An optimised YOLOv4 deep learning model for efficient malarial cell detection in thin blood smear images
Parasites & Vectors(2024)
摘要
Malaria is a serious public health concern worldwide. Early and accurate diagnosis is essential for controlling the disease’s spread and avoiding severe health complications. Manual examination of blood smear samples by skilled technicians is a time-consuming aspect of the conventional malaria diagnosis toolbox. Malaria persists in many parts of the world, emphasising the urgent need for sophisticated and automated diagnostic instruments to expedite the identification of infected cells, thereby facilitating timely treatment and reducing the risk of disease transmission. This study aims to introduce a more lightweight and quicker model—but with improved accuracy—for diagnosing malaria using a YOLOv4 (You Only Look Once v. 4) deep learning object detector. The YOLOv4 model is modified using direct layer pruning and backbone replacement. The primary objective of layer pruning is the removal and individual analysis of residual blocks within the C3, C4 and C5 (C3–C5) Res-block bodies of the backbone architecture’s C3-C5 Res-block bodies. The CSP-DarkNet53 backbone is simultaneously replaced for enhanced feature extraction with a shallower ResNet50 network. The performance metrics of the models are compared and analysed. The modified models outperform the original YOLOv4 model. The YOLOv4-RC3_4 model with residual blocks pruned from the C3 and C4 Res-block body achieves the highest mean accuracy precision (mAP) of 90.70
更多查看译文
关键词
Malaria,YOLOv4,Optimised,Residual network,Residual block,Object detection
AI 理解论文
溯源树
样例
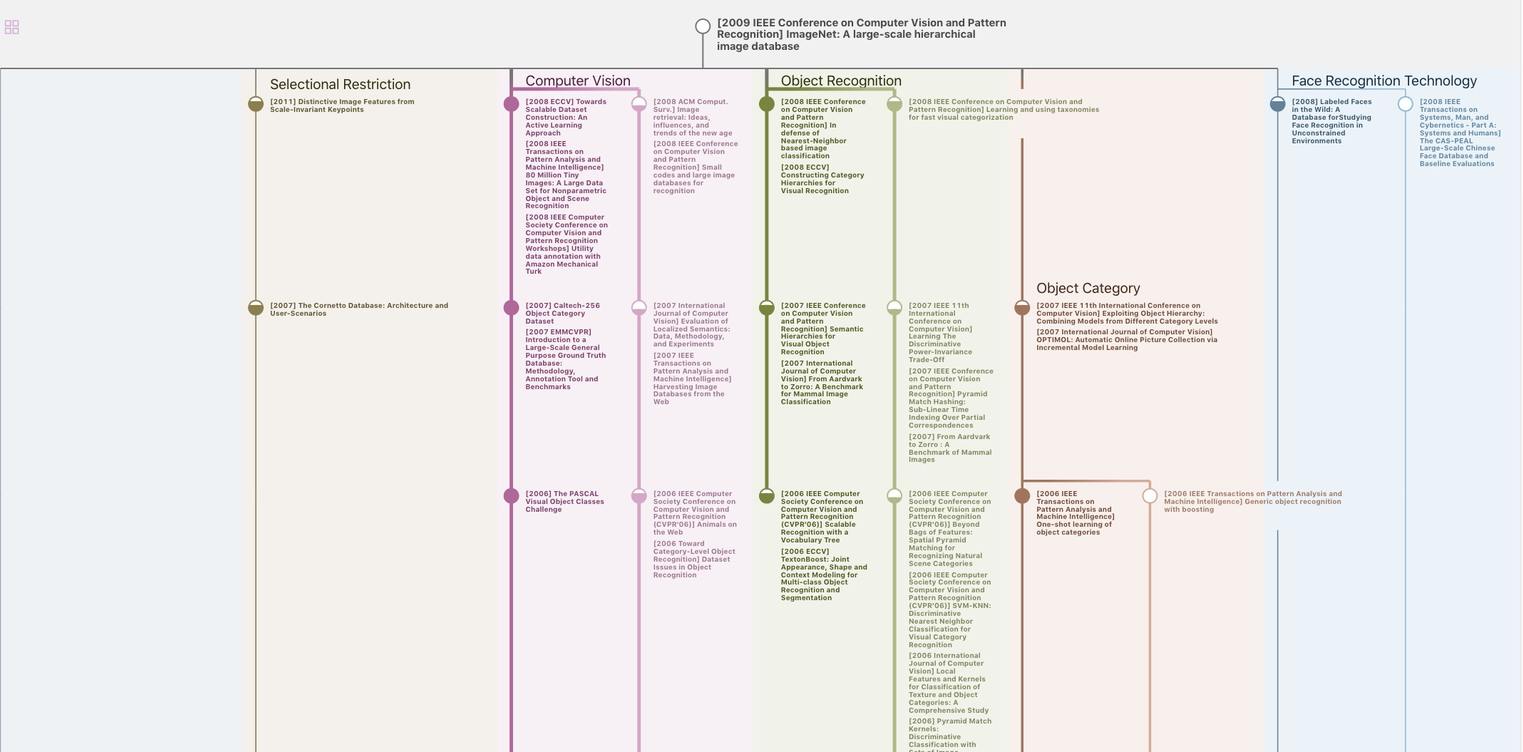
生成溯源树,研究论文发展脉络
Chat Paper
正在生成论文摘要