A machine learning algorithm based on circulating metabolic biomarkers offers improved predictions of neurological diseases
CLINICA CHIMICA ACTA(2024)
Abstract
Background and aims: A machine learning algorithm based on circulating metabolic biomarkers for the predictions of neurological diseases (NLDs) is lacking. To develop a machine learning algorithm to compare the performance of a metabolic biomarker-based model with that of a clinical model based on conventional risk factors for predicting three NLDs: dementia, Parkinson's disease (PD), and Alzheimer's disease (AD). Materials and methods: The eXtreme Gradient Boosting (XGBoost) algorithm was used to construct a metabolic biomarker-based model (metabolic model), a clinical risk factor -based model (clinical model), and a combined model for the prediction of the three NLDs. Risk discrimination (c -statistic), net reclassification improvement (NRI) index, and integrated discrimination improvement (IDI) index values were determined for each model. Results: The results indicate that incorporation of metabolic biomarkers into the clinical model afforded a model with improved performance in the prediction of dementia, AD, and PD, as demonstrated by NRI values of 0.159 (0.039-0.279), 0.113 (0.005-0.176), and 0.201 (- 0.021-0.423), respectively; and IDI values of 0.098 (0.073-0.122), 0.070 (0.049-0.090), and 0.085 (0.068-0.101), respectively. Conclusion: The performance of the model based on circulating NMR spectroscopy -detected metabolic biomarkers was better than that of the clinical model in the prediction of dementia, AD, and PD.
MoreTranslated text
Key words
Neurological diseases,Dementia,Machine learning,Risk prediction
AI Read Science
Must-Reading Tree
Example
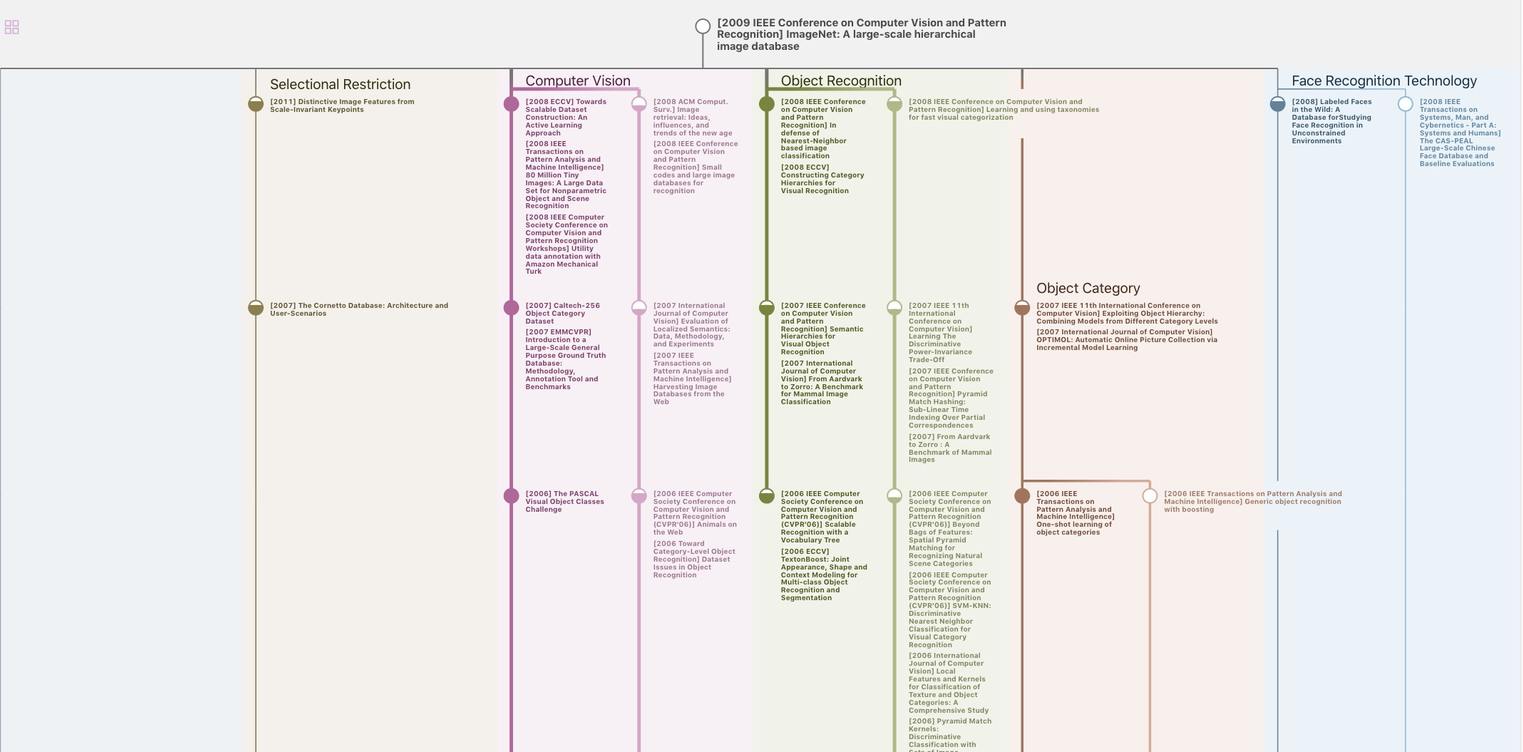
Generate MRT to find the research sequence of this paper
Chat Paper
Summary is being generated by the instructions you defined