An ensemble deep learning model for human activity analysis using wearable sensory data
APPLIED SOFT COMPUTING(2024)
摘要
Lately, the continuous temporal data from motion sensors in wearable devices has been great interest for the research community due to its demand for analyzing human activities in several applications e.g. healthcare, sports, and surveillance. Numerous solo deep learning models have been proposed in the literature to extract an adequate feature representation from temporal sensory data, however, they are limited to encode only one aspect of the data, and not sufficient to capture the in-depth association between the patterns. This paper presents an ensemble deep model to encode the complex patterns and dependencies in temporal data. An ensemble model refers to the merging or blending of separate deep models, aiming to utilize their unique strengths and abilities to construct a more resilient and effective solution. The contributions of this paper are two folds: First, a systematic evaluation of five different ensemble models is presented to encode the raw sensory data. Secondly, this study proposed a lightweight, multi -layer, and hybrid LSTM-GRU model to recognize the human activities using time series sensory data. Specifically, the raw sensory data is fed into a two -layer LSTM followed by GRU layers. To avoid over -fitting and accelerate convergence, we employed dropout and batch normalization layers. An extensive evaluation of all the five ensemble models is carried out on two large benchmark datasets, namely: UCI-HAR and WISDM. The proposed LSTM-GRU architecture achieved the recognition accuracy of 99.06% and 96.61% on the WISDM and UCI-HAR, respectively. The performance comparison with existing state-of-the-art techniques confirms the superiority and efficacy of the proposed algorithm.
更多查看译文
关键词
Ensemble deep model,Human activity recognition,Sensory data analysis,Time-series signal processing,Wearable devices
AI 理解论文
溯源树
样例
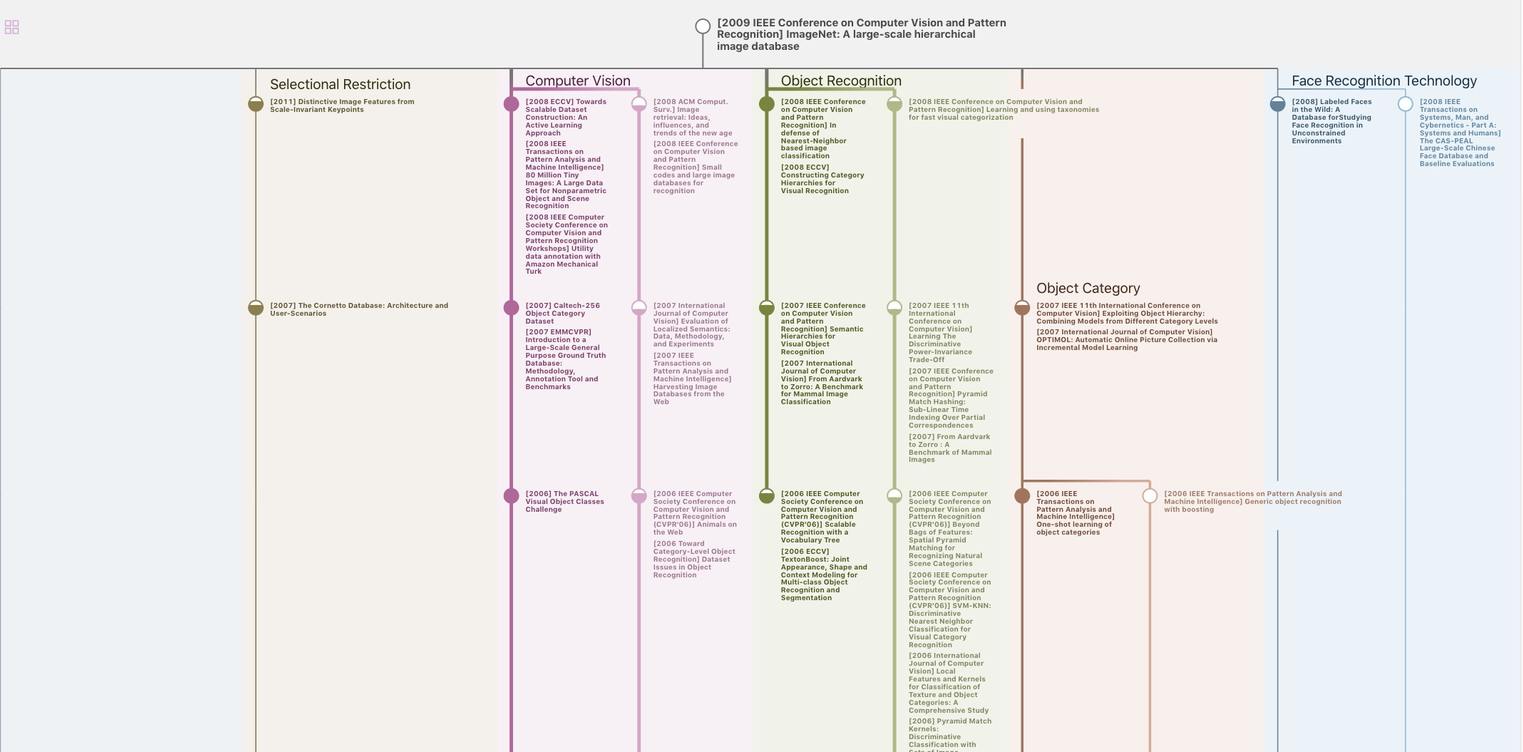
生成溯源树,研究论文发展脉络
Chat Paper
正在生成论文摘要