Deep Reinforcement Learning Module for Power Allocation in Optical Satellite Networks
2024 Panhellenic Conference on Electronics & Telecommunications (PACET)(2024)
Abstract
Optical satellite links and networks are currently being deployed by various space agencies and the industry for space, deep-space applications and intersatellite links. In this paper, a REINFORCE-based solution is presented for optimal power allocation regarding an optical geostationary satellite-to- ground system. Specifically, the proposed algorithm is channel model-free, and learns through a centralized stochastic neural network policy. The proposed allocation scheme is considered suitable for optical environments that are hard to model because it learns directly from experience. Experimental data from the ESA’s ARTEMIS optical satellite sessions are employed to evaluate the performance of the proposed algorithm. The achieved capacity outperforms the uniform power (UP) by 6% and exhibits good agreement with the model-based water-filling (WF) solution by 1.12%. The achieved channel allocated powers vary approximately 0.1 Watts from the WF ones, while the UP varies by 0.2-0.4 Watts.
MoreTranslated text
Key words
Artemis,deep reinforcement learning,free space optical,optical satellite,power allocation,reinforce
AI Read Science
Must-Reading Tree
Example
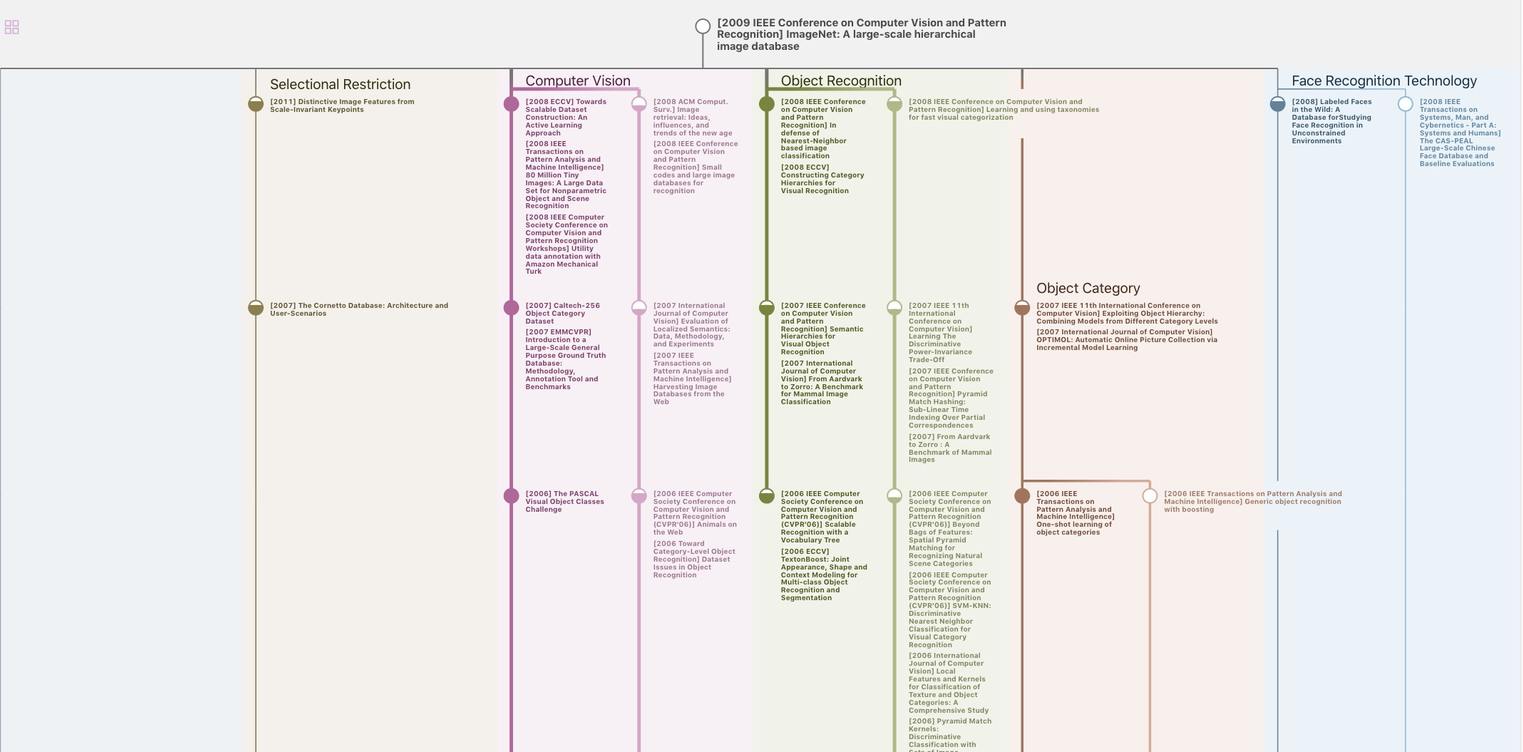
Generate MRT to find the research sequence of this paper
Chat Paper
Summary is being generated by the instructions you defined