Extending Mean-Field Variational Inference via Entropic Regularization: Theory and Computation
CoRR(2024)
摘要
Variational inference (VI) has emerged as a popular method for approximate
inference for high-dimensional Bayesian models. In this paper, we propose a
novel VI method that extends the naive mean field via entropic regularization,
referred to as Ξ-variational inference (Ξ-VI). Ξ-VI has a close
connection to the entropic optimal transport problem and benefits from the
computationally efficient Sinkhorn algorithm. We show that Ξ-variational
posteriors effectively recover the true posterior dependency, where the
dependence is downweighted by the regularization parameter. We analyze the role
of dimensionality of the parameter space on the accuracy of Ξ-variational
approximation and how it affects computational considerations, providing a
rough characterization of the statistical-computational trade-off in Ξ-VI.
We also investigate the frequentist properties of Ξ-VI and establish
results on consistency, asymptotic normality, high-dimensional asymptotics, and
algorithmic stability. We provide sufficient criteria for achieving
polynomial-time approximate inference using the method. Finally, we demonstrate
the practical advantage of Ξ-VI over mean-field variational inference on
simulated and real data.
更多查看译文
AI 理解论文
溯源树
样例
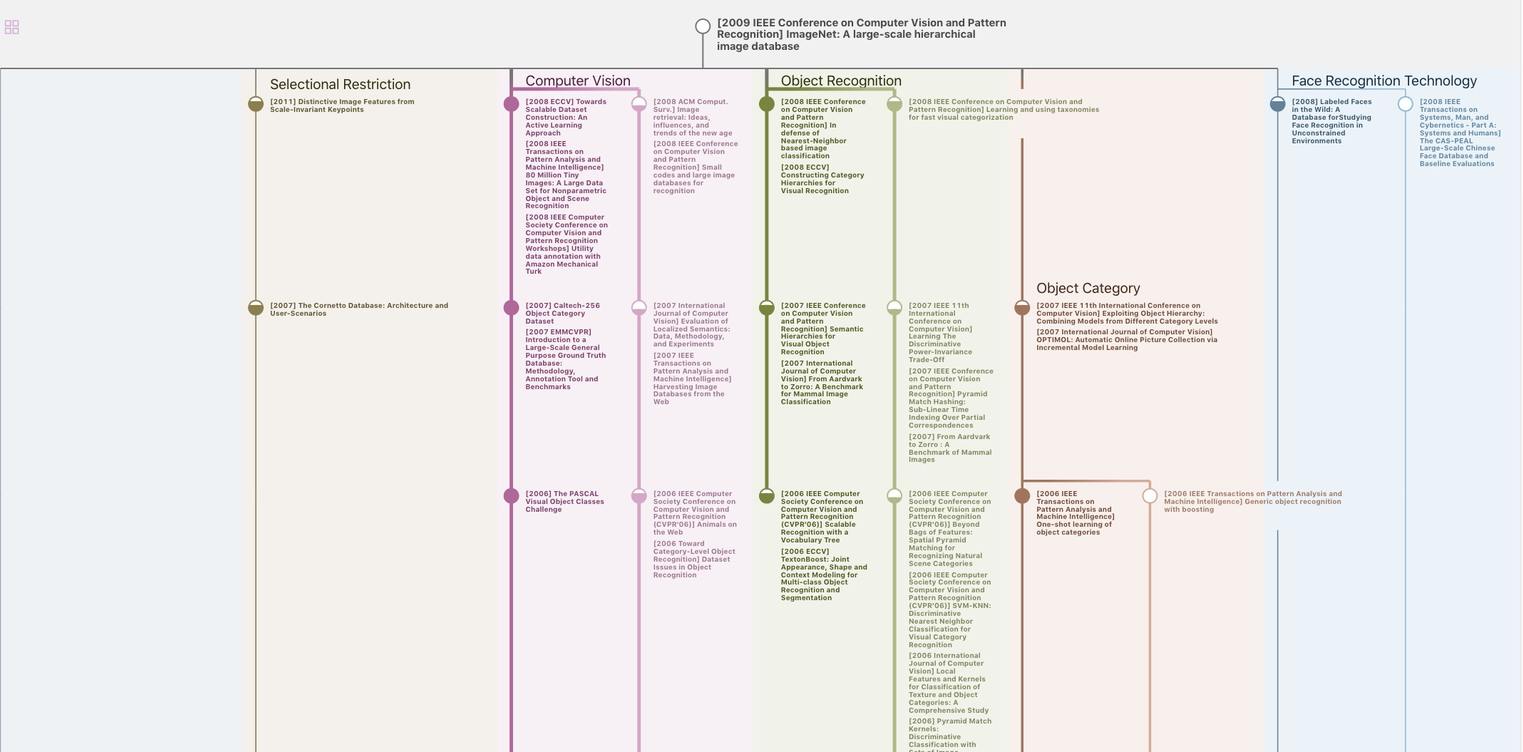
生成溯源树,研究论文发展脉络
Chat Paper
正在生成论文摘要