Improving Disturbance Estimation and Suppression via Learning Among Systems With Mismatched Dynamics
IEEE ROBOTICS AND AUTOMATION LETTERS(2024)
摘要
Iterative learning control (ILC) is a method for reducing system tracking or estimation errors over multiple iterations by using information from past iterations. The disturbance observer (DOB) is used to estimate and mitigate disturbances within the system, while the system is being affected by them. ILC enhances system performance by introducing a feedforward signal in each iteration. However, its effectiveness may diminish if the conditions change during the iterations. On the other hand, although DOB effectively mitigates the effects of new disturbances, it cannot entirely eliminate them as it operates reactively. Therefore, neither ILC nor DOB alone can ensure sufficient robustness in challenging scenarios. This study focuses on the simultaneous utilization of ILC and DOB to enhance system robustness. The proposed methodology specifically targets dynamically different linearized systems performing repetitive tasks. The systems share similar forms but differ in dynamics (e.g. sizes, masses, and controllers). Consequently, the design of learning filters must account for these differences in dynamics. To validate the approach, the study establishes a theoretical framework for designing learning filters in conjunction with DOB. The validity of the framework is then confirmed through numerical studies and experimental tests conducted on unmanned aerial vehicles (UAVs). Although UAVs are nonlinear systems, the study employs a linearized controller as they operate in proximity to the hover condition. A brief video introduction is included in the supplementary materials.
更多查看译文
关键词
Robust/adaptive control,aerial systems: mechanics and control,disturbance estimation,iterative learning
AI 理解论文
溯源树
样例
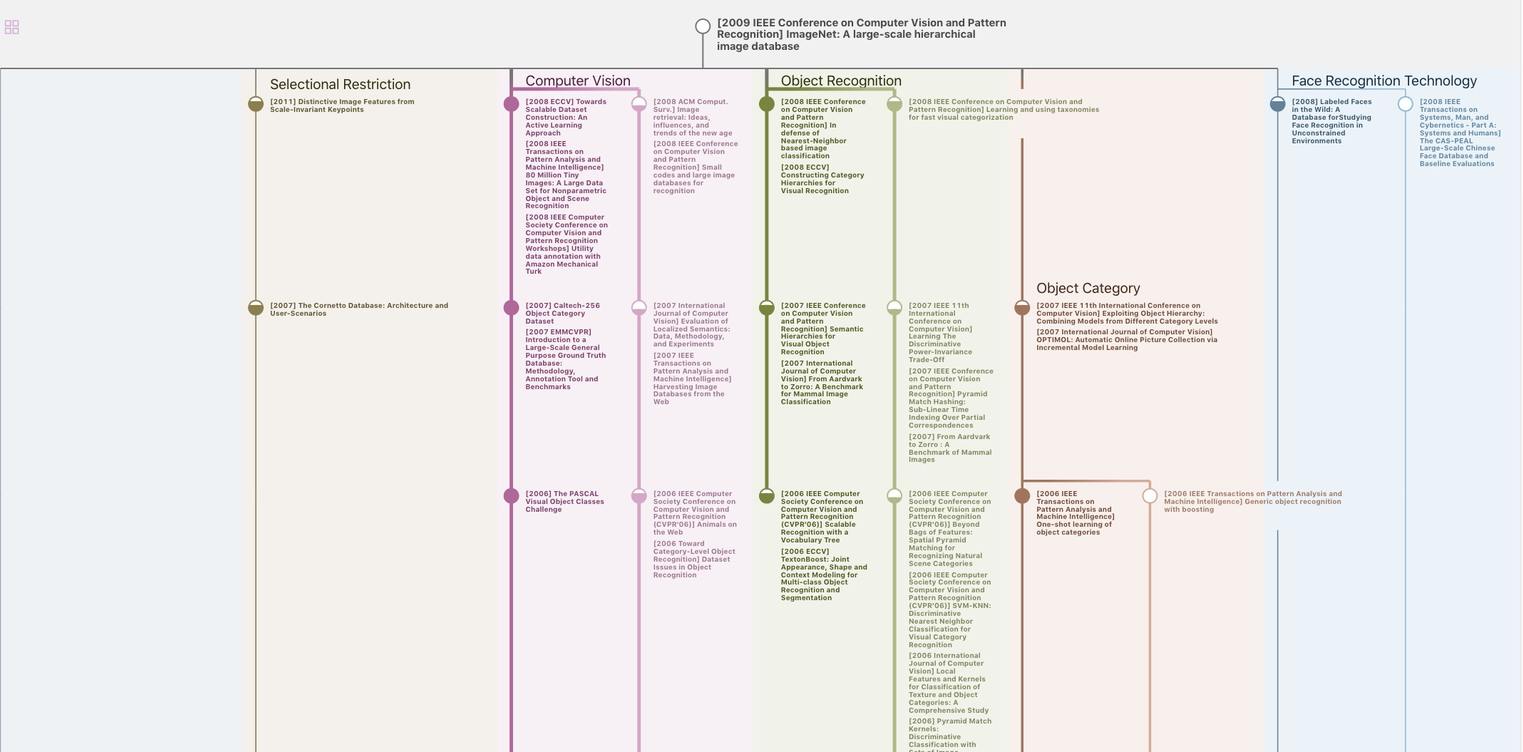
生成溯源树,研究论文发展脉络
Chat Paper
正在生成论文摘要