An efficient center-based method for real-time pig posture recognition and tracking
Applied Intelligence(2024)
摘要
Detecting, tracking, and recognizing the posture of individual pigs are the primary computer vision tasks of many camera-based decision support tools for precise livestock monitoring. Recently, the use of deep learning approaches in computer vision, particularly convolutional neural networks (CNNs), has led to performance never before possible. However, the heavy constraints of pig environments such as instinctive grouping and similar animal appearance limit the effectiveness of state-of-the-art approaches popular in other application fields. To tackle these problems, we propose a fully anchor-free center-based CNN framework that detects pigs, classifies their postures, and tracks them throughout an image sequence. Input images are first fed into two sub-networks that detect pig posture and generate a global appearance map from which local appearance vectors corresponding to the detected pig centers can be extracted. Next, an assignment strategy uses spatial and appearance metrics to associate each pig detected in the frame with one of the tracked pig trajectories in a video sequence. As shown by the experiments, our real-time method significantly outperforms state-of-the-art approaches for pig detection, posture recognition, and tracking.
更多查看译文
关键词
Object tracking,Anchor-free,Data augmentation,Re-identification,Livestock monitoring
AI 理解论文
溯源树
样例
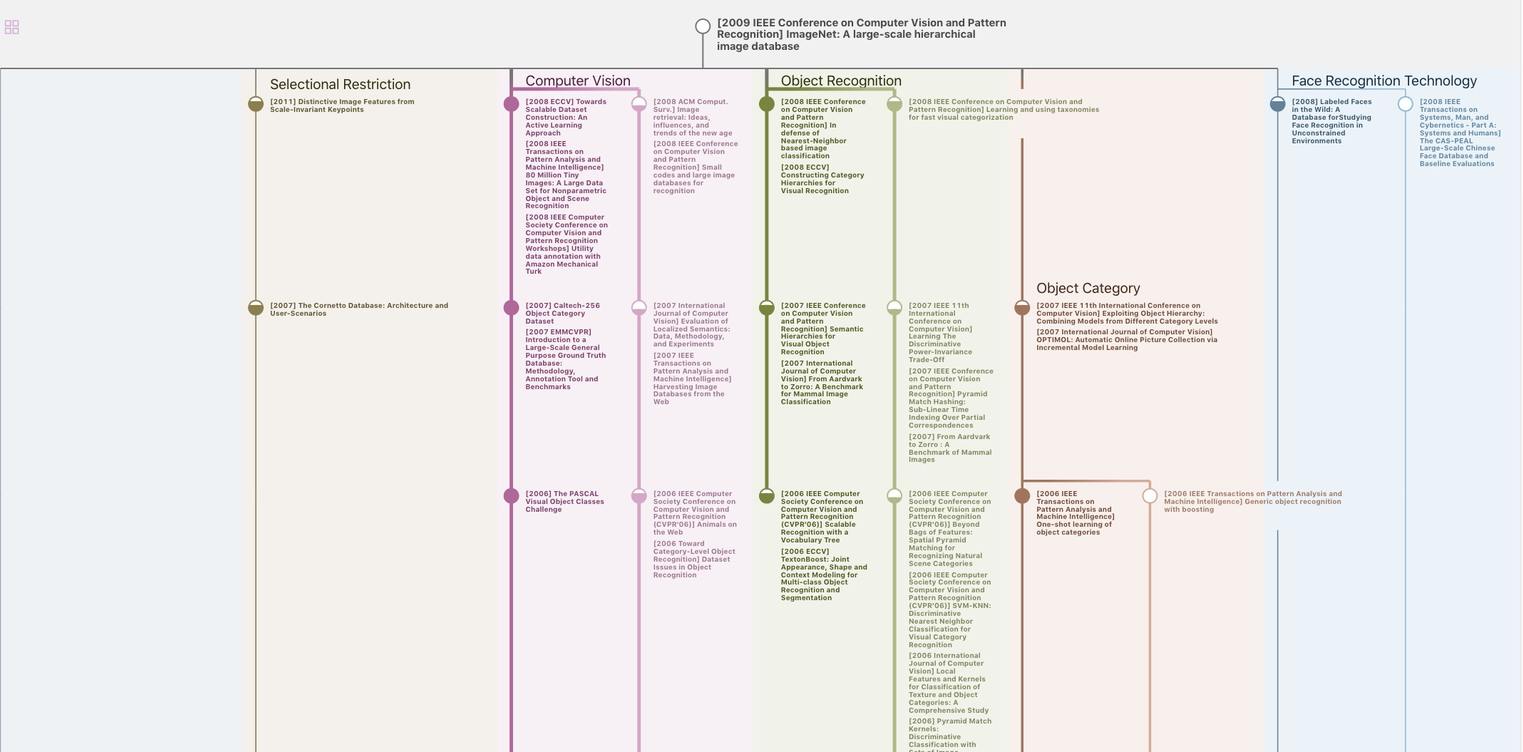
生成溯源树,研究论文发展脉络
Chat Paper
正在生成论文摘要