Hierarchical Image Feature Compression for Machines via Feature Sparsity Learning
IEEE SIGNAL PROCESSING LETTERS(2024)
Abstract
Recently, Video Coding for Machines (VCM) has gained more and more attention due to its efforts in machine vision tasks. As a crucial track in VCM, feature compression preserves and transmits critical feature information for machine vision. Most existing studies employ dimensionality reduction to the raw multi-scale feature before compression. However, feature sparsity is left insufficiently considered in removing redundancy in compressed features. In this letter, we propose a novel framework for image feature compression for machines, where the multi-scale feature is hierarchically transformed into a sparse representation for compression. The multi-scale feature is first fused by convolutional neural networks and the attention mechanism. To introduce sparsity into the fused feature, informative channels are identified by a channel-wise binary mask where activated elements are sampled from the importance distribution of channels learned from feature content. Then, the fused feature is masked to generate a sparse representation for compression. Experiments conducted on two machine tasks show significant improvements in our model over state-of-the-art methods.
MoreTranslated text
Key words
Feature compression,learned image compression,sparsity learning,video coding for machines (VCM)
AI Read Science
Must-Reading Tree
Example
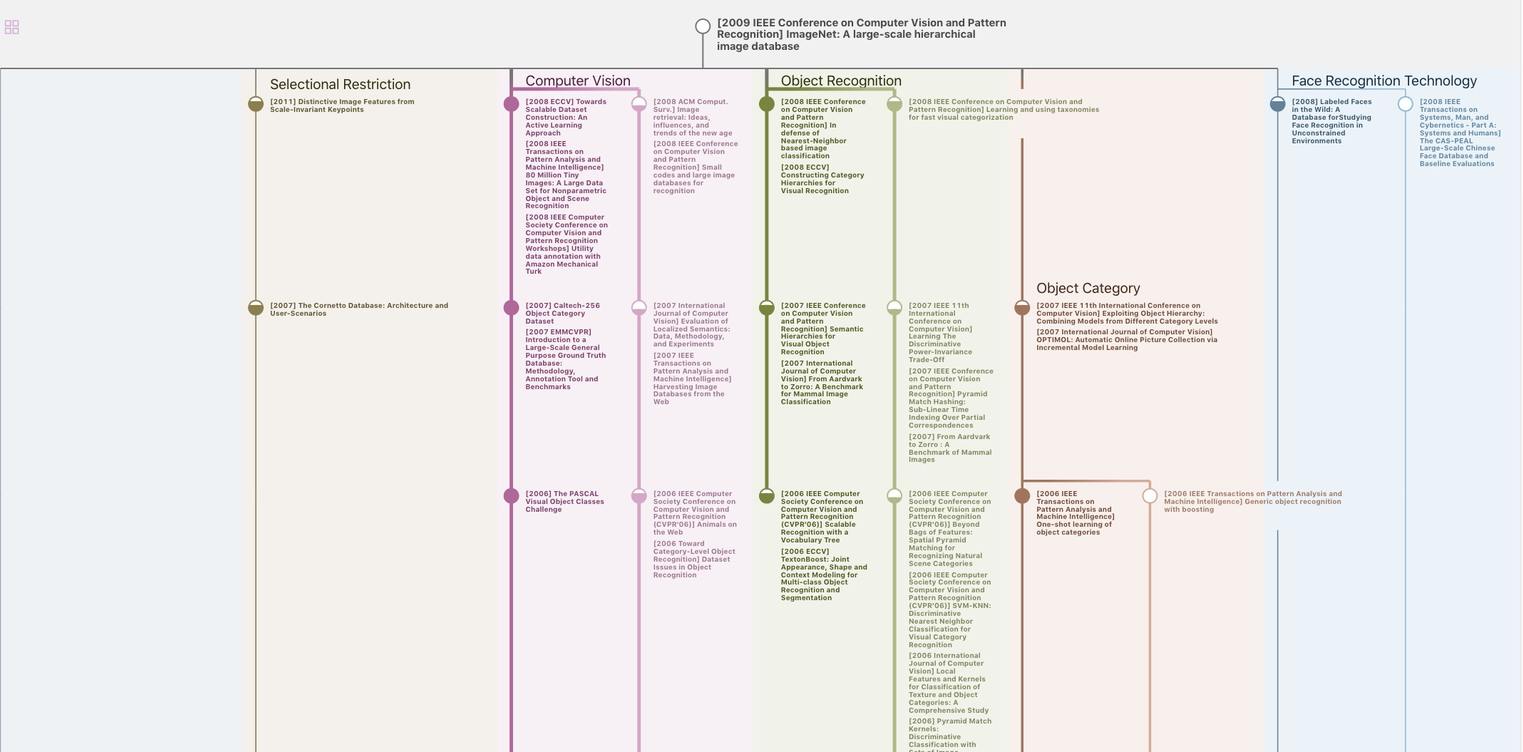
Generate MRT to find the research sequence of this paper
Chat Paper
Summary is being generated by the instructions you defined