Application of the improved dung beetle optimizer, muti-head attention and hybrid deep learning algorithms to groundwater depth prediction in the Ningxia area, China
Atmospheric and Oceanic Science Letters(2024)
摘要
Due to the lack of accurate data and complex parameterization, the prediction of groundwater depth is a challenge for numerical models. Machine learning can effectively solve this issue and has been proven useful in the prediction of groundwater depth in many areas. In this study, two new models are applied to the prediction of groundwater depth in the Ningxia area, China. The two models combine the improved dung beetle optimizer (DBO) algorithm with two deep learning models: the Multi-head Attention–Convolution Neural Network–Long Short Term Memory networks (MH-CNN-LSTM) and the Multi-head Attention–Convolution Neural Network–Gated Recurrent Unit (MH-CNN-GRU). The models with DBO show better prediction performance, with larger R (correlation coefficient), RPD (residual prediction deviation), and lower RMSE (root-mean-square error). Compared with the models with the original DBO, the R and RPD of models with the improved DBO increase by over 1.5%, and the RMSE decreases by over 1.8%, indicating better prediction results. In addition, compared with the multiple linear regression model, a traditional statistical model, deep learning models have better prediction performance.
更多查看译文
关键词
Groundwater depth,Multi-head attention,Improved dung beetle optimizer,CNN-LSTM,CNN-GRU,Ningxia,地下水深度,多头注意力机制,改进的蜣螂优化算法,CNN-LSTM,CNN-GRU,宁夏
AI 理解论文
溯源树
样例
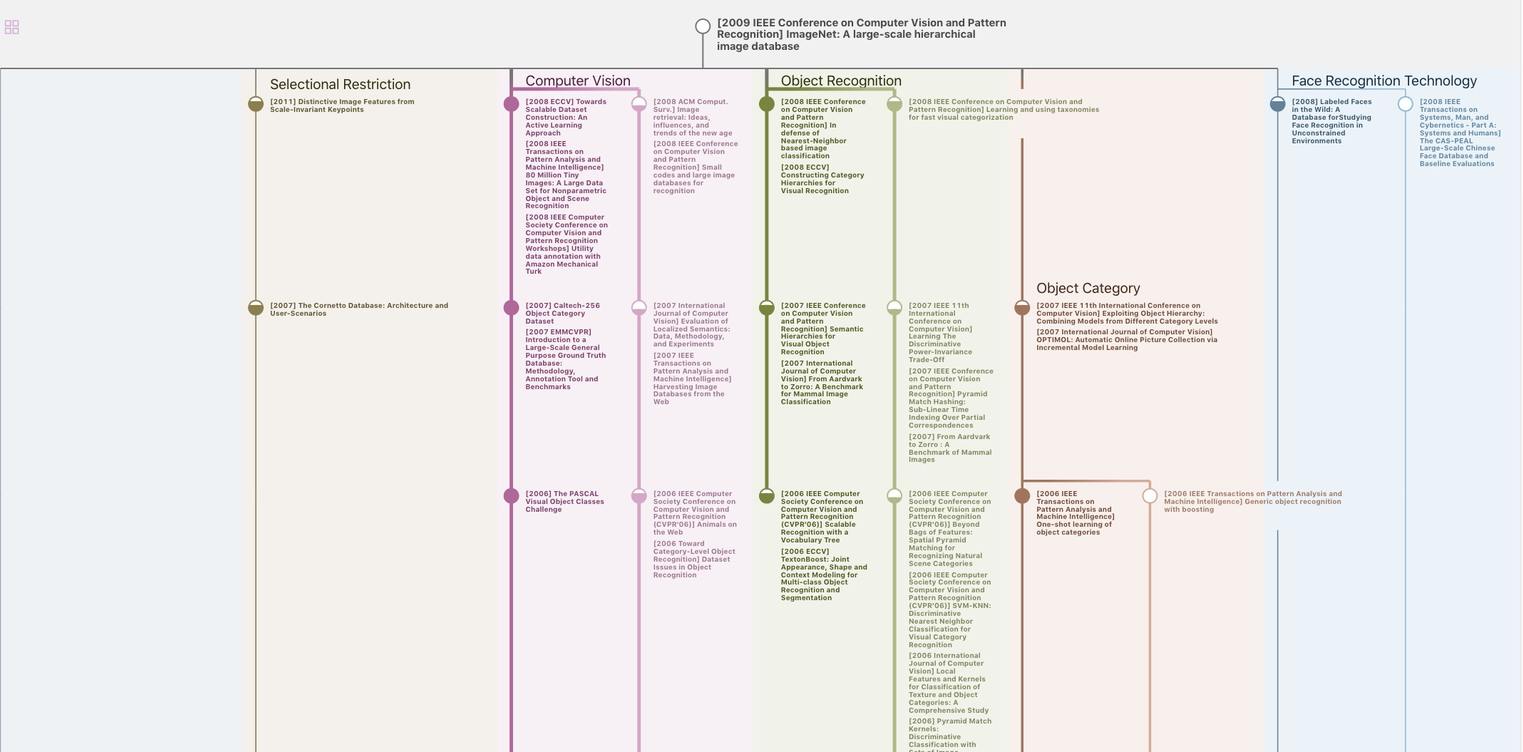
生成溯源树,研究论文发展脉络
Chat Paper
正在生成论文摘要