Combining Deep Learning and Droplet Microfluidics for Rapid and Label-Free Antimicrobial Susceptibility Testing of Colistin
Biosensors and Bioelectronics(2024)
关键词
Antimicrobial resistance,Droplet microfluidics,Diagnostics,Bacteria detection,Bright field microscopy,Deep learning
AI 理解论文
溯源树
样例
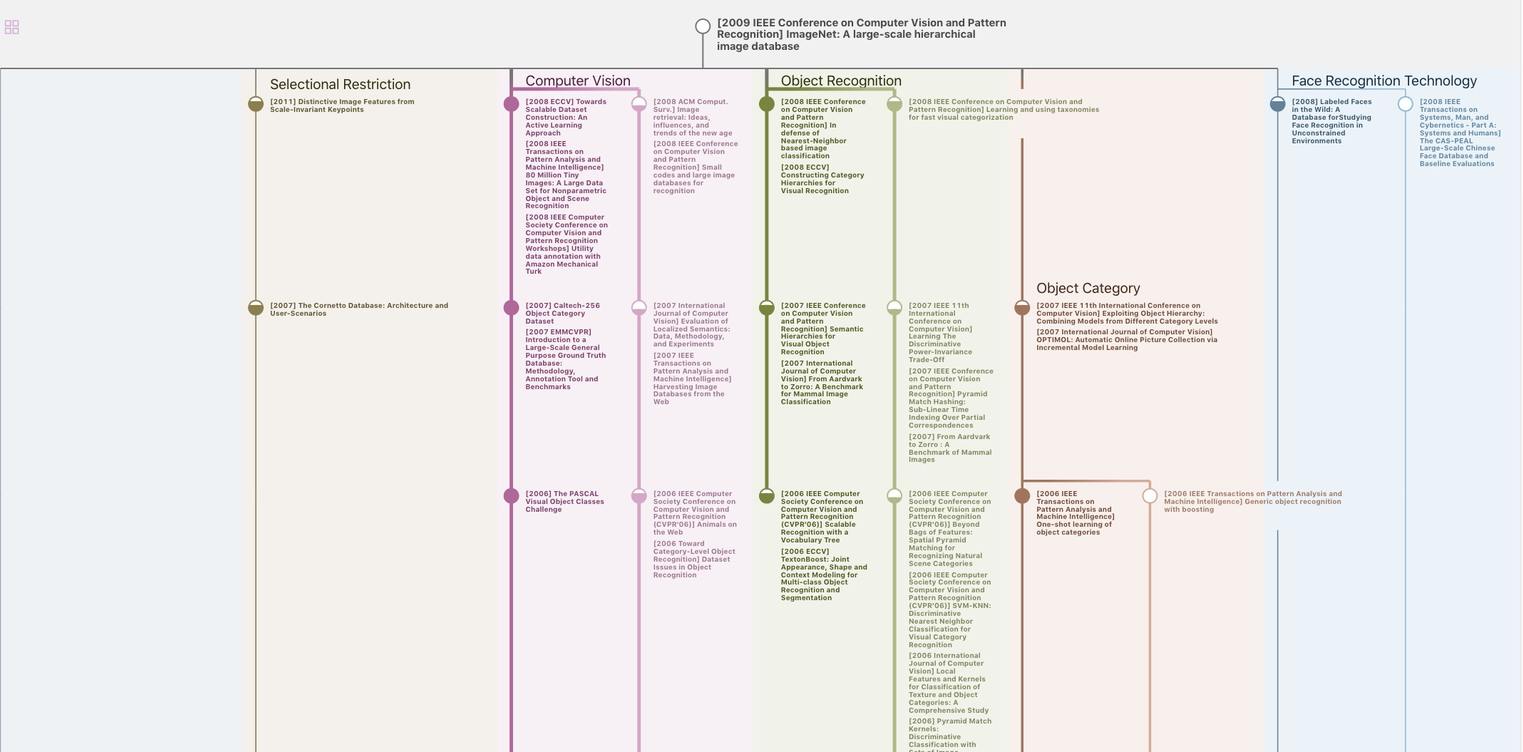
生成溯源树,研究论文发展脉络
Chat Paper
正在生成论文摘要