A Novel Local Linear Embedding Algorithm Via Local Mutual Representation for Bearing Fault Diagnosis
RELIABILITY ENGINEERING & SYSTEM SAFETY(2024)
关键词
Bearing fault diagnosis,Locally linear embedding algorithm,Feature extraction,Local mutual representation
AI 理解论文
溯源树
样例
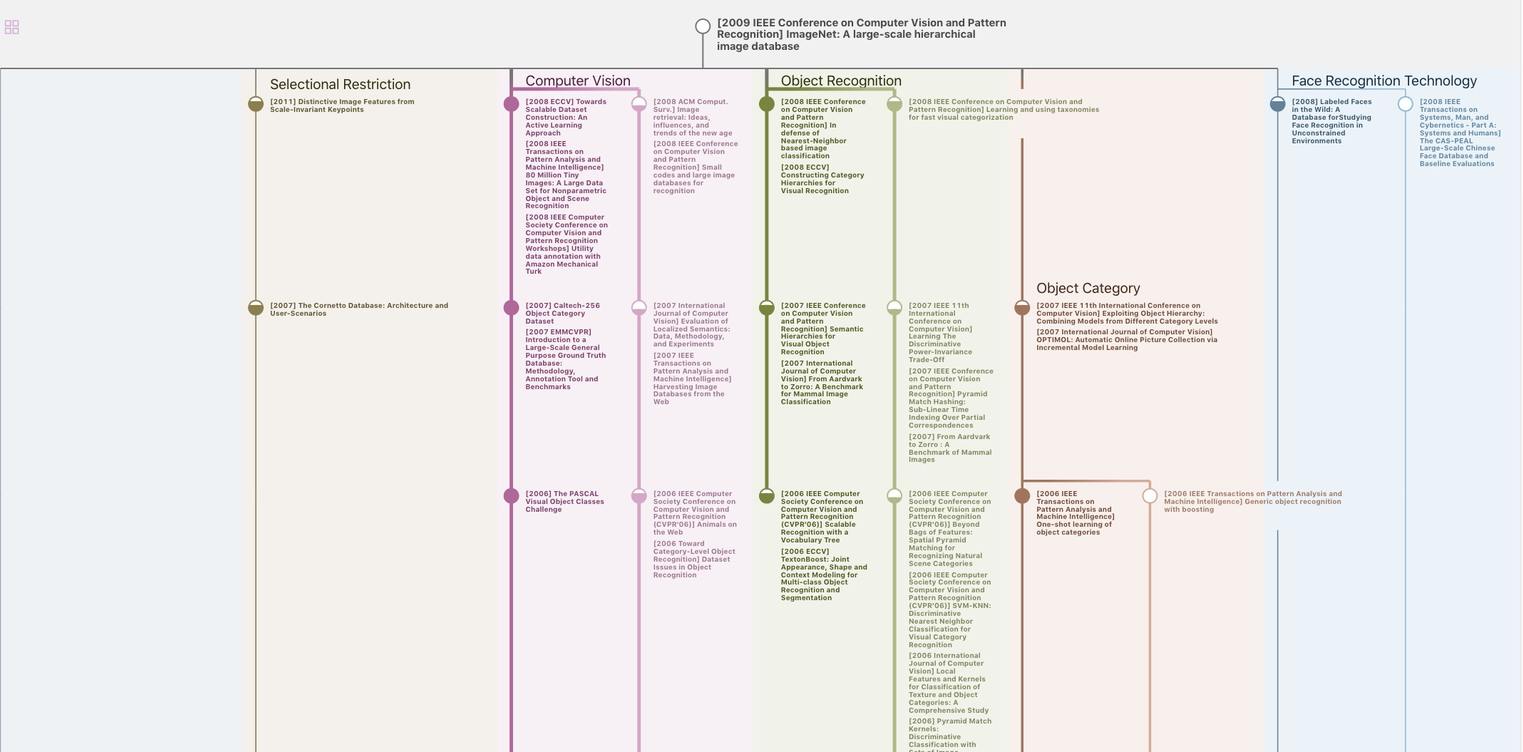
生成溯源树,研究论文发展脉络
Chat Paper
正在生成论文摘要