Comparing Accuracy of Wildfire Spread Prediction Models under Different Data Deficiency Conditions
Fire(2024)
摘要
Wildfire is one of the most severe natural disasters globally, profoundly affecting natural ecology, economy, and health and safety. Precisely predicting the spread of wildfires has become an important research topic. Current fire spread prediction models depend on inputs from a variety of geographical and environmental variables. However, unlike the ideal conditions simulated in the laboratory, data gaps often occur in real wildfire scenarios, posing challenges to the accuracy and robustness of predictions. It is necessary to explore the extent to which different missing items affect prediction accuracy, thereby providing rational suggestions for emergency decision-making. In this paper, we tested how different conditions of missing data affect the prediction accuracy of existing wildfire spread models and quantified the corresponding errors. The final experimental results suggest that it is necessary to judge the potential impact of data gaps based on the geographical conditions of the study area appropriately, as there is no significant pattern of behavior yet identified. This study aims to simulate the impact of data scarcity on the accuracy of wildfire spread prediction models in real scenarios, thereby enabling researchers to better understand the priority of different environmental variables for the model and identify the acceptable degree of missing data and the indispensable data attributes. It offers new insights for developing spread prediction models applicable to real-world scenarios and rational assessment of the effectiveness of model outcomes.
更多查看译文
关键词
wildfire spread prediction model,data gap,robustness test,uncertainty analysis,evaluation metrics
AI 理解论文
溯源树
样例
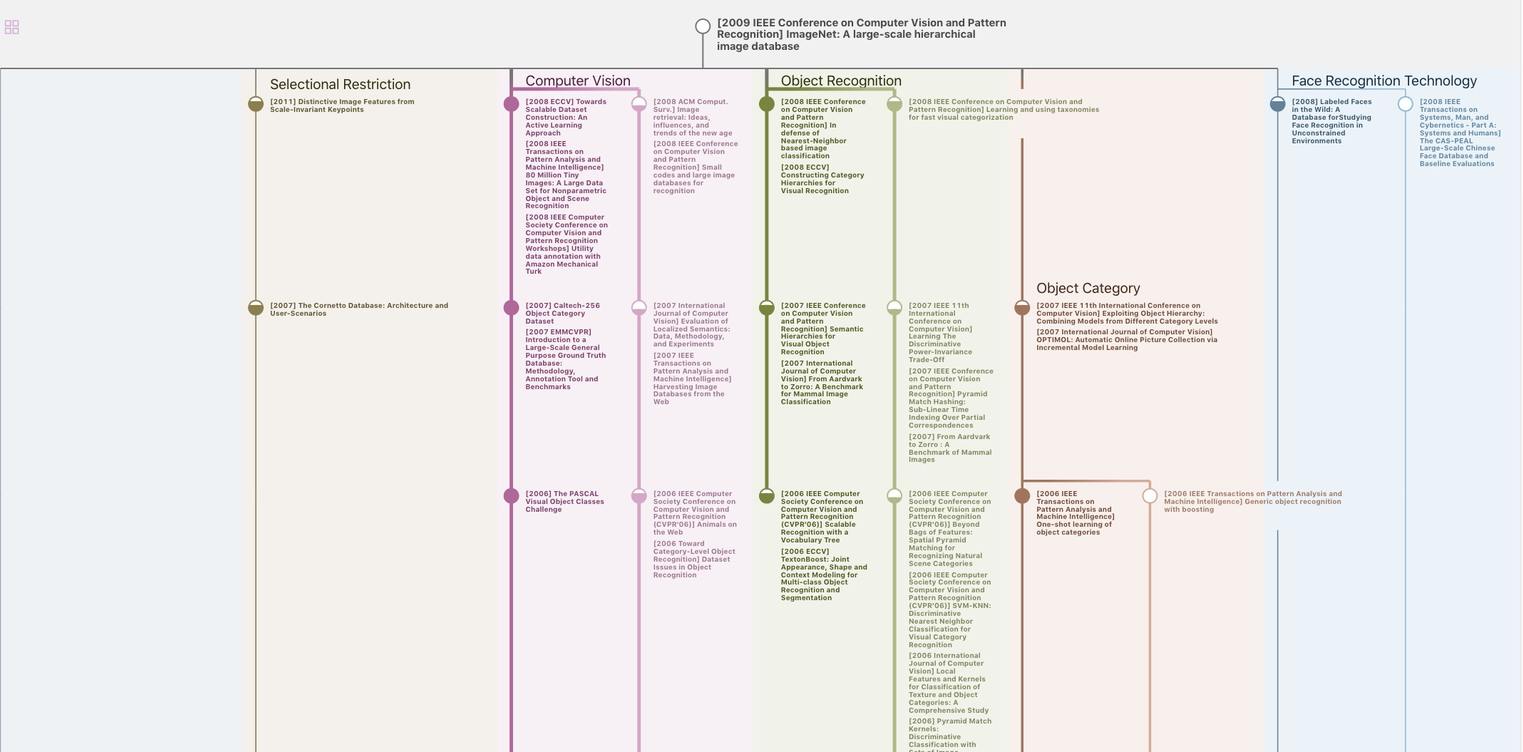
生成溯源树,研究论文发展脉络
Chat Paper
正在生成论文摘要