Low Complexity Deep Learning Based Coordinated Beamforming for mmWave Massive MIMO Vehicular Networks
2024 Panhellenic Conference on Electronics & Telecommunications (PACET)(2024)
摘要
Enabling high mobility in millimeter-wave (mmWave) systems is crucial for next-generation wireless communication systems. Challenges arise in applications such as vehicular communications and wireless virtual/augmented reality. MmWave systems grapple with concerns related to narrow beams, signal vulnerability to obstructions that affect coverage, and the necessity for frequent handovers. Additionally, identifying optimal beamforming vectors in large antenna array mmWave systems involves substantial training overhead, impacting efficiency. This paper introduces a novel solution to address challenges in enabling high mobility in mmWave systems. The approach combines deep learning (DL) and coordinated beamforming to support applications such as vehicular communications and wireless virtual/augmented reality. The solution involves multiple coordinating base stations (BSs) serving a mobile user, utilizing a single pilot training sequence. A DL model predicts beamforming vectors based on received signals, providing reliable coverage, low latency, and minimal training overhead for highly mobile mmWave applications. Simulations demonstrate its effectiveness in high-mobility scenarios, approaching the performance of an optimal solution, a genie-aided solution that knows the optimal beamforming vectors without any training overhead.
更多查看译文
关键词
Millimeter wave,coordinated beamforming,deep learning,data rates
AI 理解论文
溯源树
样例
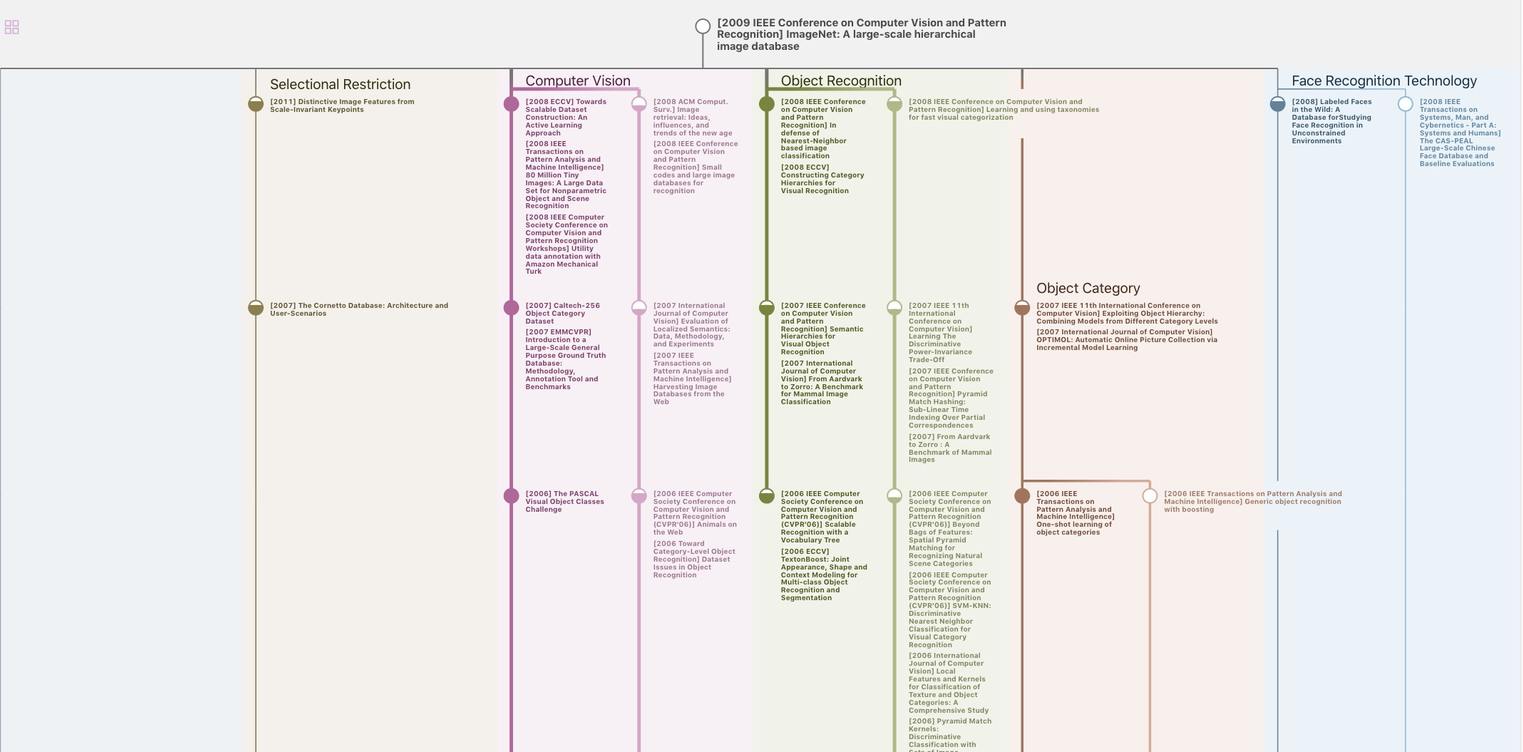
生成溯源树,研究论文发展脉络
Chat Paper
正在生成论文摘要