LGTrack: Exploiting Local and Global Properties for Robust Visual Tracking
IEEE Transactions on Circuits and Systems for Video Technology(2024)
摘要
Re-detection is a necessary capability for long-term tracking. Target candidate proposals in the whole image can provide a chance of tracking reset when tracking fails due to tracking drift or target invisibility. In this paper, we propose a unified local-global tracker based on the same transformer architecture sharing weights, which can not only search in a continuous local region but also provide target candidates of the global image in every frame. The requirements of both long-term and short-term scenarios can be addressed using a unified model. A simple proposal selection scheme is adopted to properly select the candidate proposals of re-detection, to assist tracking and obtain better performance. The scheme performs reevaluation of all high-quality proposals based on a transformer-based embedding network, once the predicted state of the local tracking is not sufficient to be accurate. To capture appearance variations brought by online updates in minimum risks, a long-term-friendly dynamic template update scheme is also designed. Extensive experiments are conducted to demonstrate the effectiveness of our proposed tracker, including three short-term tracking benchmarks and six long-term benchmarks. Our tracker can achieve results comparable to that of the state-of-the-art. The proposed tracker can also work well in balancing the performance and speed, achieving an average speed of approximately 25 fps tested on LaSOT testing set.
更多查看译文
关键词
object tracking,visual tracking,long-term tracking,re-detection
AI 理解论文
溯源树
样例
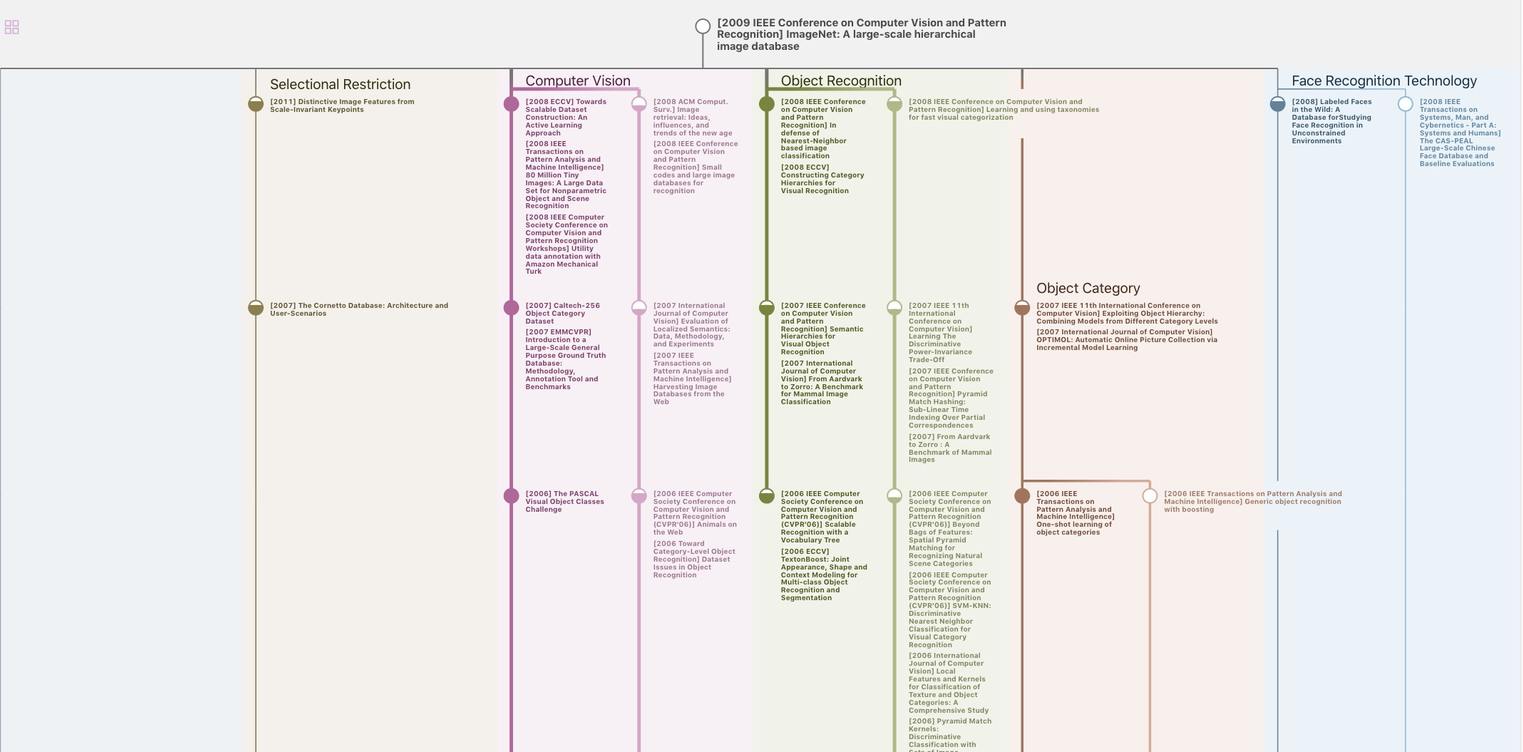
生成溯源树,研究论文发展脉络
Chat Paper
正在生成论文摘要