M2BIST-SPNet: RUL prediction for railway signaling electromechanical devices
The Journal of Supercomputing(2024)
摘要
Railway signaling electromechanical devices (RSEDs) play a pivotal role in the railway industry. Normal wear and tear of these devices occur during day-and-night operation and even progressively develop into failures. Hence, it is imperative to predict the remaining useful life (RUL) for reliable services. However, there are three existing challenges in addressing the issue. To overcome these challenges, we introduce M ^2 BIST-SPNet for RSEDs RUL prediction. The model incorporates advanced spatiotemporal feature extraction mechanisms, including a spatial-temporal attention-based convolution networks (STACN), multiple branches with multi-scale multifrequency module (MBM), and communication module (CM). Extensive experiments conducted on three typical object datasets (i.e., railway safety relay, EMU contactor, switch circuit controller) shows that the proposed approach has demonstrated state-of-the-art performance in long-term multiparameter prediction and RUL prediction.
更多查看译文
关键词
RUL,Long-term prediction,Railway signaling electromechanical device,Spatial-temporal attention-based convolution network,Multi-scale multifrequency module,Communication module
AI 理解论文
溯源树
样例
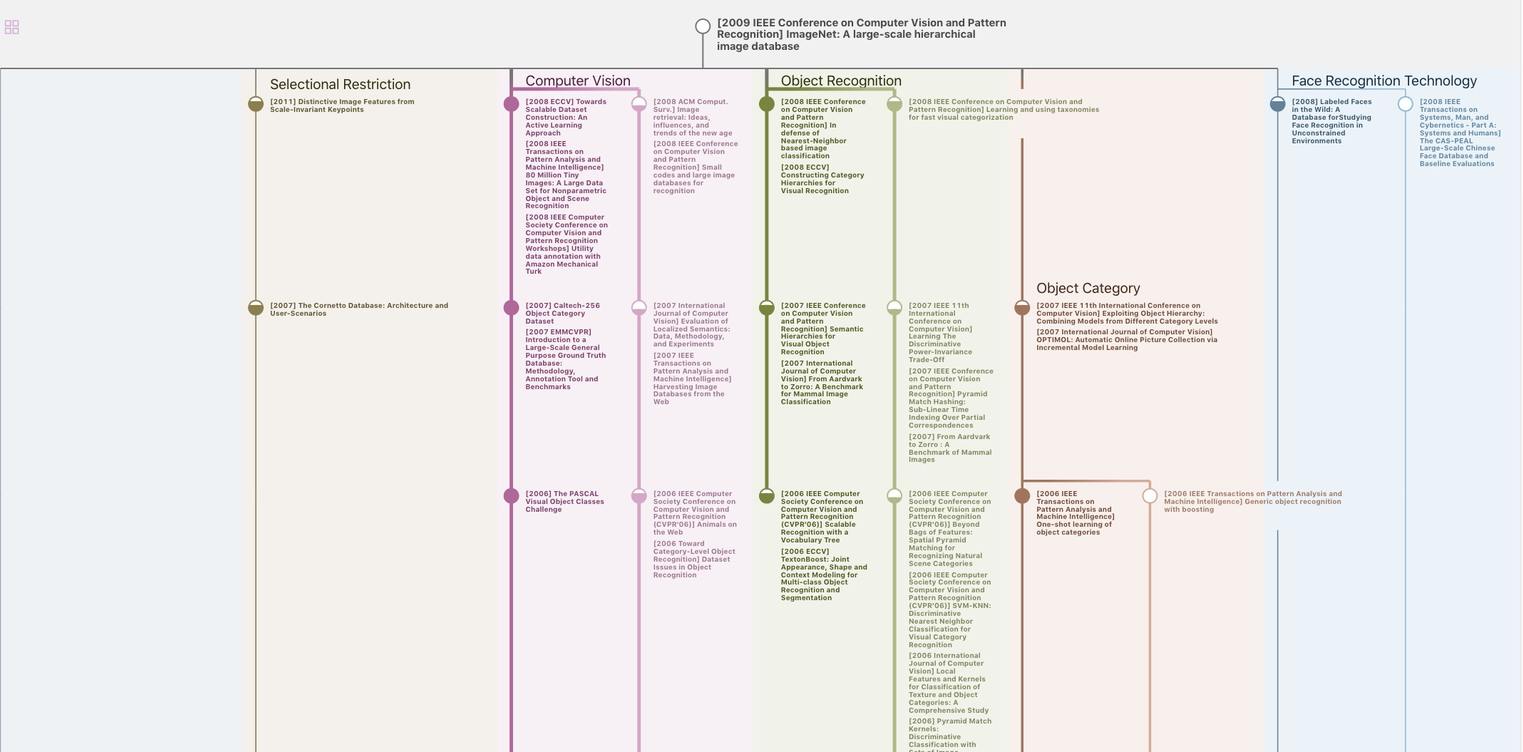
生成溯源树,研究论文发展脉络
Chat Paper
正在生成论文摘要