A machine-learning-enabled approach for bridging multiscale simulations of CNTs/PDMS composites
National Science Open(2024)
Abstract
Benefitting from the interlaced networking structure of carbon nanotubes (CNTs), the composites of CNTs/polydimethylsiloxane (PDMS) have found extensive applications in wearable electronics. While hierarchical multiscale simulation frameworks exist to optimize the structure parameters, their wide applications were hindered by the high computational cost. In this study, a machine learning model based on the artificial neural networks (ANN) embedded graph attention network, termed as AGAT, was proposed. The datasets collected from the micro-scale and the macro-scale simulations are utilized to train the model. The ANN layer within the model framework is trained to pass the information from micro-scale to macro-scale, while the whole model is aimed to predict the electro-mechanical behavior of the CNTs/PDMS composites. By comparing the AGAT model with the original multiscale simulation results, the data-driven strategy is shown to be promising with high accuracy, demonstrating the potential of the machine-learning-enabled approach for the structure optimization of CNT-based composites.
MoreTranslated text
Key words
multiscale simulation,machine learning,material property prediction,CNTs/PDMS composites
AI Read Science
Must-Reading Tree
Example
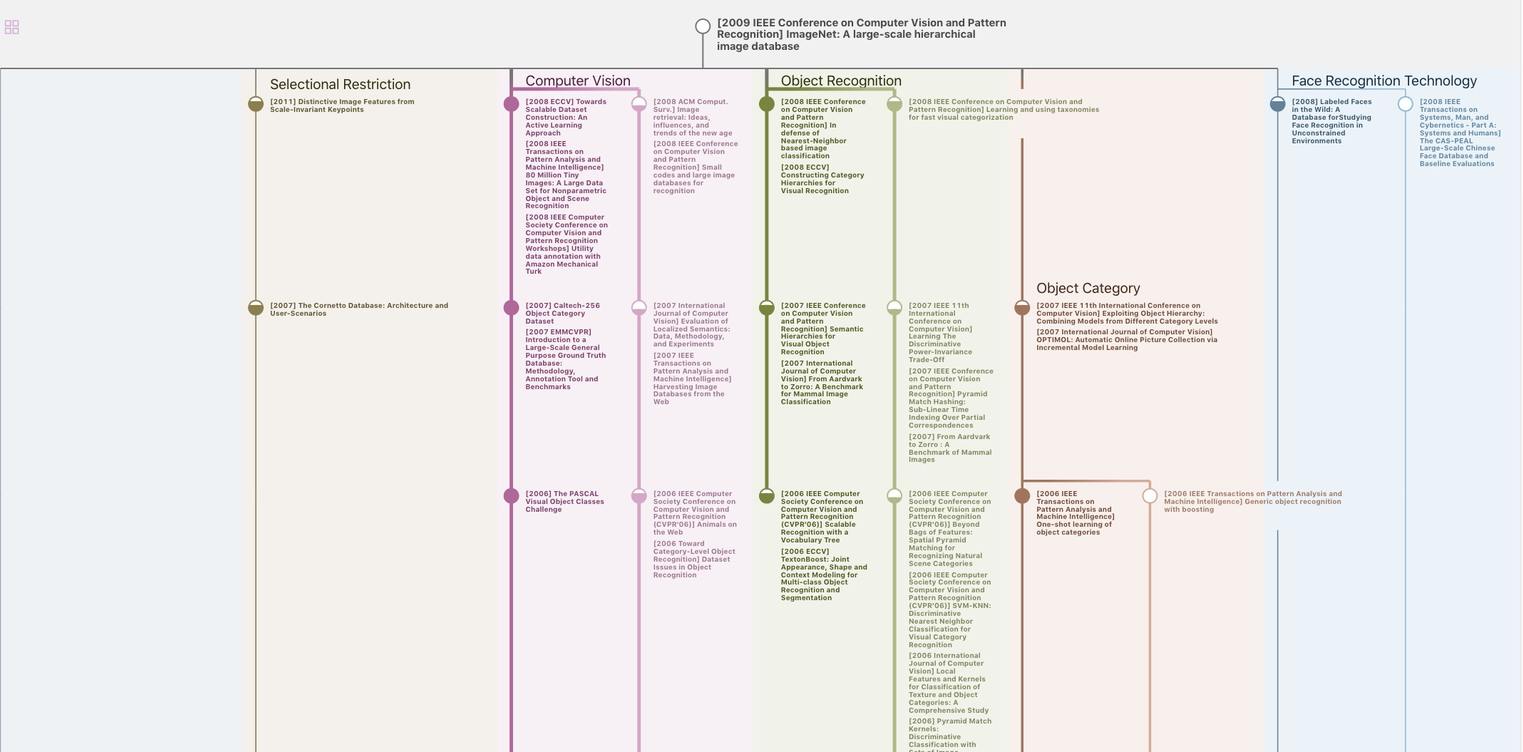
Generate MRT to find the research sequence of this paper
Chat Paper
Summary is being generated by the instructions you defined