Controlled and Real-Life Investigation of Optical Tracking Sensors in Smart Glasses for Monitoring Eating Behaviour using Deep Learning: Cross-Sectional Study (Preprint)
crossref(2024)
摘要
BACKGROUND Gaining insight into people's dietary habits is crucial for designing effective interventions aimed at promoting a healthy lifestyle. Automatic monitoring of food intake offers the opportunity to mitigate the significant limitations associated with traditional self-reporting approaches. OBJECTIVE The aim of this study is to introduce a technique for automated detection of chewing activity and identification of eating segments utilizing data from optical tracking sensors embedded within smart glasses. METHODS Utilizing a deep learning framework, the system analyses facial muscle movements indicative of chewing, leveraging patented OCO™ sensors. To account for the temporal dependence of chewing events in real-life scenarios, a Hidden Markov Model (HMM) is integrated, allowing to infer eating segments in real-life. The study comprehensively explores the impact of sensor positions over different facial muscles, window sizes, and various deep learning architectures on detection performance, evaluating the system's performance across controlled and real life settings. RESULTS The extensive evaluations show that our method for automatic chewing detection achieves precision of 0.92, recall of 0.89, and F1 score of 0.91 in a laboratory setting with 126 participants and precision of 0.95, recall of 0.82, and F1-score of 0.88 in a real-life setting with 8 participants. CONCLUSIONS This research contributes significantly to the advancement of dietary monitoring technology by introducing a non-invasive tool for tracking eating behavior. The promising results demonstrated by the presented method in both laboratory and real-life settings underscores its potential for practical application in diverse contexts.
更多查看译文
AI 理解论文
溯源树
样例
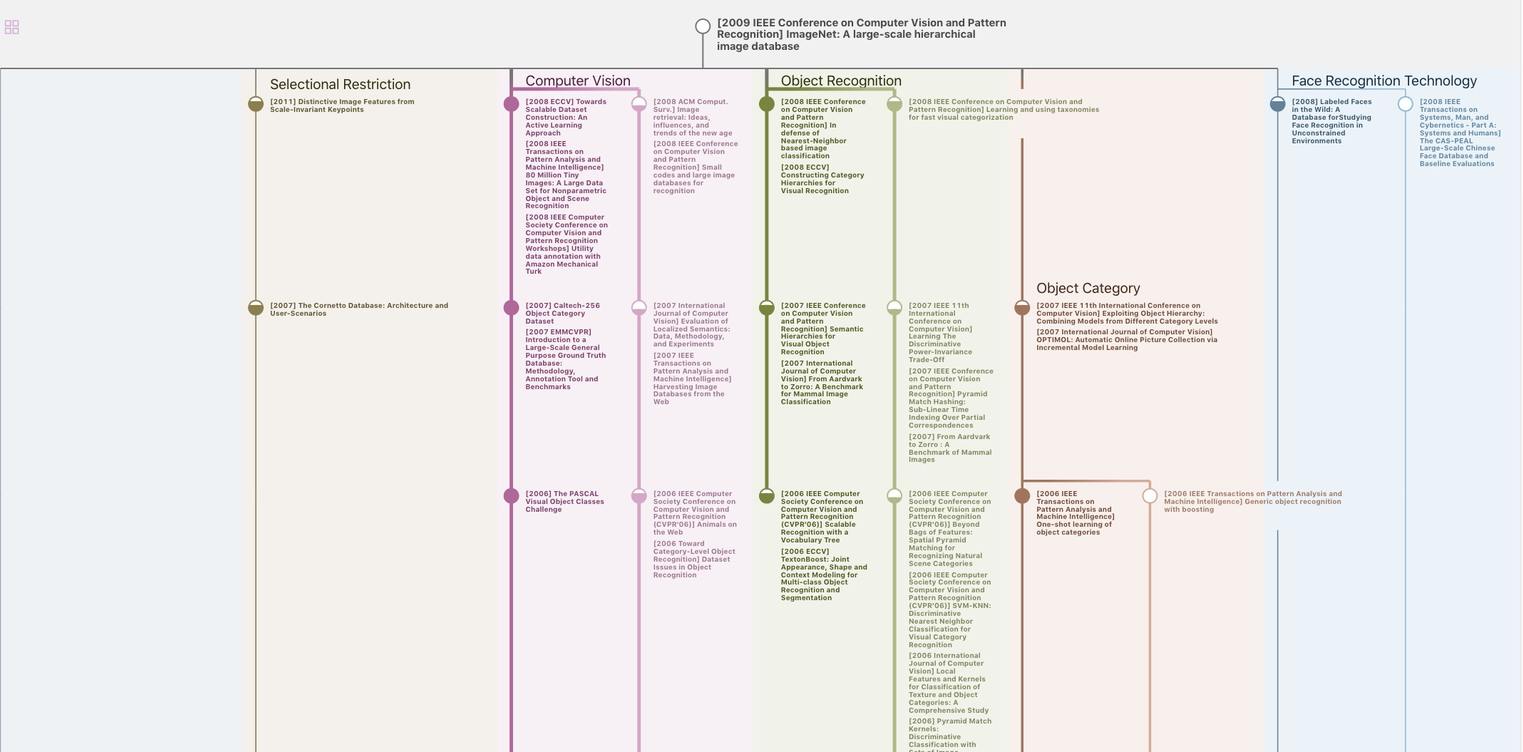
生成溯源树,研究论文发展脉络
Chat Paper
正在生成论文摘要