Can Deep Learning Search for Exceptional Chiroptical Properties? The Halogenated [6]Helicene Case
crossref(2024)
摘要
In this work we predict, among more than a billion possibilities, the best candidates of halogenated [6]helicenes in order to obtain excellent chiroptical properties in terms of the rotatory strength (R). We have used DFT calculations to randomly create derivatives from 1 to 16 halogen atoms, that were then used as a data set to train different deep neural network models. It is worth noting that the simplest model affords a parametrization that allows to easily predict the value of R for any hexahalogenated [6]helicene. The correlation between calculated and predicted data increases together with the complexity of the model. The results show that some positions and halogens are preferred to increase the R value. In this sense, we have also synthesized the derivatives with the higher predicted R, obtaining excellent correlation among the values obtained experimentally, by DFT-calculations and machine learning predictions.
更多查看译文
AI 理解论文
溯源树
样例
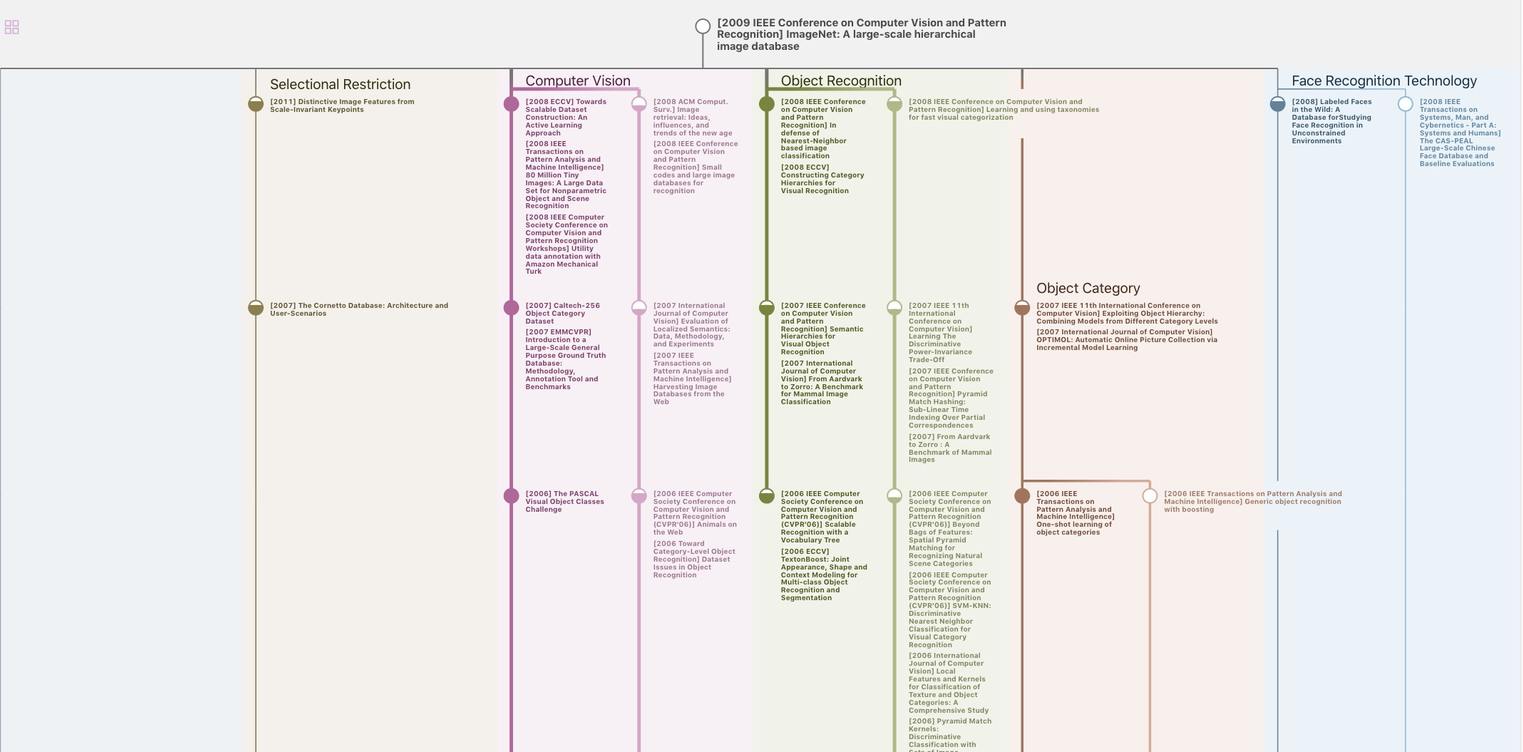
生成溯源树,研究论文发展脉络
Chat Paper
正在生成论文摘要