FDT-Net: Deep-Learning Network for Thin-Cloud Removal in Remote Sensing Image Using Frequency Domain Training Strategy
IEEE Geoscience and Remote Sensing Letters(2024)
摘要
Earth’s surface is covered by thin-cloud throughout the year, which greatly limits the application of remote sensing (RS) images obtained at a high cost. Currently, deep-learning technology has received widespread attention in the field of thin-cloud removal from RS images. The parameter training process of the deep network in this letter is divided into two stages according to the frequency domain feature of training samples. In the first stage, the loss between the thin-cloud removal image and the corresponding clear image is minimized by the full-frequency domain training. In the second stage, the parameters of the first stage are frozen, and the high-frequency features of the thin-cloud image are integrated into the Decoder module for network training. Furthermore, the corresponding attention module of the dual-tree wavelet is designed. For better training in the second stage, the high-frequency loss function based on three-level wavelet transform is designed. Compared with the highest average values of peak signal to noise ratio (PSNR) and structural similarity (SSIM) of traditional methods, FDT-Net achieves an improvement by up to 33.4108% and 7.5184%, respectively. In quantitative experiments, compared with the highest average values of PSNR and SSIM among deep-learning methods, FDT-Net has an improvement by 2.1505% and 0.9123%, respectively. Therefore, the proposed deep-learning network FDT-Net can significantly extend the temporal and spatial range of RS image application under thin-cloud weather conditions. The code of model is available at https://github.com/chonghaozhan/FDT-Net.
更多查看译文
关键词
deep learning,convolutional neural networks,image restoration,cloud removal
AI 理解论文
溯源树
样例
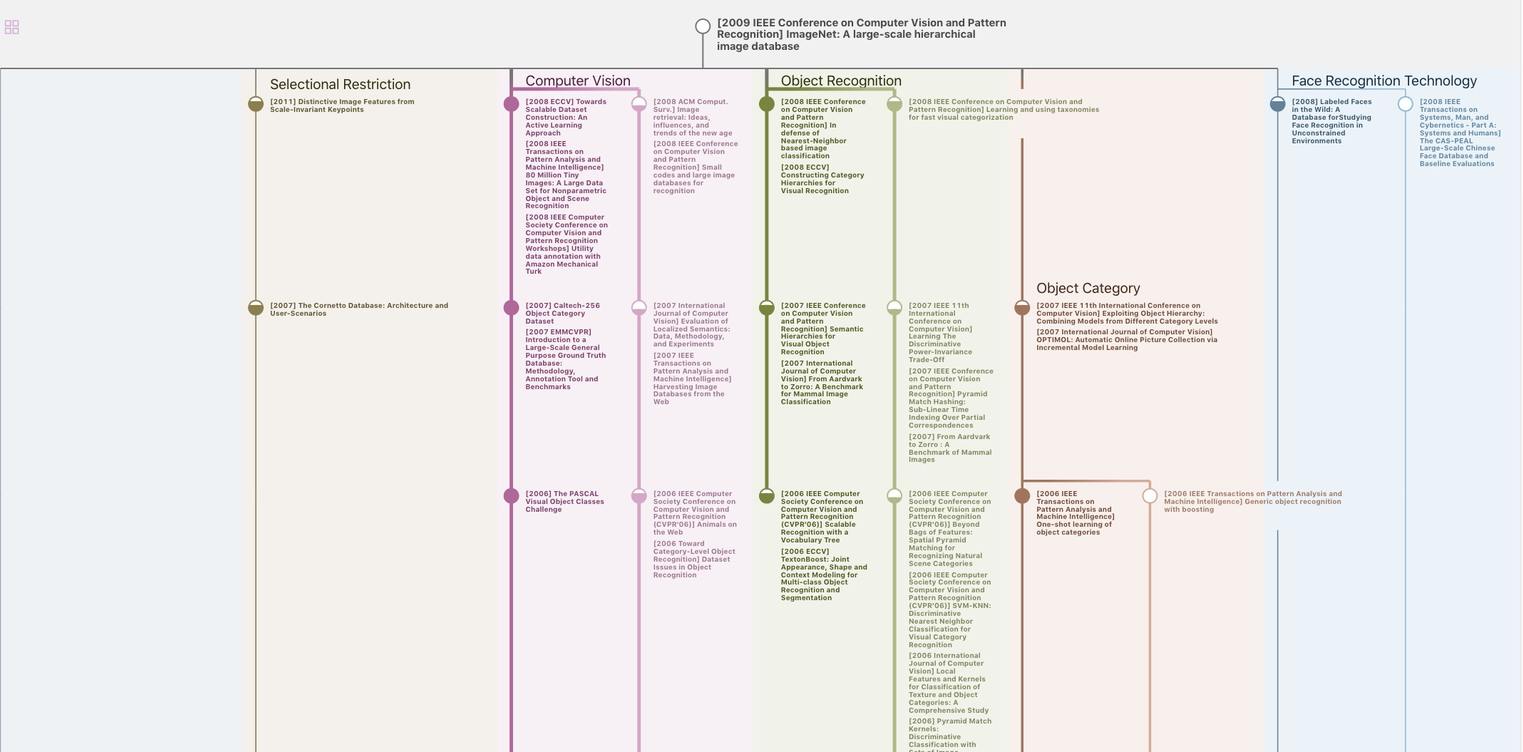
生成溯源树,研究论文发展脉络
Chat Paper
正在生成论文摘要