An Information Quantity and Machine Learning Integrated Model for Landslide Susceptibility Mapping in Jiuzhaigou, China
Natural Hazards(2024)
Key words
Landslide susceptibility,Negative samples,Information quantity model,Machine learning,Jiuzhaigou
AI Read Science
Must-Reading Tree
Example
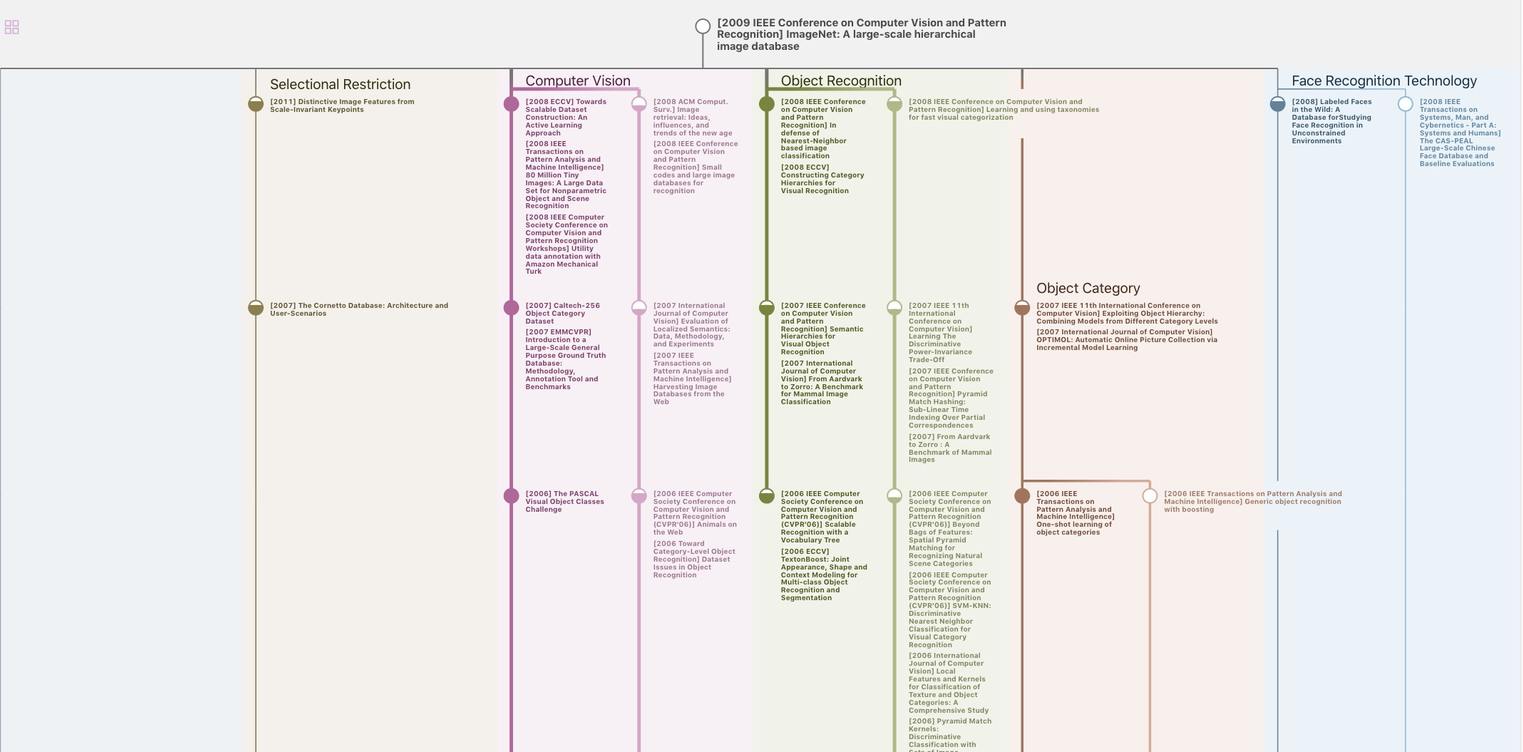
Generate MRT to find the research sequence of this paper
Chat Paper
Summary is being generated by the instructions you defined