Physics-Informed deep Autoencoder for fault detection in New-Design systems
Mechanical Systems and Signal Processing(2024)
Abstract
The industrial application of data-driven methods for fault detection of new-design systems is limited by the inevitable scarcity of real data. Physics-Informed Neural Networks (PINNs) can mitigate this problem by integrating data and physical knowledge. In this work, we develop a novel fault detection method that combines physics-based simulations for data generation with a Physics-Informed Deep Autoencoder (PIDAE) for reproducing the system behaviour in normal conditions; the Sequential Probability Ratio Test (SPRT) is, then, used for detecting abnormal conditions. The proposed method is applied to new-design electro-hydraulic servo actuators used in turbofan engine fuel systems. The results show that it can provide more satisfactory fault detection performance, in terms of false and missed alarms, than state-of-the-art methods based on traditional autoencoders only and pure physics-based models only. Furthermore, the PIDAE outcomes are physically consistent and, therefore, more acceptable and trustworthy.
MoreTranslated text
Key words
Prognostics and health management,Fault detection,Physics-informed neural network (PINN),Autoencoder,Electro-Hydraulic Servo Actuator (EHSA),Turbofan engine
AI Read Science
Must-Reading Tree
Example
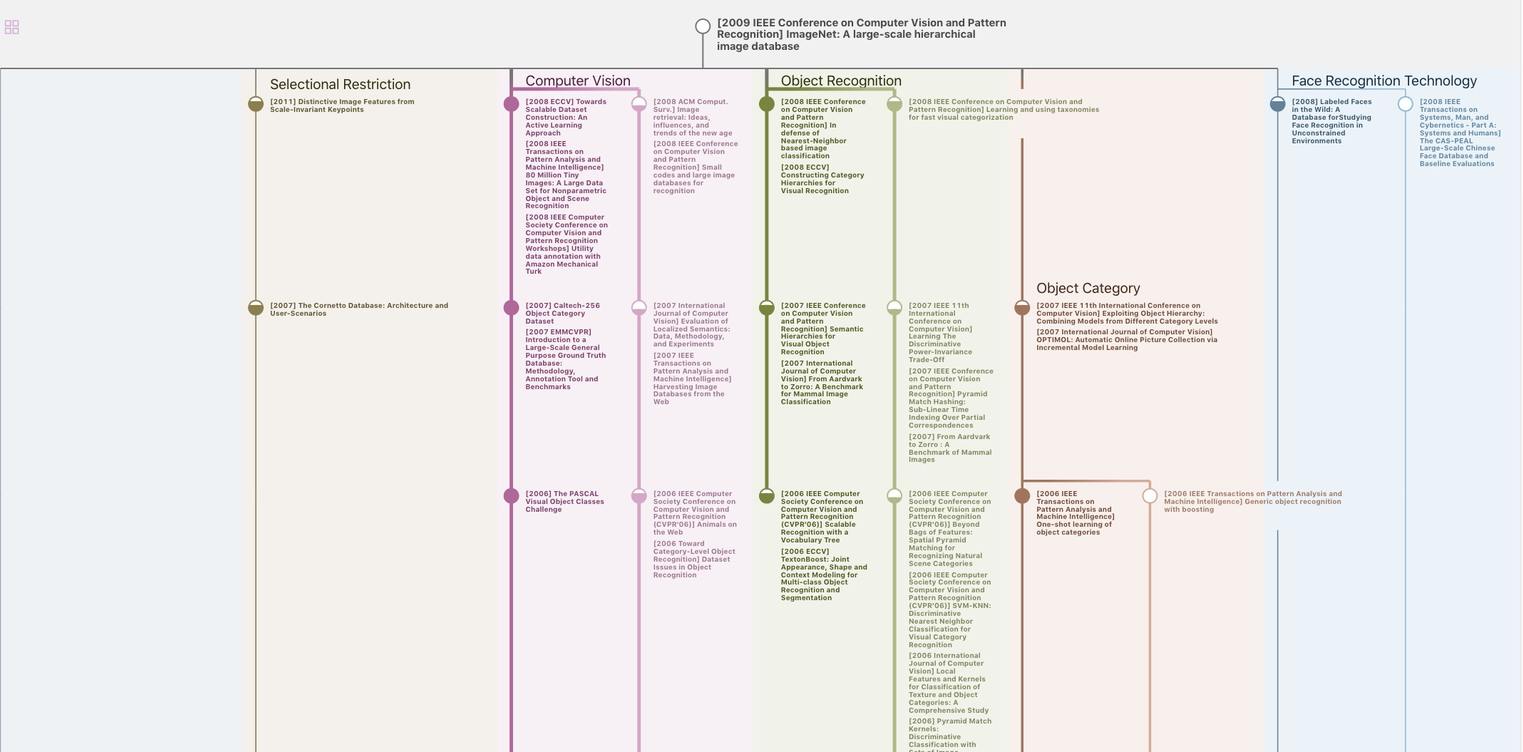
Generate MRT to find the research sequence of this paper
Chat Paper
Summary is being generated by the instructions you defined