An Integrated Radiology-Pathology Machine Learning Classifier for Outcome Prediction Following Radical Prostatectomy: Preliminary Findings
Heliyon(2024)
Abstract
Objectives
To evaluate the added benefit of integrating features from pre-treatment MRI (radiomics) and digitized post-surgical pathology slides (pathomics) in prostate cancer (PCa) patients for prognosticating outcomes post radical-prostatectomy (RP) including a) rising prostate specific antigen (PSA), and b) extraprostatic-extension (EPE).
Methods
Multi-institutional data (N=58) of PCa patients who underwent pre-treatment 3-Tesla MRI prior to RP were included in this retrospective study. Radiomic and pathomic features were extracted from PCa regions on MRI and RP specimens delineated by expert clinicians. On training set (D1, N=44), Cox Proportional-Hazards models MR, MP and MRaP were trained using radiomics, pathomics, and their combination, respectively, to prognosticate rising PSA (PSA>0.03ng/mL). Top features from MRaP were used to train a model to predict EPE on D1 and test on external dataset (D2, N=14). C-index, Kalplan-Meier curves were used for survival analysis, and area under ROC(AUC) was used for EPE. MRaP was compared with the existing post-treatment risk-calculator, CAPRA(MC).
Results
Patients had median follow-up of 34 months. MRaP(c-index=0.685±0.05) significantly outperformed MR(c-index=0.646±0.05), MP(c-index=0.631±0.06) and MC(c-index=0.601±0.071)(p<0.0001). Cross-validated Kaplan-Meier curves showed significant separation among risk groups for rising PSA for MRaP (p<0.005, Hazard Ratio(HR)=11.36) as compared to MR(p=0.64, HR=1.33), MP (p=0.19, HR=2.82) and MC (p=0.10, HR=3.05). Integrated radio-pathomic model MRaP(AUC=0.80) outperformed MR(AUC=0.57) and MP (AUC=0.76) in predicting EPE on external-data (D2).
Conclusions
Results from this preliminary study suggest that a combination of radiomic and pathomic features can better predict post-surgical outcomes (rising PSA and EPE) compared to either of them individually as well as extant prognostic nomogram (CAPRA).
MoreTranslated text
Key words
Prostate cancer,MRI,Histopathology,Machine Learning,Prognosis
AI Read Science
Must-Reading Tree
Example
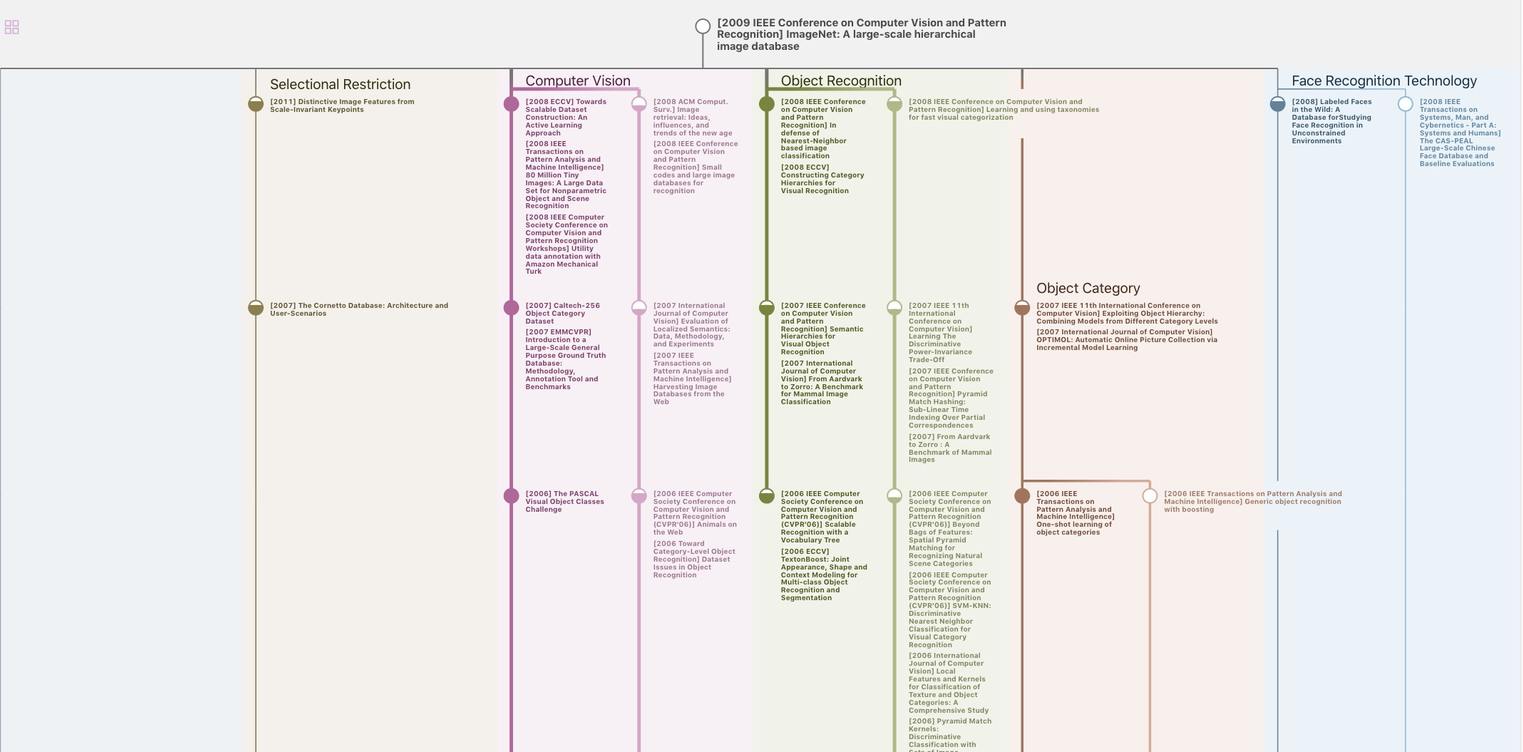
Generate MRT to find the research sequence of this paper
Chat Paper
Summary is being generated by the instructions you defined