Evaluation of CNN Models with Transfer Learning in Art Media Classification in Terms of Accuracy and Class Relationship
COMPUTACION Y SISTEMAS(2024)
摘要
The accuracy obtained in Art Media Classification (AMC) using CNN is lower compared to other image classification problems, where the acceptable accuracy ranges from 90 to 99%. This article presents an analysis of the performance of three different CNNs with transfer learning for AMC, to answer the question of what challenges arise in this application. We proposed the Art Media Dataset (ArtMD) to train three CNNs. ArtMD contains five classes of art: Drawing, Engraving, Iconography, Painting, and Sculpture. The analysis of the results demonstrates that all the tested CNNs exhibit similar behavior. Drawing, Engraving, and Painting had the highest relationship, showing a strong relationship between Drawing and Engraving. We implemented two more experiments, removing first Drawing and then Engraving. The best performance with 86% accuracy was achieved by removing Drawing. Analysis of the confusion matrix of the three experiments for each CNN confirms that Drawing and Painting have the lowest accuracy, showing a strong misclassification with the other classes. This analysis presents the degree of relationship between the three CNN models and details the challenges of AMC.
更多查看译文
关键词
Art media classification,convolutional neural networks,transfer learning
AI 理解论文
溯源树
样例
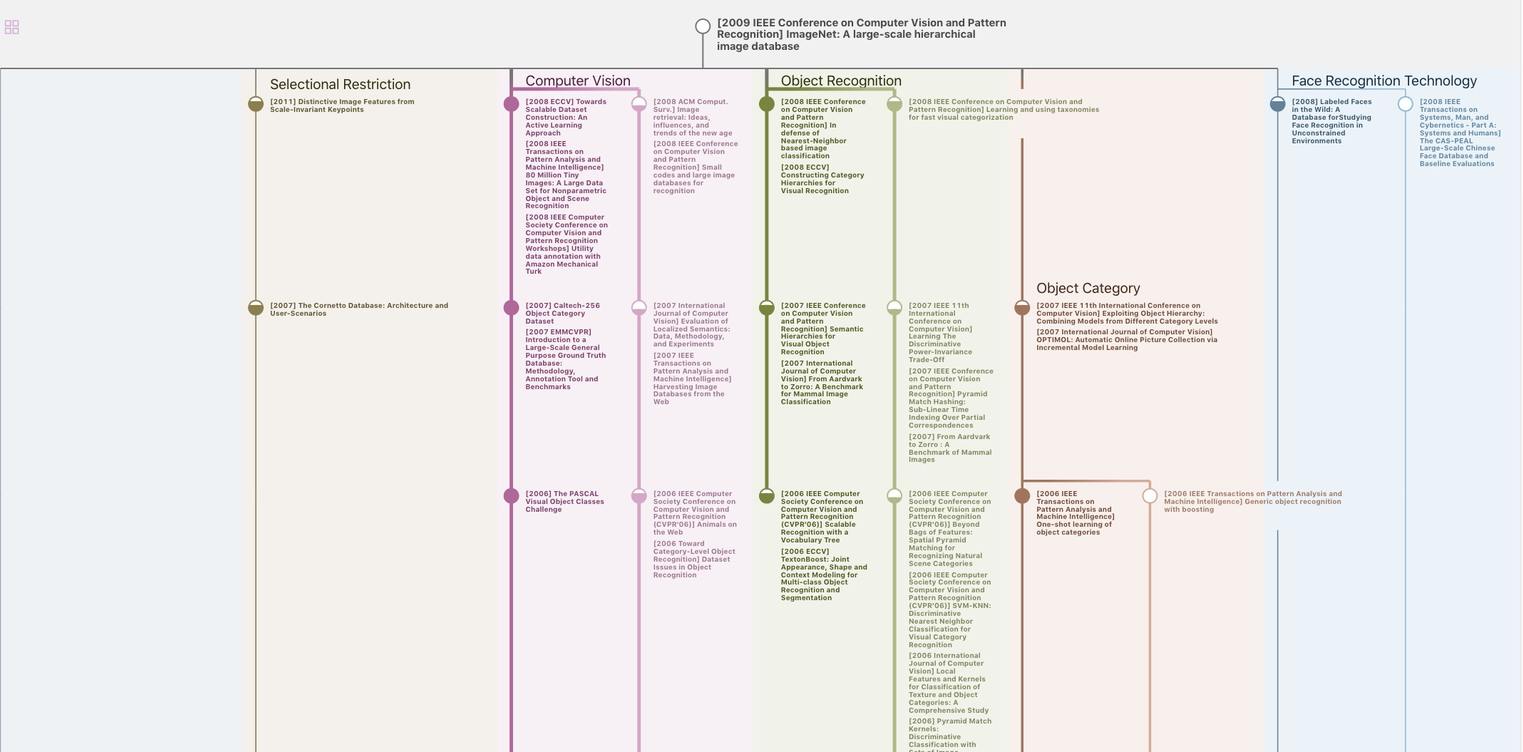
生成溯源树,研究论文发展脉络
Chat Paper
正在生成论文摘要