A Novel Fault Diagnosis Based on FFT-1DCNN
2023 7th International Conference on Electrical, Mechanical and Computer Engineering (ICEMCE)(2023)
摘要
Intelligent fault diagnosis of rotating machinery has been an important topic in mechanical fault research. Due to the difficulty of manual diagnosis in providing timely and accurate results, convolutional neural networks (CNNs) have been widely used for fault diagnosis. However, some existing methods directly process time-domain signals using artificial neural networks, lacking analysis of frequency domain information, leading to limited accuracy. Others extract frequency domain information but result in complex networks with numerous parameters, requiring extensive training time and insufficient diagnosis speed. In this paper, we propose an intelligent diagnostic approach based on Fast Fourier Transform (FFT) and one-dimensional Convolutional Neural Network (1DCNN). We validate the ef- fectiveness of this approach using the Case Western Reserve University's rolling bearing dataset, achieving diagnostic accu- racy of over 99% for different types and severities of damage datasets. Moreover, the proposed method achieves a diagnosis speed of 115ms, demonstrating higher accuracy and efficiency compared to existing diagnostic methods and enabling real-time fault diagnosis of bearings.
更多查看译文
关键词
Rolling Bearing,Fault Diagnosis,One-Dimensional Convolutional Neural Network,Fast Fourier transform
AI 理解论文
溯源树
样例
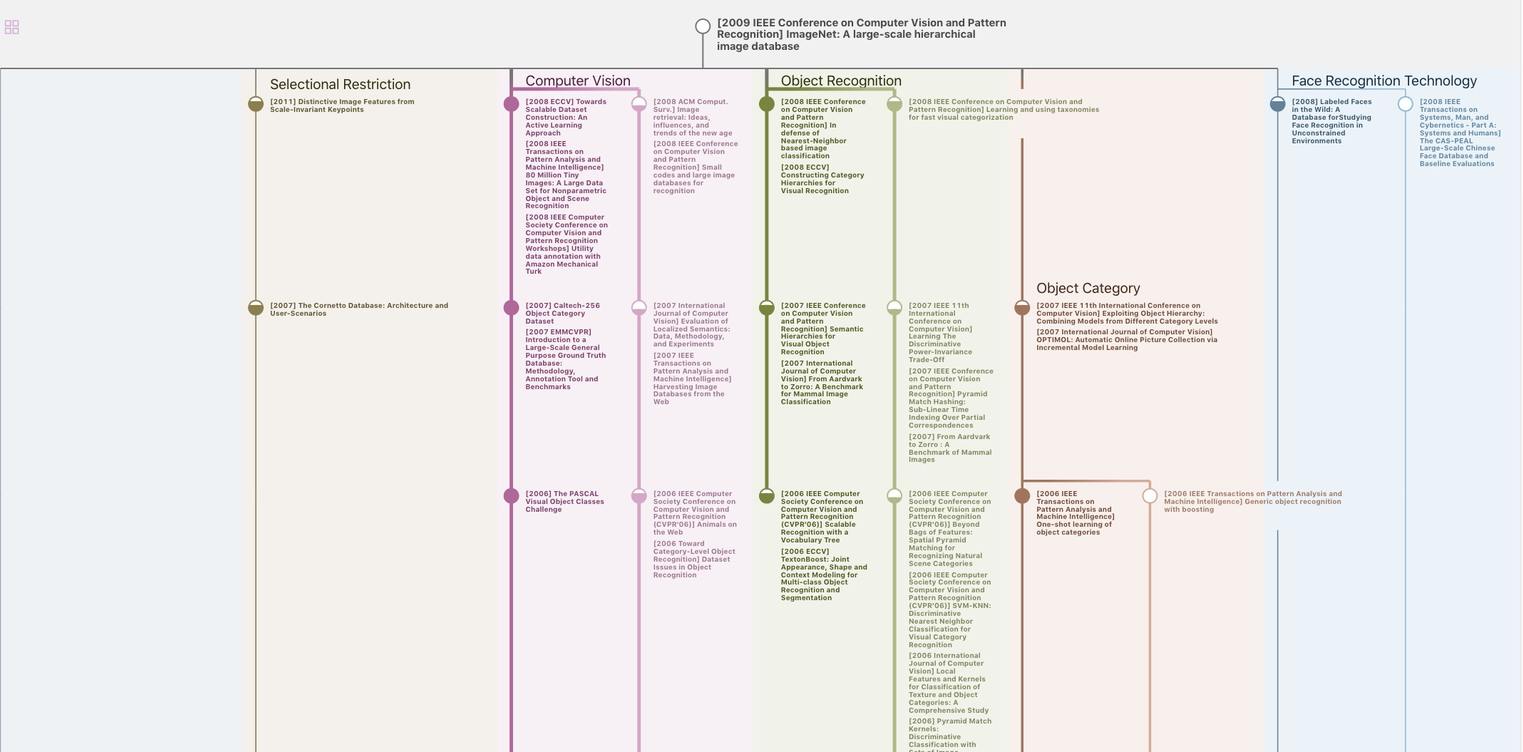
生成溯源树,研究论文发展脉络
Chat Paper
正在生成论文摘要