ML-based inter-slice load balancing control for proactive offloading of virtual services
COMPUTER NETWORKS(2024)
摘要
In the new 5G networking digital era, Network Slicing is pivotal in delivering new visionary applications (e.g., holographic calls, tactical Internet, immersive video, and expanded reality) as well as driving new business models and market opportunities. To pave the way for delivering new applications, the Quality of Network -Slice Service (QoNSS) must be maintained over time, which requires intelligent decision-making logic at the network edge for agility. In this paper, we propose a new Network Slice control -plane function that lies on predictive analysis using Machine Learning (ML) over throughput and packet loss rate Key Quality Indicators (KQIs) at slice-delivered service application data traffic granularity to guarantee QoNSS over time. The study brings the following significant contributions to the field of dynamic network traffic management within a network slicing framework: ( i ) assessing the performance of three popular machine learning models to predict QoNSS-aware data traffic patterns at slice-delivered applications granularity; ( ii ) designing an intelligent decision-making function capable of proactively fostering inter -slice offloading of slice-delivered applications flows in response to QoNSS degradation predictions; and, ( iii ) providing testing outcomes to offer a better understanding of the different mapping features displayed by each machine learning model. The experiments were conducted atop different testbed settings featuring real-world slice-defined network and computing infrastructure. Detailed mappings and statistical outcome analyses suggest that the proactive load-balancing function outperformed a regular rule-based approach by significantly reducing the total packet loss of the network system while allowing high accuracy and anticipation reaction time.
更多查看译文
关键词
Network slicing,Load balancing,Service quality control,Machine learning,QoS
AI 理解论文
溯源树
样例
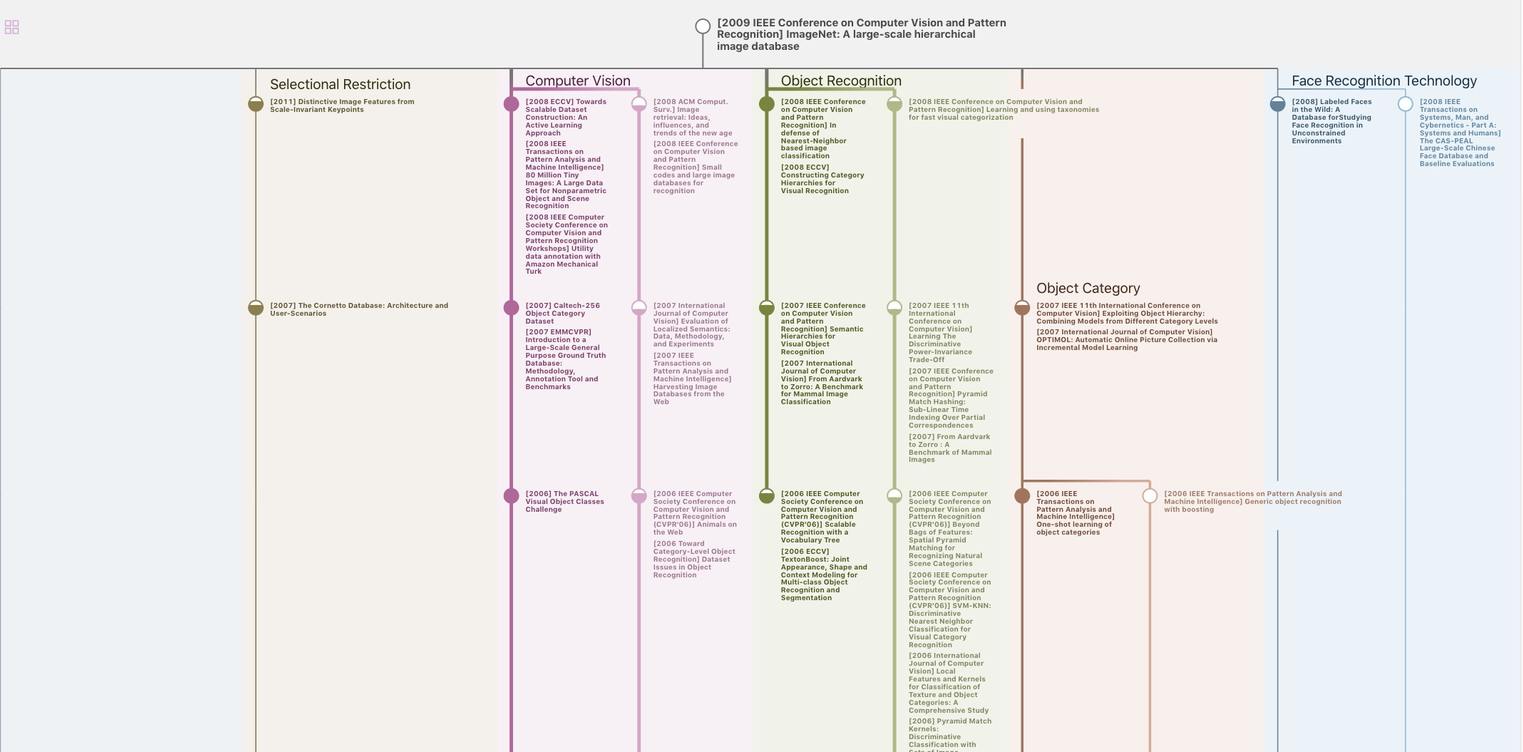
生成溯源树,研究论文发展脉络
Chat Paper
正在生成论文摘要