MAP: Model Aggregation and Personalization in Federated Learning with Incomplete Classes
IEEE Transactions on Knowledge and Data Engineering(2024)
摘要
In some real-world applications, data samples are usually distributed on
local devices, where federated learning (FL) techniques are proposed to
coordinate decentralized clients without directly sharing users' private data.
FL commonly follows the parameter server architecture and contains multiple
personalization and aggregation procedures. The natural data heterogeneity
across clients, i.e., Non-I.I.D. data, challenges both the aggregation and
personalization goals in FL. In this paper, we focus on a special kind of
Non-I.I.D. scene where clients own incomplete classes, i.e., each client can
only access a partial set of the whole class set. The server aims to aggregate
a complete classification model that could generalize to all classes, while the
clients are inclined to improve the performance of distinguishing their
observed classes. For better model aggregation, we point out that the standard
softmax will encounter several problems caused by missing classes and propose
"restricted softmax" as an alternative. For better model personalization, we
point out that the hard-won personalized models are not well exploited and
propose "inherited private model" to store the personalization experience. Our
proposed algorithm named MAP could simultaneously achieve the aggregation and
personalization goals in FL. Abundant experimental studies verify the
superiorities of our algorithm.
更多查看译文
关键词
Federated learning,non-I.I.D. data,label distribution shift,aggregation,personalization,incomplete classes,restricted softmax,inherited private model
AI 理解论文
溯源树
样例
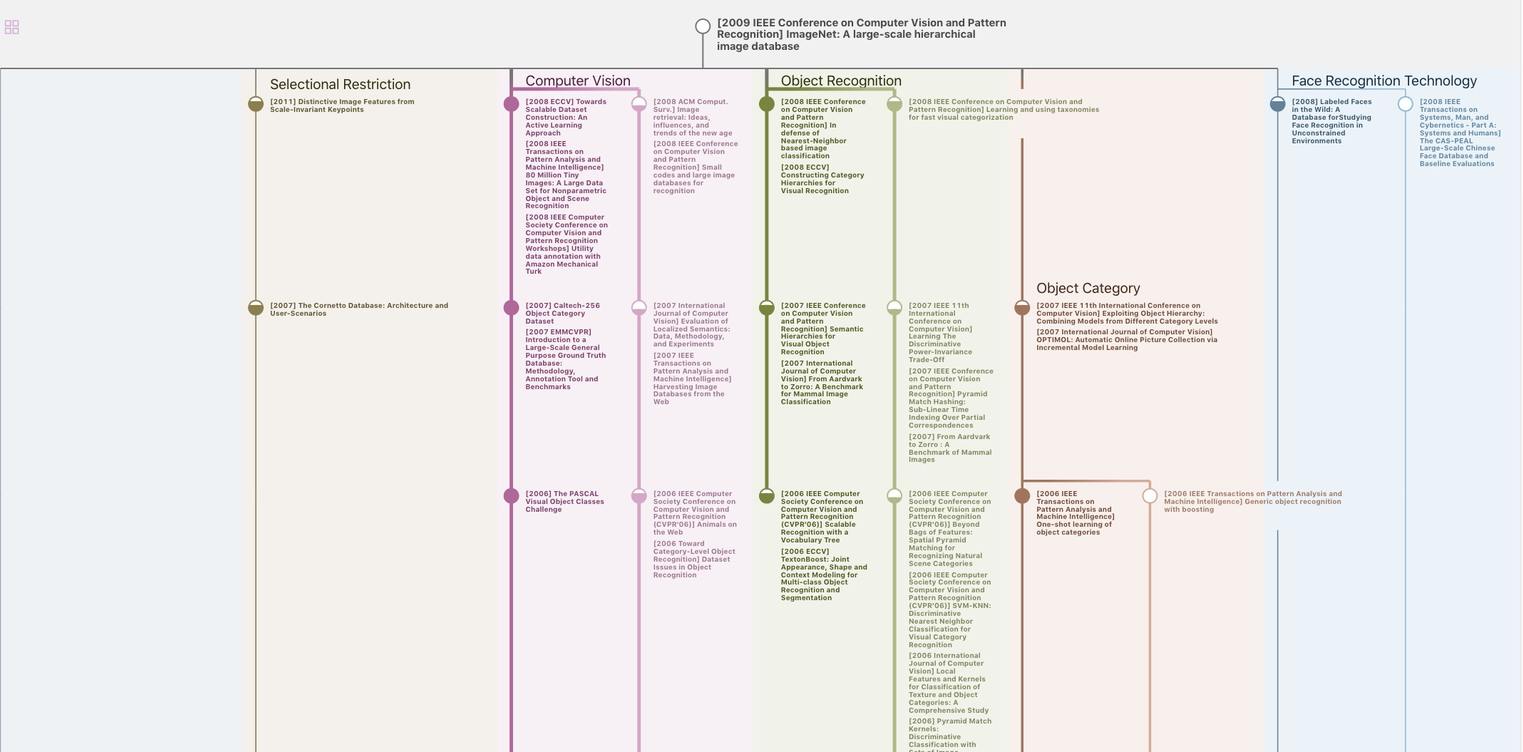
生成溯源树,研究论文发展脉络
Chat Paper
正在生成论文摘要