Recent Advancements in Machine Learning-Based Bloodstream Infection Prediction: A Systematic Review and Meta-analysis of Diagnostic Test Accuracy
medrxiv(2024)
摘要
Abstract. Purpose: Bloodstream infections (BSIs) present significant public health challenges. With the advent of machine learning (ML), promising predictive models have been developed. This study evaluates their performance through a systematic review and meta-analysis. Methods: We performed a comprehensive systematic review across multiple databases, including PubMed, IEEE Xplore, ScienceDirect, ACM Digital Library, SpringerLink, Web of Science, Scopus, and Google Scholar. Eligible studies focused on BSIs within any hospital setting, employing ML models as the diagnostic test. We evaluated the risk of bias with the Quality Assessment of Diagnostic Accuracy Studies (QUADAS-2) checklist and assessed the quality of evidence using the Grading of Recommendations, Assessment, Development, and Evaluation (GRADE) approach. Models reporting the area under the receiver operating characteristic curve (AUROC) were included in the meta-analysis to identify key performance drivers. Results: After screening, a total of 30 studies were eligible for synthesis, from which 41 models and 8 data types were extracted. Most of the studies were carried out in the inpatient settings (n=17; 56%), followed by the emergency department (ED) settings (n=7; 23%), and followed by the ICU settings (n=6; 20%). The reported AUROCs in the hospital inpatients settings, ranged from 0.51-0.866, in the ICU settings AUROCs ranged from 0.668-0.970, and in the emergency department (ED) settings the AUROCs of the models ranged from 0.728-0.844. One study reported prospective cohort study, while two prospectively validated their models. In the meta-analysis, laboratory tests, Complete Blood Count/Differential Count (CBC/DC), and ML model type contributed the most to model performance. Conclusion: This systematic review and meta-analysis show that on retrospective data, individual ML models can accurately predict BSIs at different stages of patient trajectory. Although they enable early prediction of BSI, a comprehensive approach to integrate data types and models is necessary. Systematic reporting, externally validated, and clinical implementation studies are needed to establish clinical confidence.
### Competing Interest Statement
The authors have declared no competing interest.
### Funding Statement
Financial support for this study was provided by the Computational Sepsis Mining and Modelling project through the Norwegian University of Science and Technology Health Strategic Area.
### Author Declarations
I confirm all relevant ethical guidelines have been followed, and any necessary IRB and/or ethics committee approvals have been obtained.
Yes
I confirm that all necessary patient/participant consent has been obtained and the appropriate institutional forms have been archived, and that any patient/participant/sample identifiers included were not known to anyone (e.g., hospital staff, patients or participants themselves) outside the research group so cannot be used to identify individuals.
Yes
I understand that all clinical trials and any other prospective interventional studies must be registered with an ICMJE-approved registry, such as ClinicalTrials.gov. I confirm that any such study reported in the manuscript has been registered and the trial registration ID is provided (note: if posting a prospective study registered retrospectively, please provide a statement in the trial ID field explaining why the study was not registered in advance).
Yes
I have followed all appropriate research reporting guidelines, such as any relevant EQUATOR Network research reporting checklist(s) and other pertinent material, if applicable.
Yes
All data produced in the present work are contained in the manuscript
更多查看译文
AI 理解论文
溯源树
样例
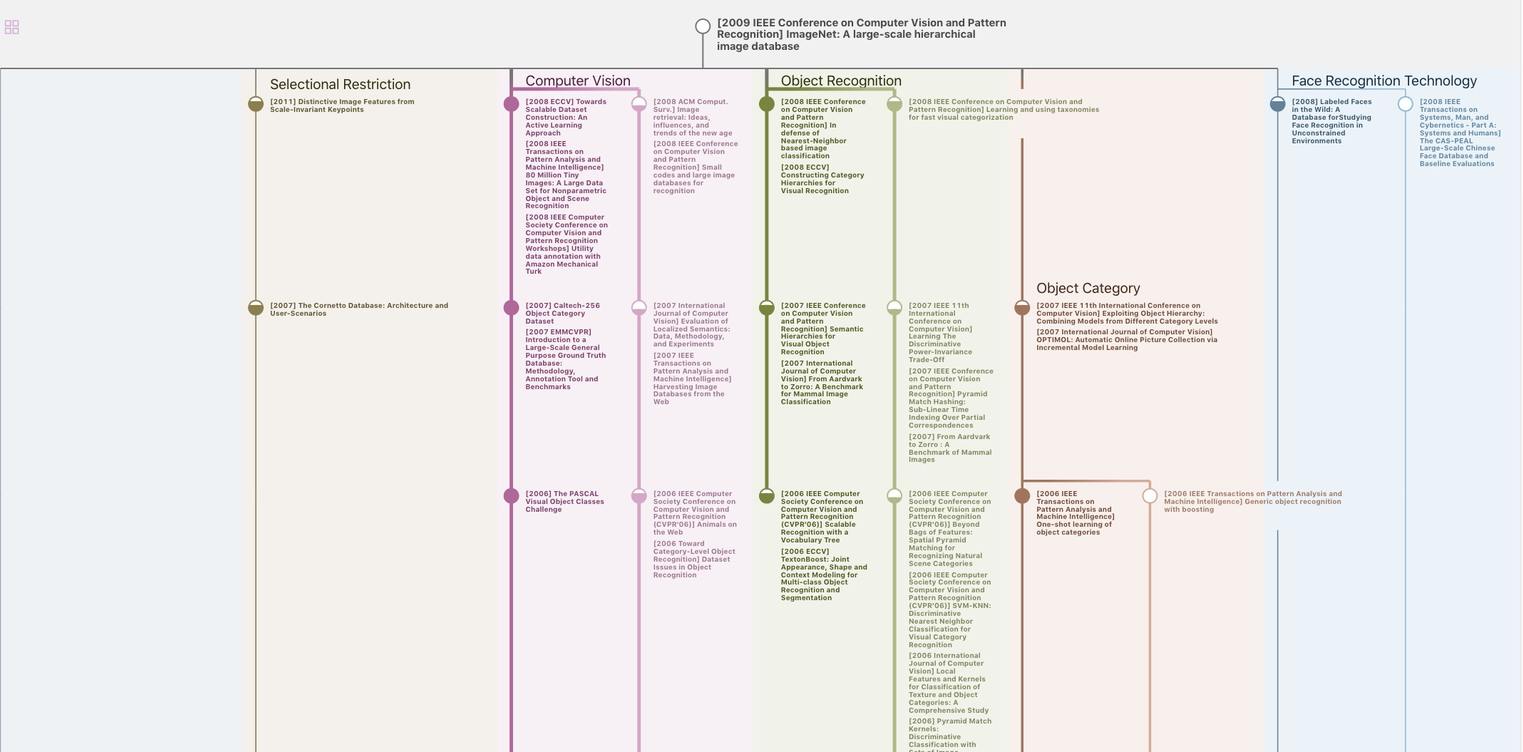
生成溯源树,研究论文发展脉络
Chat Paper
正在生成论文摘要