Two system transformation data-driven algorithms for linear quadratic mean-field games
arxiv(2024)
摘要
This paper studies a class of continuous-time linear quadratic (LQ)
mean-field game problems. We develop two system transformation data-driven
algorithms to approximate the decentralized strategies of the LQ mean-field
games. The main feature of the obtained data-driven algorithms is that they
eliminate the requirement on all system matrices. First, we transform the
original stochastic system into an ordinary differential equation (ODE).
Subsequently, we construct some Kronecker product-based matrices by the
input/state data of the ODE. By virtue of these matrices, we implement a
model-based policy iteration (PI) algorithm and a model-based value iteration
(VI) algorithm in a data-driven fashion. In addition, we also demonstrate the
convergence of these two data-driven algorithms under some mild conditions.
Finally, we illustrate the practicality of our algorithms via two numerical
examples.
更多查看译文
AI 理解论文
溯源树
样例
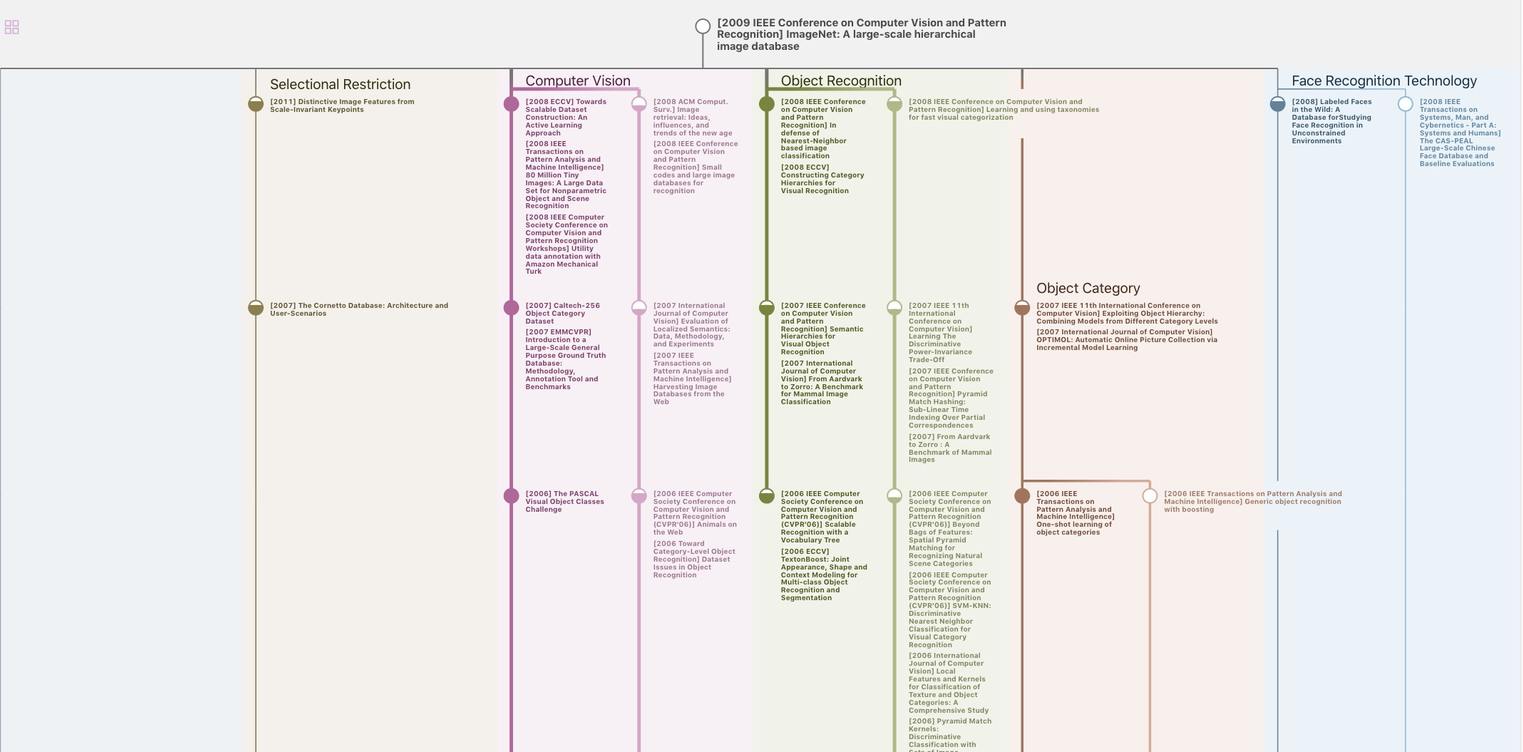
生成溯源树,研究论文发展脉络
Chat Paper
正在生成论文摘要