Soft Calibration of Online DGA Sensors Using Semi-Supervised S3TR for Power Transformer.
IEEE Trans. Instrum. Meas.(2024)
摘要
Dissolved gas analysis (DGA) is one of the key techniques for the condition monitoring of power transformers. With the development of online DGA sensors, condition monitoring has shifted from manual to online. However, these sensors are not as accurate as expected so they may provide inaccurate even misleading maintenance suggestions. To ensure the accuracy of data, these sensors are required to be carefully calibrated. Thus, a novel method is proposed to calibrate online sensors using manually measured data. However, the calibration is not an easy task because manual measurements are evaluated much sparser than online sensor (more than 1 month vs. 15 minutes), leading to a training set containing a few labeled samples (manual data) and a large amount of unlabeled samples (online data). To deal with such a dataset, a Semi-Supervised Safe Tri-Training Regression (S3TR) is proposed to build the calibration model. The proposed method adds reliable labels to the data during the training thus easing the difficulty of the dataset. The efficacy of the proposed method is demonstrated by evaluating a DGA dataset collected from in-service power transformers. The superiority of the proposed method is confirmed through comparing it with popular calibration methods.
更多查看译文
关键词
transformer diagnosis,dissolved gas analysis (DGA),sensor calibration,semi-supervised regression,small data
AI 理解论文
溯源树
样例
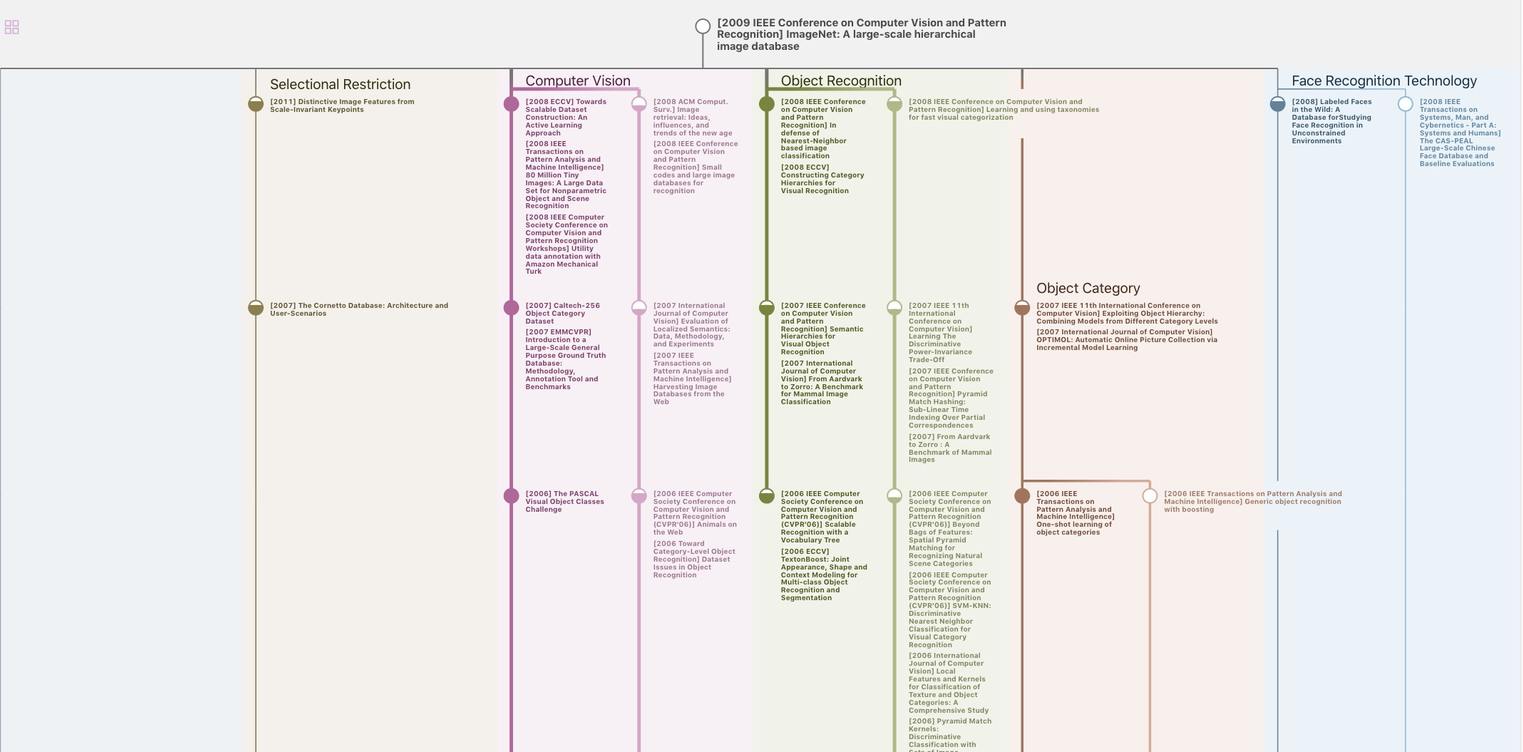
生成溯源树,研究论文发展脉络
Chat Paper
正在生成论文摘要