A Standard Self-Learning Unsupervised Contrastive Learning Model for Bearing Fault Diagnosis in Imbalanced Datasets
2023 Global Reliability and Prognostics and Health Management Conference (PHM-Hangzhou)(2023)
摘要
Deep learning is widely employed in rolling bearing fault diagnosis due to its powerful feature extraction capability. However, deep learning heavily relies on balanced datasets, and the number of samples for fault categories in actual detected data is much smaller than that of normal categories, which significantly reduces the performance of deep learning models for fault diagnosis. To address this issue, this article proposes an unsupervised contrastive learning phase method based on standard self‑learning data augmentation (SSDA-USCL). Firstly, positive and negative sample pairs are constructed through SSDA. Secondly, feature extraction of sample pairs is achieved through unsupervised comparative learning (USCL). Finally, cross-entropy with optimized weight is utilized to classify samples. Experiments were conducted on the CWRU dataset according to the proposed method in this article and results showed that it can learn distinct discriminative features in imbalanced datasets with high diagnostic accuracy.
更多查看译文
关键词
imbalanced dataset,intelligent fault diagnosis,unsupervised contrastive learning,standard self-learning data augmentation
AI 理解论文
溯源树
样例
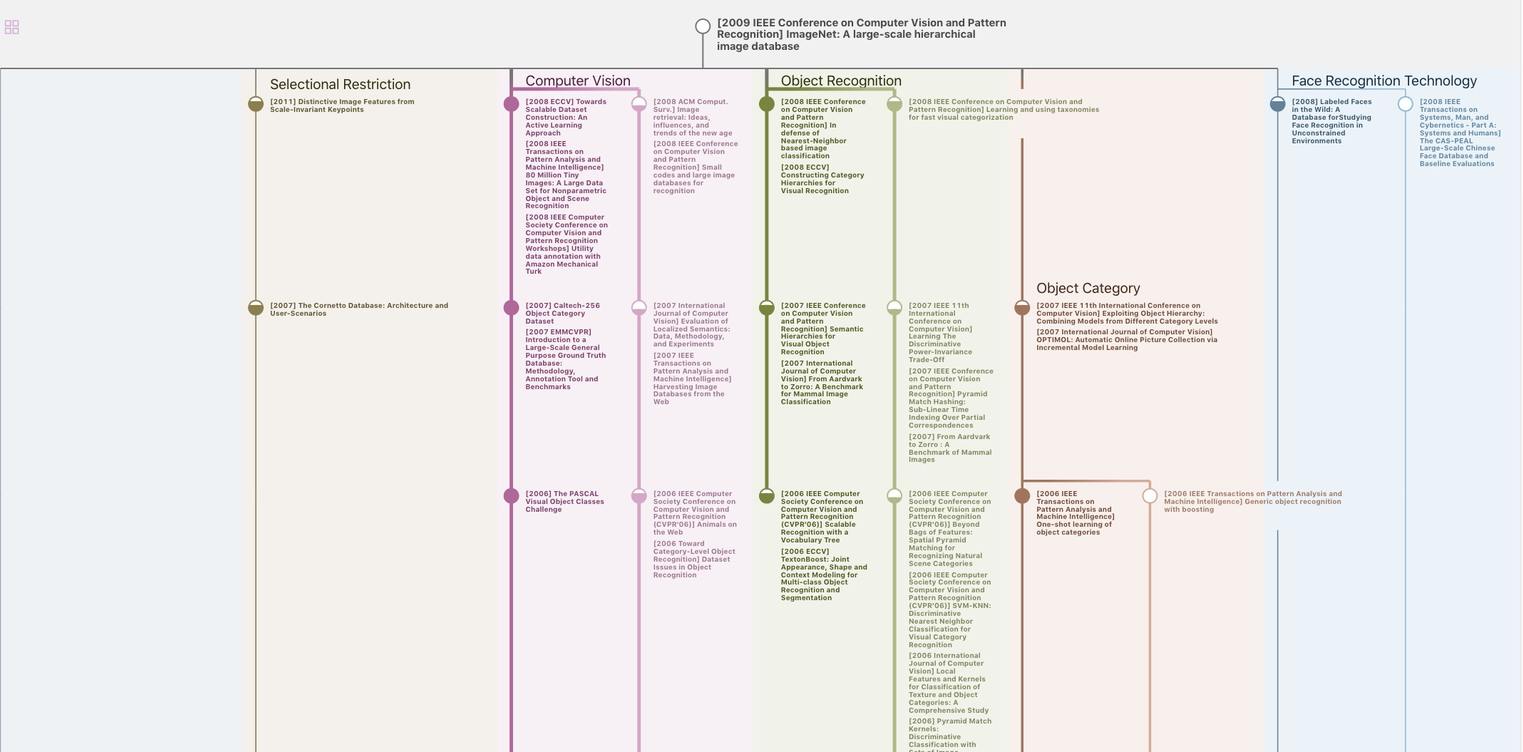
生成溯源树,研究论文发展脉络
Chat Paper
正在生成论文摘要