A Comprehensive Interaction in Multiscale Multichannel EEG Signals for Emotion Recognition
MATHEMATICS(2024)
Abstract
Electroencephalogram (EEG) is the most preferred and credible source for emotion recognition, where long-short range features and a multichannel relationship are crucial for performance because numerous physiological components function at various time scales and on different channels. We propose a cascade scale-aware adaptive graph convolutional network and cross-EEG transformer (SAG-CET) to explore the comprehensive interaction between multiscale and multichannel EEG signals with two novel ideas. First, to model the relationship of multichannel EEG signals and enhance signal representation ability, the multiscale EEG signals are fed into a scale-aware adaptive graph convolutional network (SAG) before the CET model. Second, the cross-EEG transformer (CET), is used to explicitly capture multiscale features as well as their correlations. The CET consists of two self-attention encoders for gathering features from long-short time series and a cross-attention module to integrate multiscale class tokens. Our experiments show that CET significantly outperforms a vanilla unitary transformer, and the SAG module brings visible gains. Our methods also outperform state-of-the-art methods in subject-dependent tasks with 98.89%/98.92% in accuracy for valence/arousal on DEAP and 99.08%/99.21% on DREAMER.
MoreTranslated text
Key words
EEG classification,emotion recognition,multiscale feature,cross attention
AI Read Science
Must-Reading Tree
Example
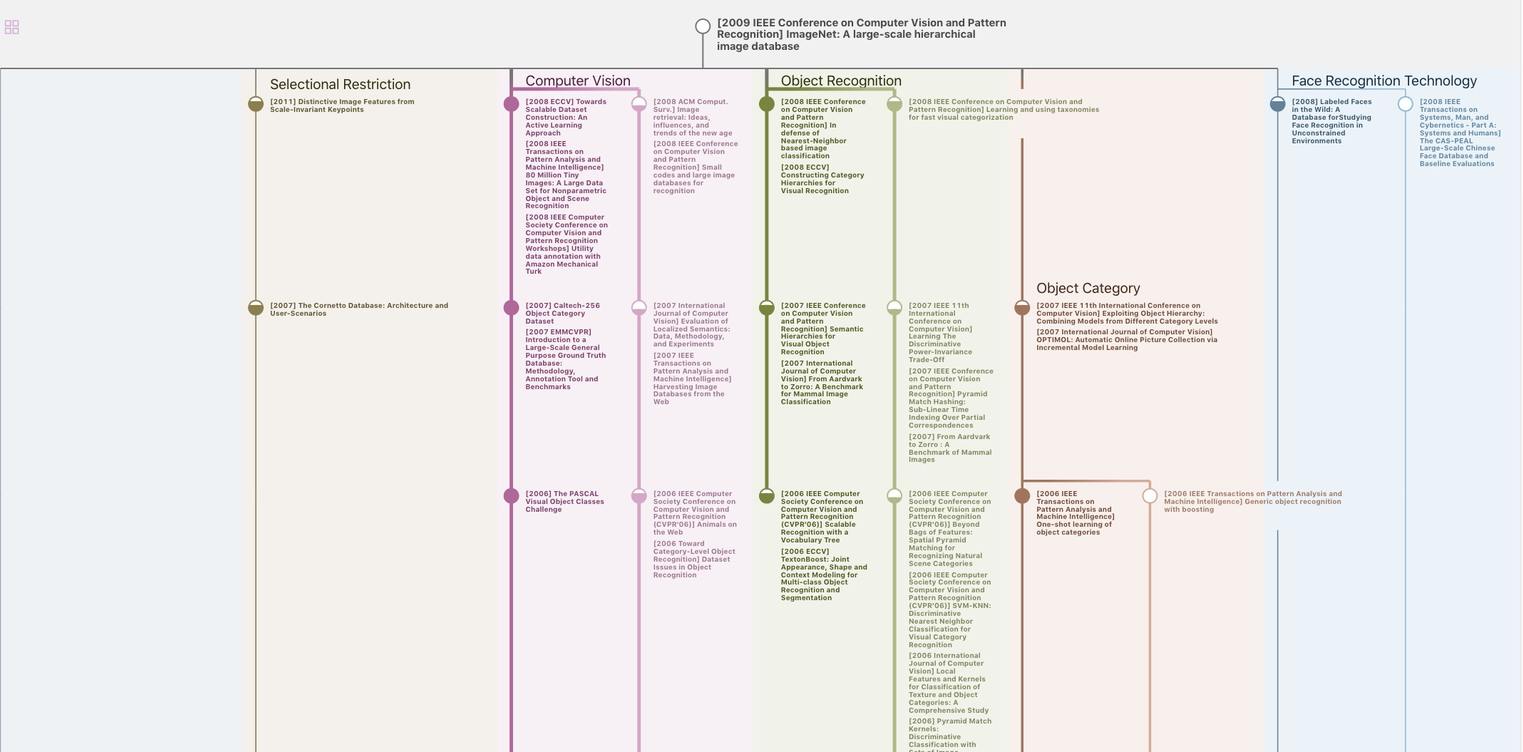
Generate MRT to find the research sequence of this paper
Chat Paper
Summary is being generated by the instructions you defined