SDAC-DA: Semi-Supervised Deep Attributed Clustering Using Dual Autoencoder
IEEE Transactions on Knowledge and Data Engineering(2024)
Abstract
Attributed graph clustering aims to group nodes into disjoint categories using deep learning to represent node embeddings and has shown promising performance across various applications. However, two main challenges hinder further performance improvement. Firstly, reliance on unsupervised methods impedes the learning of low-dimensional, clustering-specific features in the representation layer, thus impacting clustering performance. Secondly, the predominant use of separate approaches leads to suboptimal learned embeddings that are insufficient for subsequent clustering steps. To address these limitations, we propose a novel method called Semi-supervised Deep Attributed Clustering using Dual Autoencoder (SDAC-DA). This approach enables semi-supervised deep end-to-end clustering in attributed networks, promoting high structural cohesiveness and attribute homogeneity. SDAC-DA transforms the attribute network into a dual-view network, applies a semi-supervised autoencoder layering approach to each view, and integrates dimensionality reduction matrices by considering complementary views. The resulting representation layer contains high clustering-friendly embeddings, which are optimized through a unified end-to-end clustering process for effectively identifying clusters. Extensive experiments on both synthetic and real networks demonstrate the superiority of our proposed method over seven state-of-the-art approaches.
MoreTranslated text
Key words
Attributed network,deep attributed clustering,pairwise constraints,semi-supervised clustering
AI Read Science
Must-Reading Tree
Example
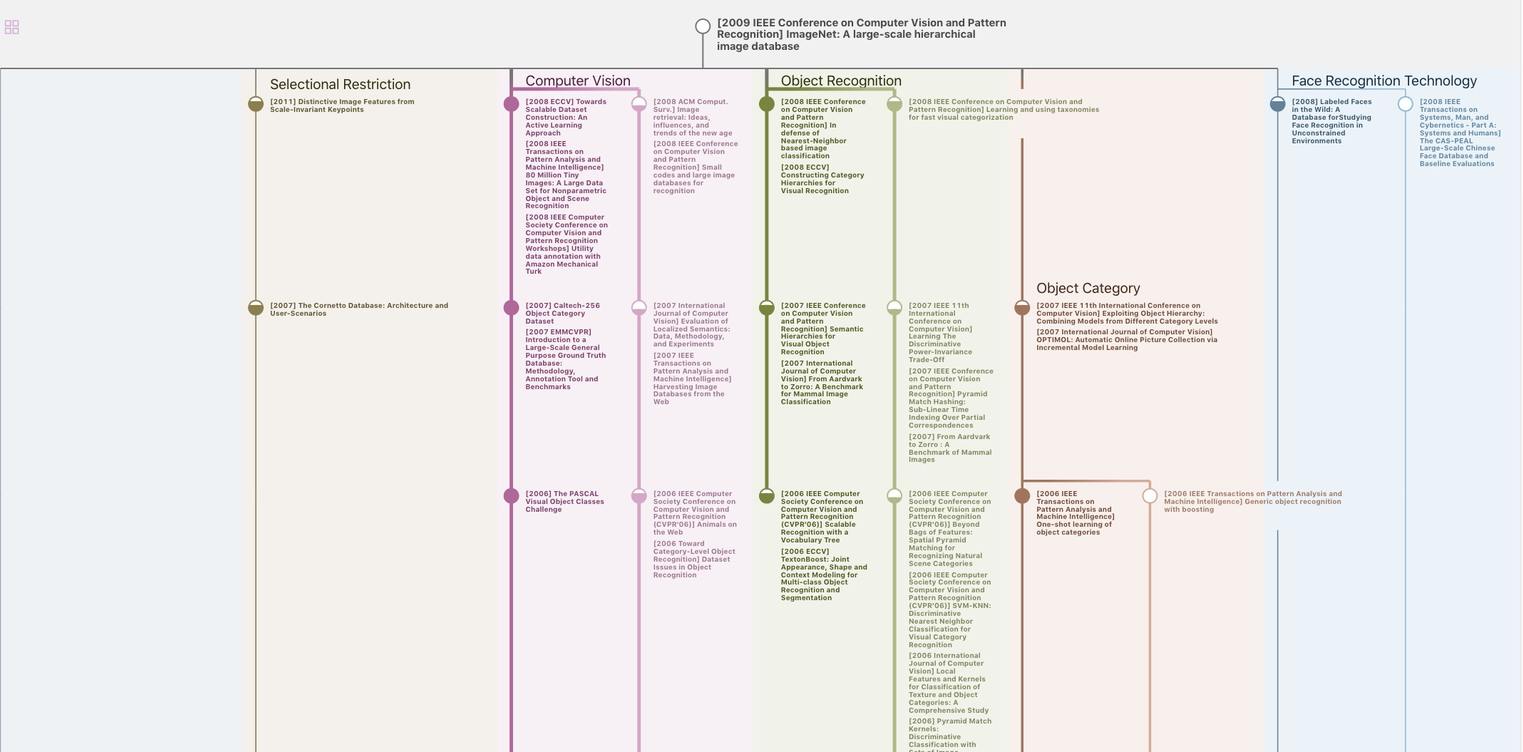
Generate MRT to find the research sequence of this paper
Chat Paper
Summary is being generated by the instructions you defined