A Pretrained Significant Sample Generator for Partial Domain Transfer in Rotating Machinery Bearing Fault Diagnosis
2023 Global Reliability and Prognostics and Health Management Conference (PHM-Hangzhou)(2023)
摘要
In the context of bearing fault diagnosis, traditional supervised models often struggle due to a lack of sufficient information under novel operational conditions. Transfer learning is designed to address this issue by mitigating the domain shift between the source and target domains, thereby enhancing the utilization of the information available in the source domain. Furthermore, partial domain transfer learning addresses the problem of label imbalance, where the target domain has fewer labels than the source domain. Nevertheless, these methods generally presume the availability of a sufficiently large and balanced sample size, an assumption that often proves challenging in reality. For instance, when machinery faults occur, operations are usually terminated immediately. This results in the target domain being left with inadequate sample sizes, thereby inducing a sample imbalance between the two domains. To address the problem of sample imbalance in partial domain transfer scenarios, this paper proposes a pre-trained significance sample generator. This generator employs the backbone network to identify secure samples within the target domain, which are then used to augment the dataset of that domain. This process not only prevents mode collapse but also rectifies the sample imbalance. Experimental results validate the effectiveness of this generator in resolving the sample imbalance problem in the context of partial domain transfer bearing fault diagnosis.
更多查看译文
关键词
fault diagnosis,partial domain adaptation,rotating machinery,sample imbalance,transfer learning
AI 理解论文
溯源树
样例
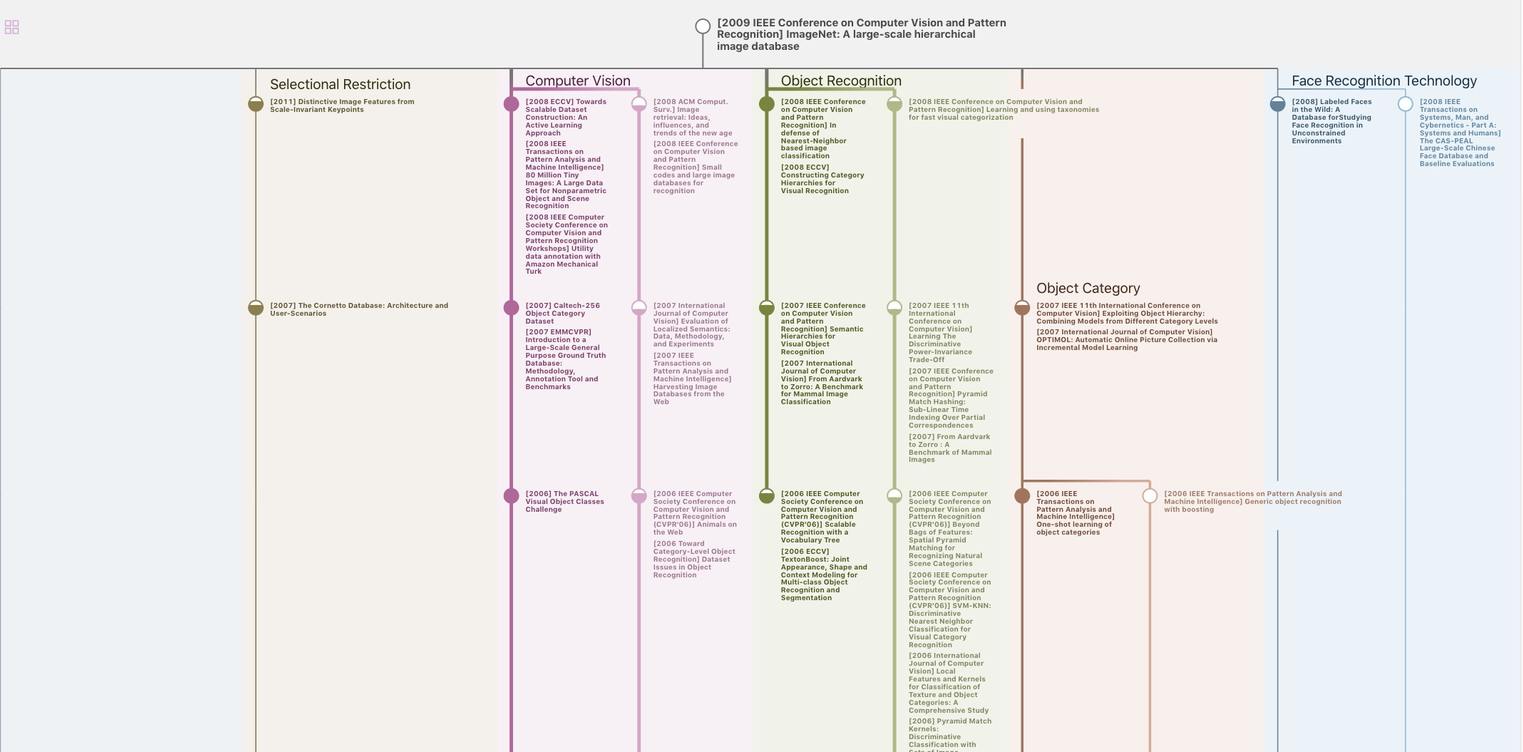
生成溯源树,研究论文发展脉络
Chat Paper
正在生成论文摘要