Moving Target Defense Approach for Secure Relay Selection in Vehicular Networks
Vehicular Communications(2024)
Abstract
Ensuring the security and reliability of cooperative vehicle-to-vehicle (V2V) communications is an extremely challenging task due to the dynamic nature of vehicular networks as well as the delay-sensitive wireless medium. In this context, the moving target defense (MTD) paradigm has been proposed to overcome the challenges of conventional solutions based on static network services and configurations. Specifically, the MTD approach involves the dynamic altering of network configurations to improve resilience to cyberattacks. Nevertheless, the current MTD solution for cooperative networks poses several limitations, such as that they are not well-suited for highly dynamic environments; they require high synchronization modules that are resource-intensive and difficult to implement; and finally, they rely heavily on attack-defense models, which may not always be accurate or comprehensive to use. To overcome these challenges, the proposed approach introduces an adaptive defense strategy within the MTD framework. This strategy proposes an intelligent spatiotemporal diversification-based MTD scheme to defend against eavesdropping attacks in cooperative V2V networks. It involves altering the system configuration spatially through relay selection and adjusting the percentage of injected fake data over time. This approach aims to balance reducing intercept probability while ensuring high throughput. Our methodology involves modeling the configuration of vehicular relays and data injection patterns as a Markov decision process, followed by applying deep reinforcement learning to determine the optimal configuration. We then iteratively evaluate the intercept probability and the percentage of transmitted real data for each configuration until convergence is achieved. To optimize the security-real data percentage (S-RDP), we developed a two-agent framework, namely MTD-DQN-RSS & MTD-DQN-RSS-RDP. The first agent, MTD-DQN-RSS, tries to minimize the intercept probability by injecting additional fake data, which in turn reduces the overall RDP, while the second agent, MTD-DQN-RSS-RDP, attempts to inject a sufficient amount of fake data to achieve a target S-RDP. Finally, extensive simulation results are conducted to demonstrate the effectiveness of our proposed solution, which improved system security by almost 28% and 49%, respectively, compared to the conventional relay selection approach.
MoreTranslated text
Key words
Cooperative networks,data injection,deep Q-networks,intercept probability,moving target defense (MTD),spatiotemporal diversity,and vehicular networks
AI Read Science
Must-Reading Tree
Example
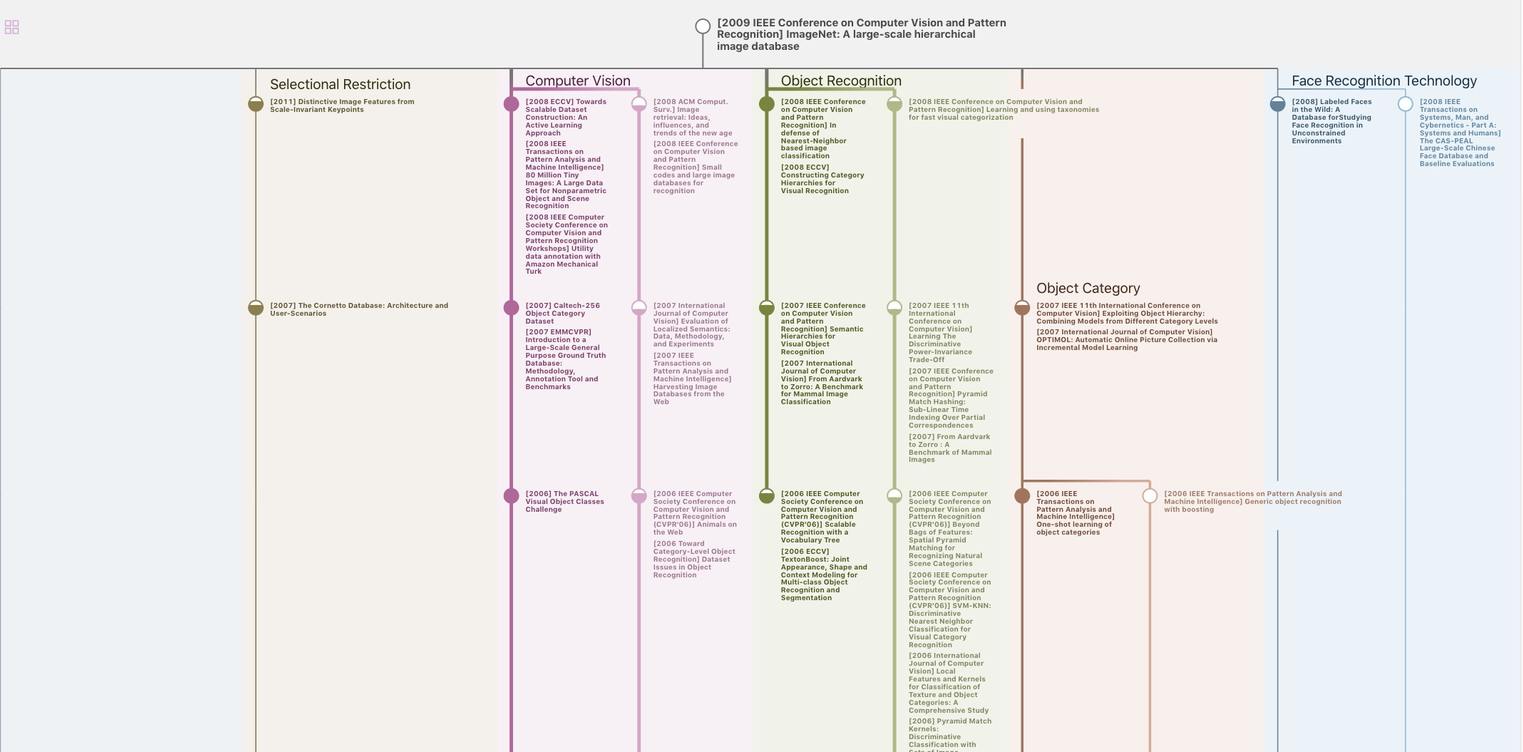
Generate MRT to find the research sequence of this paper
Chat Paper
Summary is being generated by the instructions you defined