and Geoinformation(2024)
Abstract
Satellite global digital elevation models (GDEMs) suffer from positive biases in urban areas due to building artifacts. While various machine learning (ML)-based methods have been proposed to remove these biases, their generalizability is limited by spatial heterogeneity and redundancy in prediction factors across different regions. Therefore, to investigate the spatial heterogeneity of prediction factors and address the problem of factor redundancy in ML-based model prediction, this paper proposes an explainable artificial intelligence framework (XAI) for correcting urban GDEMs using the SHapley additive explanation (SHAP)-random forest (RF) algorithm. The performance of the proposed model for correcting the 30-m COPDEM (COPDEM30) was demonstrated in New York City. The results were compared with the first global Forests-And-Buildings removed DEM (FABDEM) and three classical RF-based models without considering spatial heterogeneity and (or) factor optimization. The results indicate that each factor contributes differently to the correction of COPDEM30 across the regions, showing distinct regional characteristics and spatial heterogeneity. The constructed model is more applicable to regions with similar features to the training regions. In comparison to the three traditional RF-based models in areas with training points, the proposed method obtains the high accuracy. Specifically, while the Root Mean Square Error (RMSE) and Mean Absolute Error (MAE) values of the classical RF models ranged between 2.601 m and 2.724 m, and 1.686 m and 1.785 m, respectively, the proposed method achieves an RMSE of 2.258 m and an MAE of 1.436 m. Moreover, the proposed method reduces the RMSE (MAE) of the original COPDEM30 from 7.652 m (4.858 m) to 3.797 m (2.404 m), when applied to an area without providing training points. In summary, the XAI framework based on SHAP-RF can effectively quantify the contribution of prediction factors on GDEM correction, both globally and locally, which is conducive to the construction and improvement of the prediction factor system for urban GDEM correction in different regions. It also provides a reference for improving the performance of ML-based spatial prediction in geosciences.
MoreTranslated text
Key words
Global digital elevation model,SHAP,Feature optimization,Accuracy
AI Read Science
Must-Reading Tree
Example
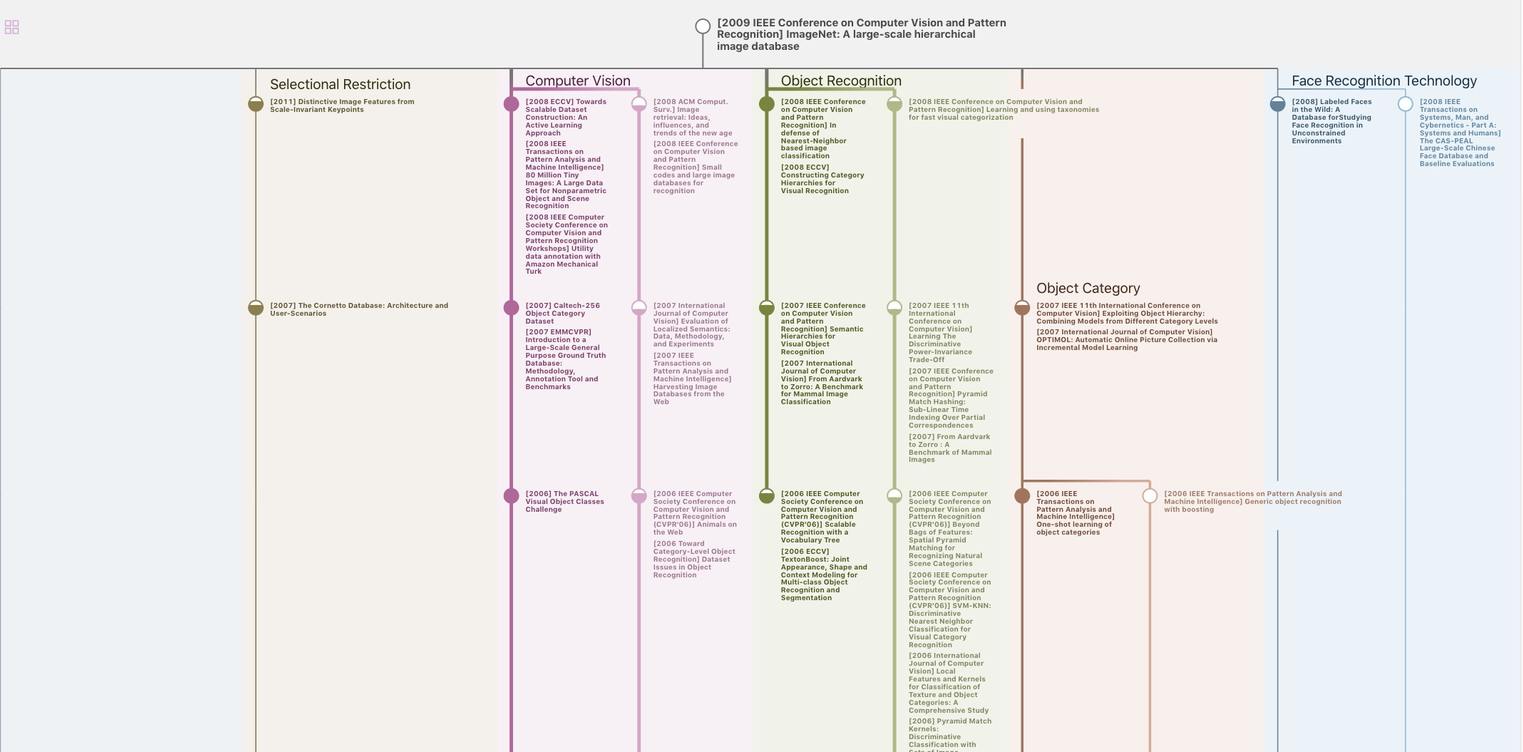
Generate MRT to find the research sequence of this paper
Chat Paper
Summary is being generated by the instructions you defined