SSNet: Exploiting Spatial Information for Tobacco Stem Impurity Detection With Hyperspectral Imaging
IEEE ACCESS(2024)
Abstract
Tobacco stems are a critical component in cigarette production in China. However, accurately and promptly identifying impurities in tobacco stems remains a challenge with current deep learning methods, often failing to meet industry standards. This study introduces the Spectral-Spatial Network (SSNet), which is specifically optimized for rapid and precise detection of impurities in tobacco stems, leveraging spatial information in hyperspectral imaging. Designed to align with the high-speed requirements of industrial processing, SSNet effectively overcomes the complexities associated with analyzing hyperspectral data. The integration of spatial information particularly enhances recognition accuracy, especially in areas affected by fringe patterns. Our experiments reveal that SSNet significantly outperforms traditional methods in terms of speed and accuracy, marking a substantial advancement in the shift from manual to automated impurity detection in tobacco processing. Furthermore, SSNet's successful application highlights its potential for broader uses in various industrial image processing tasks.
MoreTranslated text
Key words
Spectral-spatial network,hyperspectral imaging,deep learning,computer vision,tobacco sorting
AI Read Science
Must-Reading Tree
Example
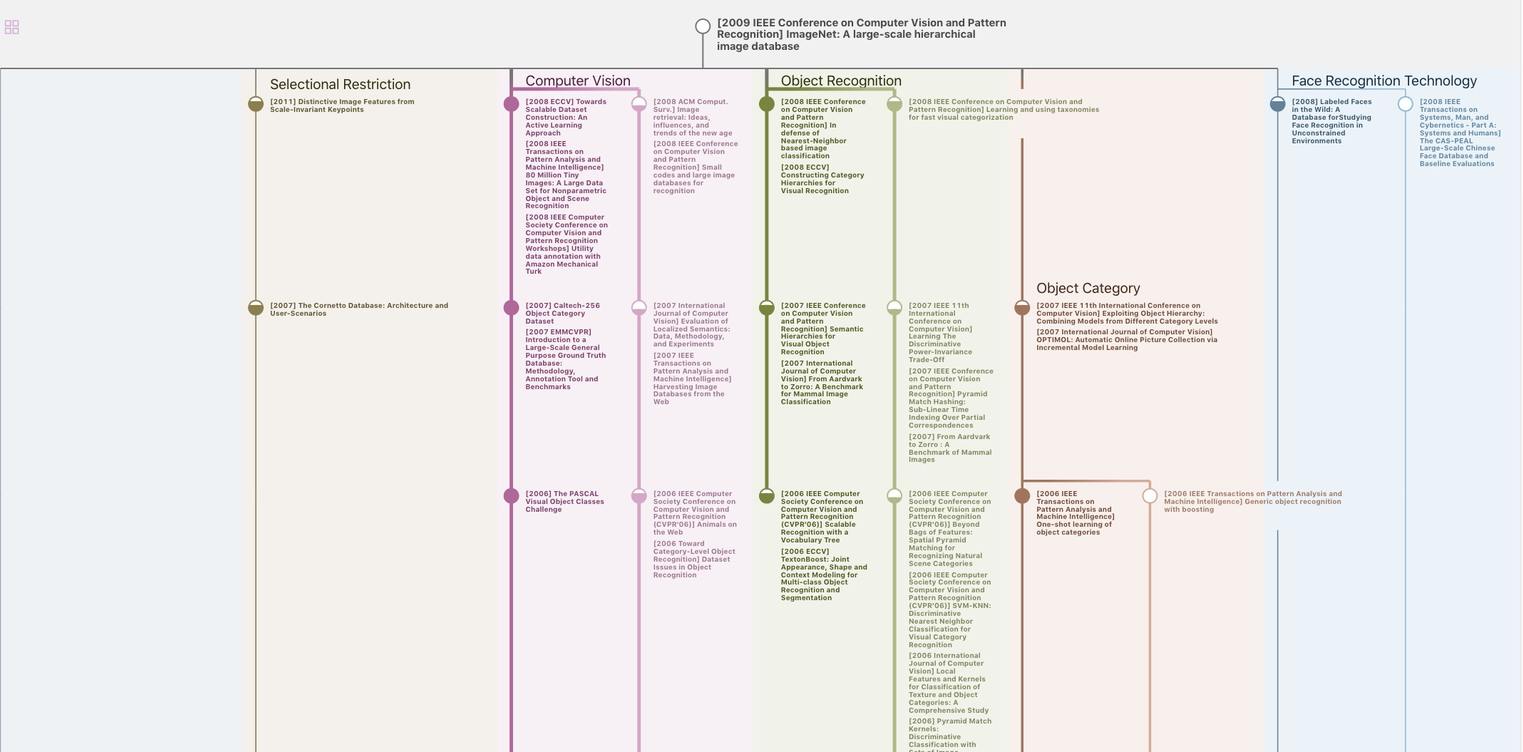
Generate MRT to find the research sequence of this paper
Chat Paper
Summary is being generated by the instructions you defined